书目名称 | Generative Adversarial Learning: Architectures and Applications |
编辑 | Roozbeh Razavi-Far,Ariel Ruiz-Garcia,Juergen Schmi |
视频video | |
概述 | Presents high-quality research articles addressing theoretical work for improving the learning process.Provides a gentle introduction to GANs and related domains.Describes most well-known GAN architec |
丛书名称 | Intelligent Systems Reference Library |
图书封面 | 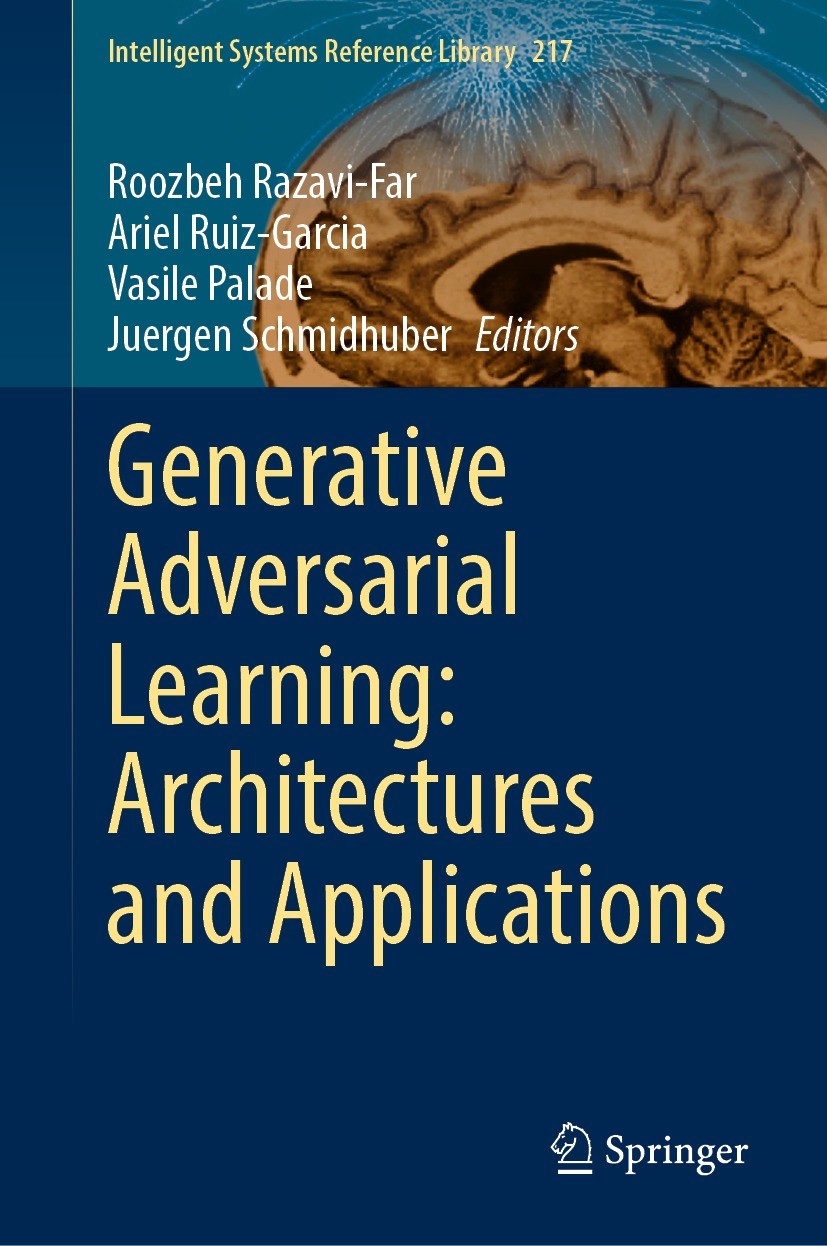 |
描述 | .This book provides a collection of recent research works addressing theoretical issues on improving the learning process and the generalization of GANs as well as state-of-the-art applications of GANs to various domains of real life. Adversarial learning fascinates the attention of machine learning communities across the world in recent years. Generative adversarial networks (GANs), as the main method of adversarial learning, achieve great success and popularity by exploiting a minimax learning concept, in which two networks compete with each other during the learning process. Their key capability is to generate new data and replicate available data distributions, which are needed in many practical applications, particularly in computer vision and signal processing. The book is intended for academics, practitioners, and research students in artificial intelligence looking to stay up to date with the latest advancements on GANs’ theoretical developments and their applications.. |
出版日期 | Book 2022 |
关键词 | Generative Adversarial Networks; Deep Learning; Artificial Intelligence; Neural Networks; Machine Learni |
版次 | 1 |
doi | https://doi.org/10.1007/978-3-030-91390-8 |
isbn_softcover | 978-3-030-91392-2 |
isbn_ebook | 978-3-030-91390-8Series ISSN 1868-4394 Series E-ISSN 1868-4408 |
issn_series | 1868-4394 |
copyright | The Editor(s) (if applicable) and The Author(s), under exclusive license to Springer Nature Switzerl |