书目名称 | Generalized Principal Component Analysis | 编辑 | René Vidal,Yi Ma,S.S. Sastry | 视频video | | 概述 | Introduces fundamental statistical, geometric and algebraic concepts.Encompasses relevant data clustering and modeling methods in machine learning.Addresses a general class of unsupervised learning pr | 丛书名称 | Interdisciplinary Applied Mathematics | 图书封面 | 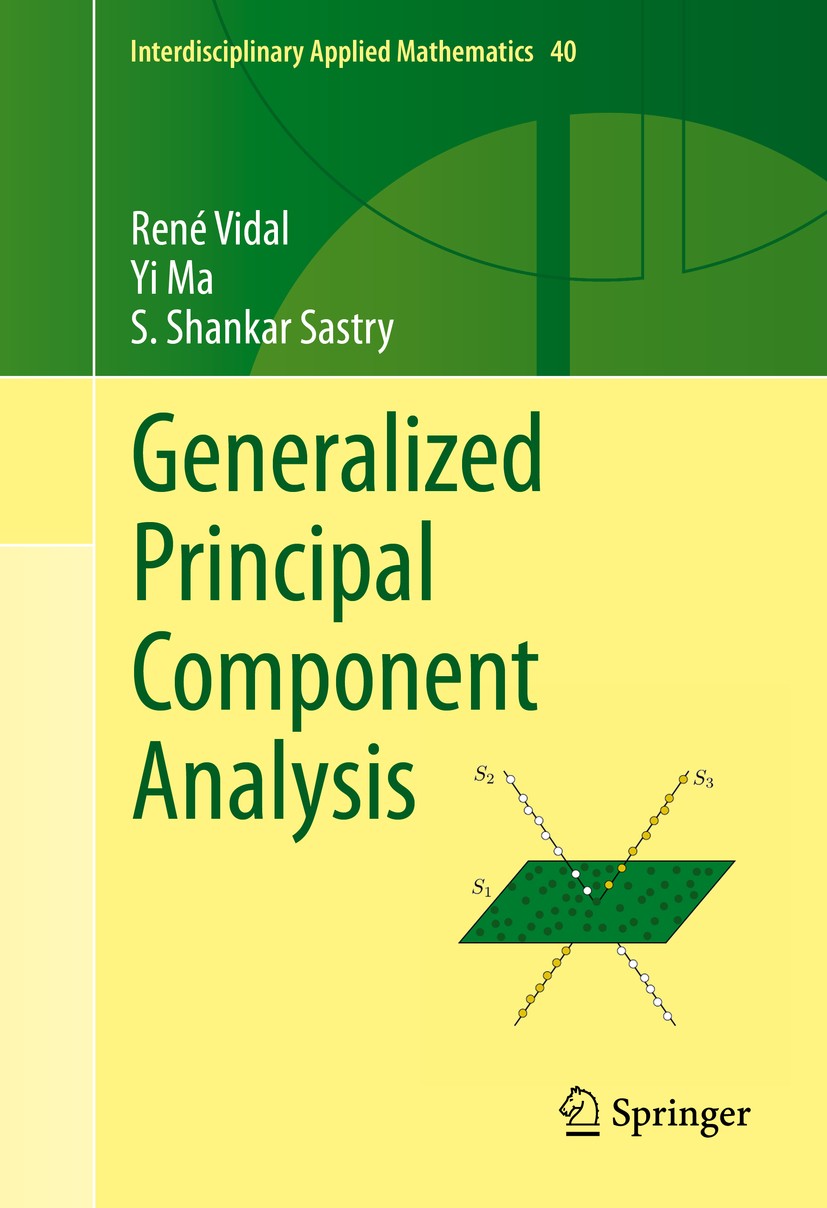 | 描述 | .This book provides a comprehensive introduction to the latest advances in the mathematical theory and computational tools for modeling high-dimensional data drawn from one or multiple low-dimensional subspaces (or manifolds) and potentially corrupted by noise, gross errors, or outliers. This challenging task requires the development of new algebraic, geometric, statistical, and computational methods for efficient and robust estimation and segmentation of one or multiple subspaces. The book also presents interesting real-world applications of these new methods in image processing, image and video segmentation, face recognition and clustering, and hybrid system identification etc. .This book is intended to serve as a textbook for graduate students and beginning researchers in data science, machine learning, computer vision, image and signal processing, and systems theory. It contains ample illustrations, examples, and exercises and is made largely self-contained with three Appendices which survey basic concepts and principles from statistics, optimization, and algebraic-geometry used in this book..René. Vidal. is a Professor of Biomedical Engineering and Director of the Vision Dynam | 出版日期 | Textbook 2016 | 关键词 | Principal component analysis; Robust principal component analysis; Manifold learning; Spectral clusteri | 版次 | 1 | doi | https://doi.org/10.1007/978-0-387-87811-9 | isbn_softcover | 978-1-4939-7912-7 | isbn_ebook | 978-0-387-87811-9Series ISSN 0939-6047 Series E-ISSN 2196-9973 | issn_series | 0939-6047 | copyright | Springer-Verlag New York 2016 |
The information of publication is updating
|
|