书目名称 | Explainable AI: Interpreting, Explaining and Visualizing Deep Learning | 编辑 | Wojciech Samek,Grégoire Montavon,Klaus-Robert Müll | 视频video | | 概述 | Assesses the current state of research on Explainable AI (XAI).Provides a snapshot of interpretable AI techniques.Reflects the current discourse and provides directions of future development | 丛书名称 | Lecture Notes in Computer Science | 图书封面 | 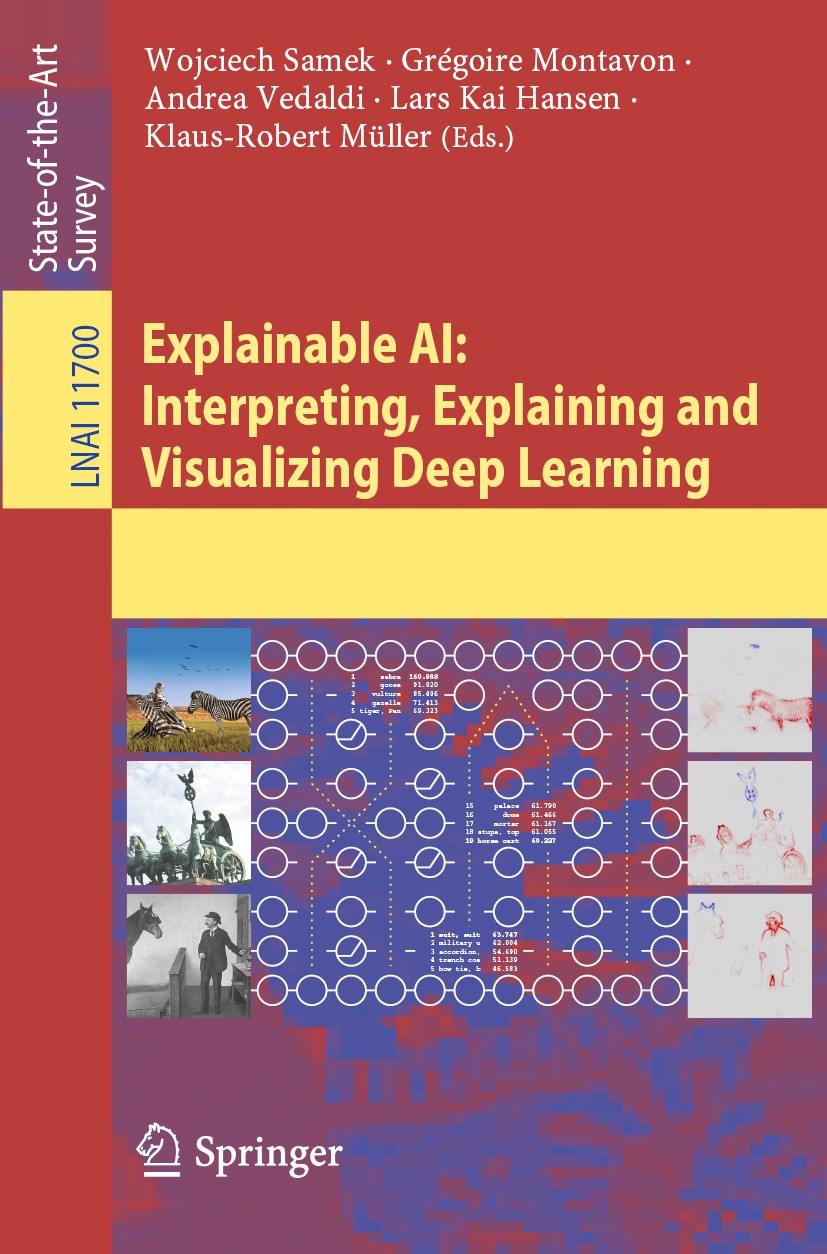 | 描述 | .The development of “intelligent” systems that can take decisions and perform autonomously might lead to faster and more consistent decisions. A limiting factor for a broader adoption of AI technology is the inherent risks that come with giving up human control and oversight to “intelligent” machines. For sensitive tasks involving critical infrastructures and affecting human well-being or health, it is crucial to limit the possibility of improper, non-robust and unsafe decisions and actions. Before deploying an AI system, we see a strong need to validate its behavior, and thus establish guarantees that it will continue to perform as expected when deployed in a real-world environment. In pursuit of that objective, ways for humans to verify the agreement between the AI decision structure and their own ground-truth knowledge have been explored. Explainable AI (XAI) has developed as a subfield of AI, focused on exposing complex AI models to humans in a systematic and interpretable manner...The 22 chapters included in this book provide a timely snapshot of algorithms, theory, and applications of interpretable and explainable AI and AI techniques that have been proposed recently reflecti | 出版日期 | Book 2019 | 关键词 | artificial intelligence; computer vision; deep Learning; explainable AI; explanation Methods; fuzzy contr | 版次 | 1 | doi | https://doi.org/10.1007/978-3-030-28954-6 | isbn_softcover | 978-3-030-28953-9 | isbn_ebook | 978-3-030-28954-6Series ISSN 0302-9743 Series E-ISSN 1611-3349 | issn_series | 0302-9743 | copyright | Springer Nature Switzerland AG 2019 |
The information of publication is updating
书目名称Explainable AI: Interpreting, Explaining and Visualizing Deep Learning影响因子(影响力) 
书目名称Explainable AI: Interpreting, Explaining and Visualizing Deep Learning影响因子(影响力)学科排名 
书目名称Explainable AI: Interpreting, Explaining and Visualizing Deep Learning网络公开度 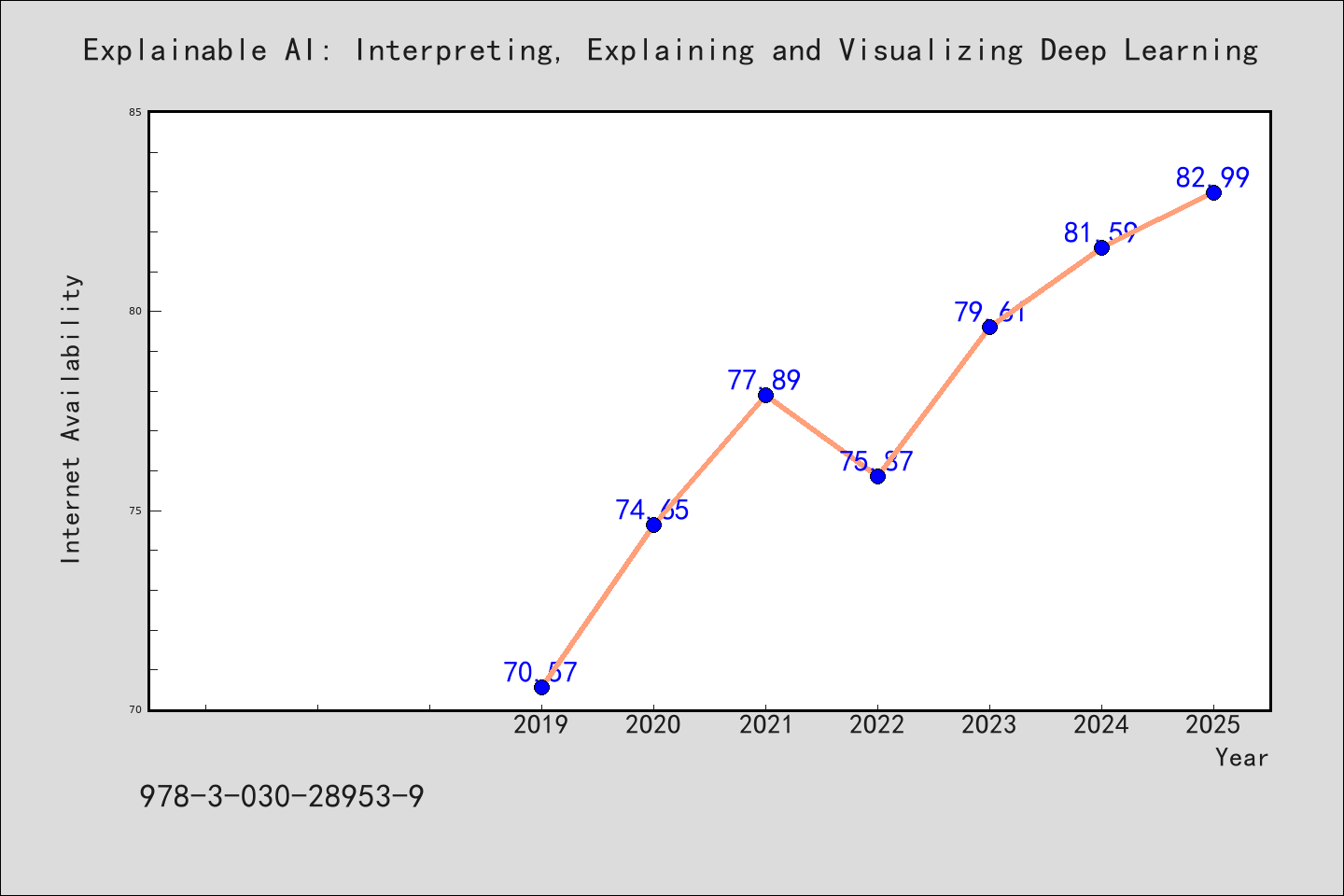
书目名称Explainable AI: Interpreting, Explaining and Visualizing Deep Learning网络公开度学科排名 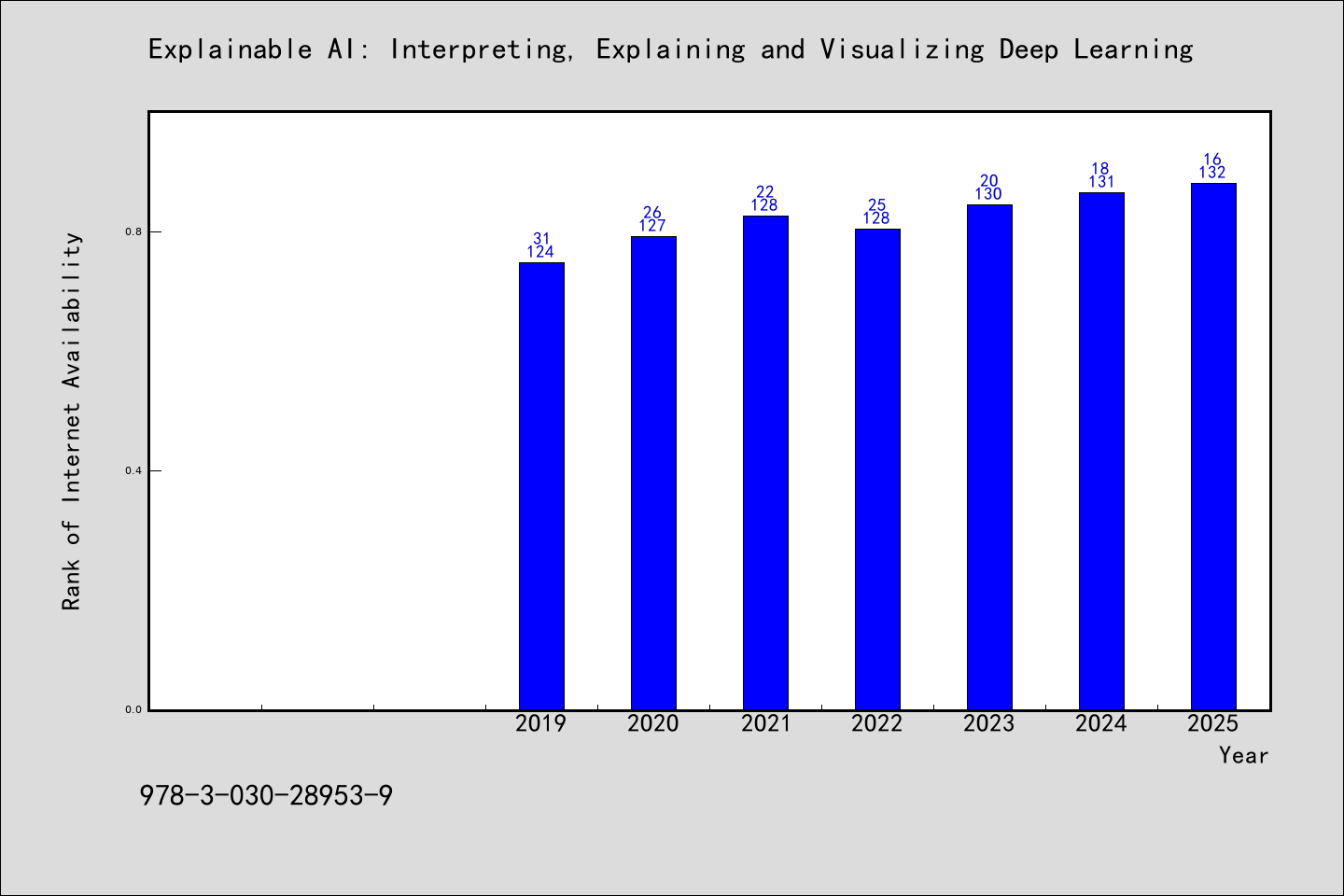
书目名称Explainable AI: Interpreting, Explaining and Visualizing Deep Learning被引频次 
书目名称Explainable AI: Interpreting, Explaining and Visualizing Deep Learning被引频次学科排名 
书目名称Explainable AI: Interpreting, Explaining and Visualizing Deep Learning年度引用 
书目名称Explainable AI: Interpreting, Explaining and Visualizing Deep Learning年度引用学科排名 
书目名称Explainable AI: Interpreting, Explaining and Visualizing Deep Learning读者反馈 
书目名称Explainable AI: Interpreting, Explaining and Visualizing Deep Learning读者反馈学科排名 
|
|
|