书目名称 | Explainable AI in Healthcare and Medicine | 副标题 | Building a Culture o | 编辑 | Arash Shaban-Nejad,Martin Michalowski,David L. Buc | 视频video | | 概述 | Highlights the latest advances in explainable AI in health care and medicine by presenting significant findings on theory, methods, systems, and applications.Includes revised versions of selected pape | 丛书名称 | Studies in Computational Intelligence | 图书封面 | 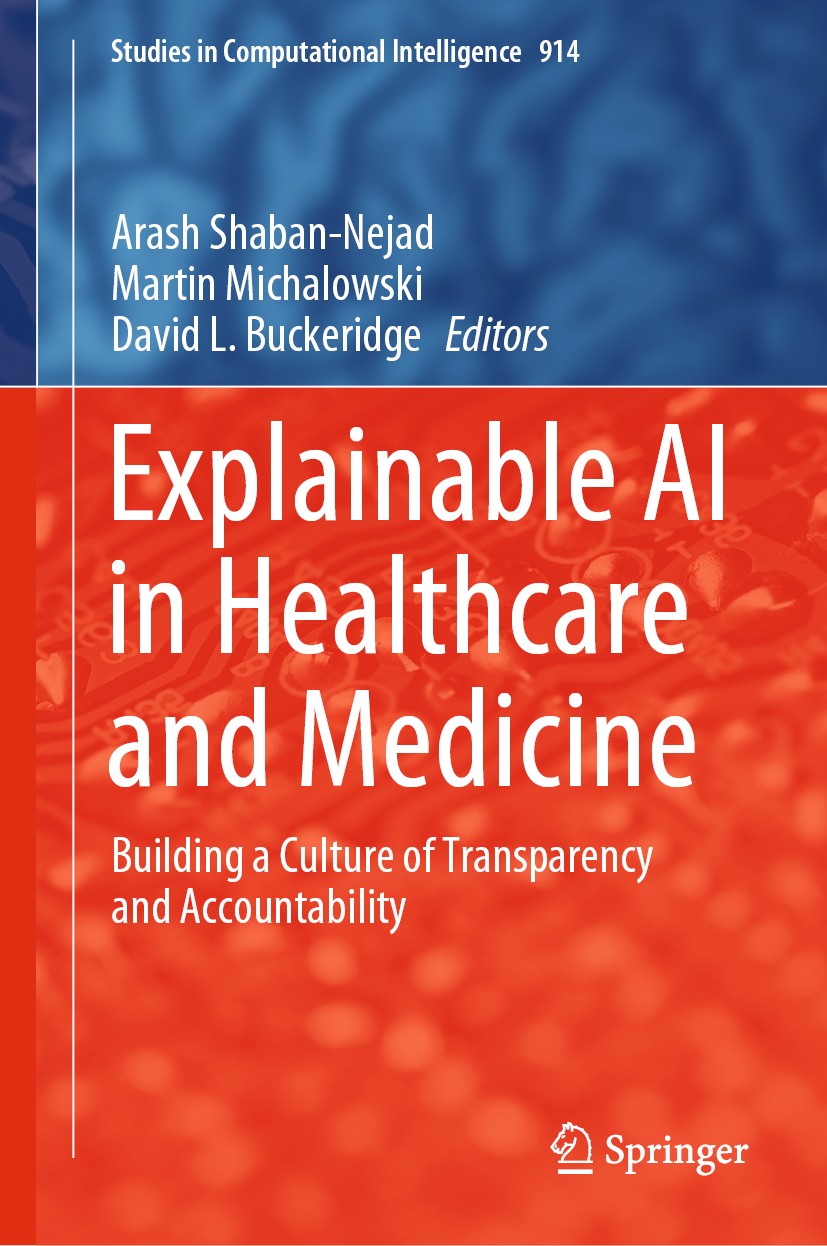 | 描述 | This book highlights the latest advances in the application of artificial intelligence and data science in health care and medicine. Featuring selected papers from the 2020 Health Intelligence Workshop, held as part of the Association for the Advancement of Artificial Intelligence (AAAI) Annual Conference, it offers an overview of the issues, challenges, and opportunities in the field, along with the latest research findings. Discussing a wide range of practical applications, it makes the emerging topics of digital health and explainable AI in health care and medicine accessible to a broad readership. The availability of explainable and interpretable models is a first step toward building a culture of transparency and accountability in health care. As such, this book provides information for scientists, researchers, students, industry professionals, public health agencies, and NGOs interested in the theory and practice of computational models of public and personalized health intelligence. | 出版日期 | Book 2021 | 关键词 | Health Intelligence; Precession Medicine; Precession Health; Digital Medicine; Big Data; Predictive Analy | 版次 | 1 | doi | https://doi.org/10.1007/978-3-030-53352-6 | isbn_softcover | 978-3-030-53354-0 | isbn_ebook | 978-3-030-53352-6Series ISSN 1860-949X Series E-ISSN 1860-9503 | issn_series | 1860-949X | copyright | The Editor(s) (if applicable) and The Author(s), under exclusive license to Springer Nature Switzerl |
The information of publication is updating
|
|