书目名称 | Evolutionary Machine Learning Techniques | 副标题 | Algorithms and Appli | 编辑 | Seyedali Mirjalili,Hossam Faris,Ibrahim Aljarah | 视频video | | 概述 | Provides an in-depth analysis of the current evolutionary machine learning techniques.Includes training algorithms for machine learning techniques.Covers the application of improved artificial neural | 丛书名称 | Algorithms for Intelligent Systems | 图书封面 | 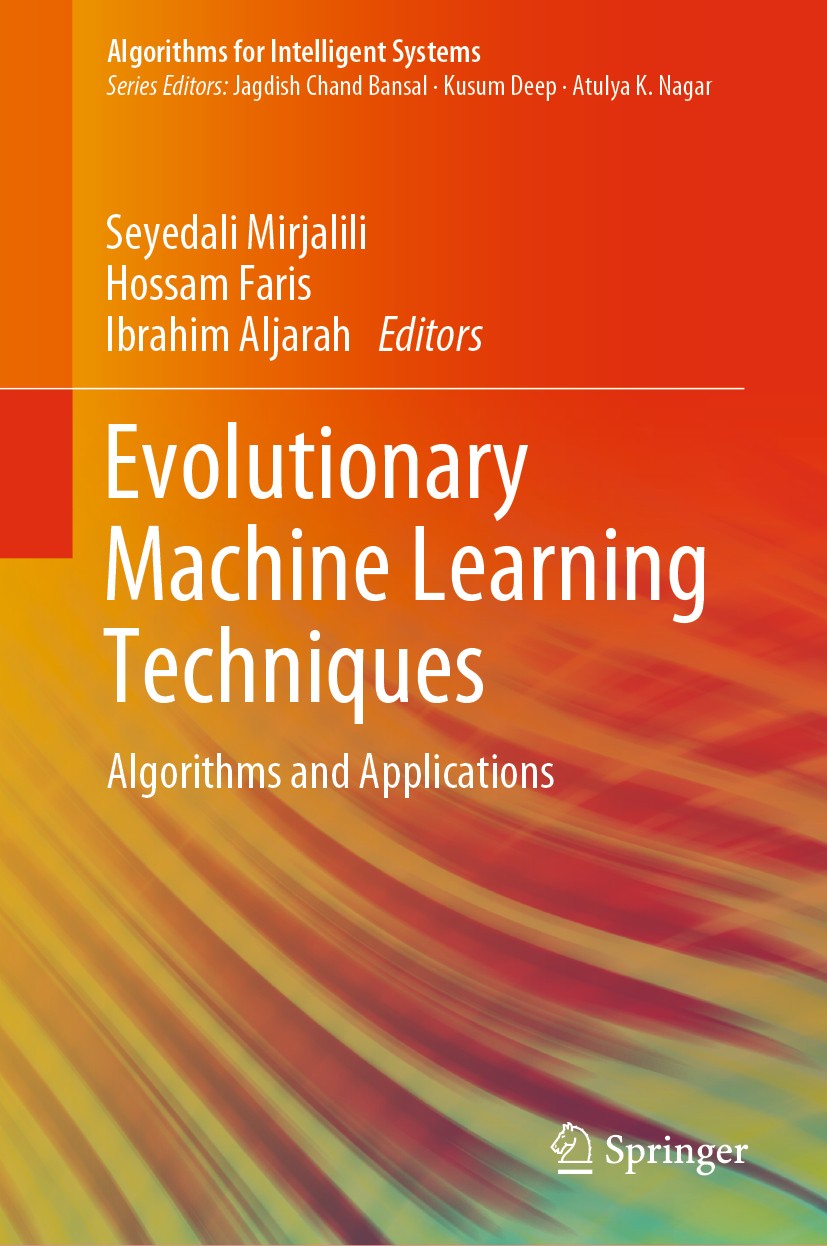 | 描述 | .This book provides an in-depth analysis of the current evolutionary machine learning techniques. Discussing the most highly regarded methods for classification, clustering, regression, and prediction, it includes techniques such as support vector machines, extreme learning machines, evolutionary feature selection, artificial neural networks including feed-forward neural networks, multi-layer perceptron, probabilistic neural networks, self-optimizing neural networks, radial basis function networks, recurrent neural networks, spiking neural networks, neuro-fuzzy networks, modular neural networks, physical neural networks, and deep neural networks.. ..The book provides essential definitions, literature reviews, and the training algorithms for machine learning using classical and modern nature-inspired techniques. It also investigates the pros and cons of classical training algorithms. It features a range of proven and recent nature-inspired algorithms used to train different types of artificial neural networks, including genetic algorithm, ant colony optimization, particle swarm optimization, grey wolf optimizer, whale optimization algorithm, ant lion optimizer, moth flame algorithm, | 出版日期 | Book 2020 | 关键词 | Artificial Neural Network; Probabilistic Neural Network; Self-Optimizing Neural Network; Feedforward Ne | 版次 | 1 | doi | https://doi.org/10.1007/978-981-32-9990-0 | isbn_softcover | 978-981-32-9992-4 | isbn_ebook | 978-981-32-9990-0Series ISSN 2524-7565 Series E-ISSN 2524-7573 | issn_series | 2524-7565 | copyright | Springer Nature Singapore Pte Ltd. 2020 |
The information of publication is updating
|
|