书目名称 | Evolutionary Approach to Machine Learning and Deep Neural Networks | 副标题 | Neuro-Evolution and | 编辑 | Hitoshi Iba | 视频video | | 概述 | Begins with the essentials of evolutionary algorithms and covers state-of-the-art research methodologies in the field as well as growing research trends.Presents concepts to promote and facilitate eff | 图书封面 | 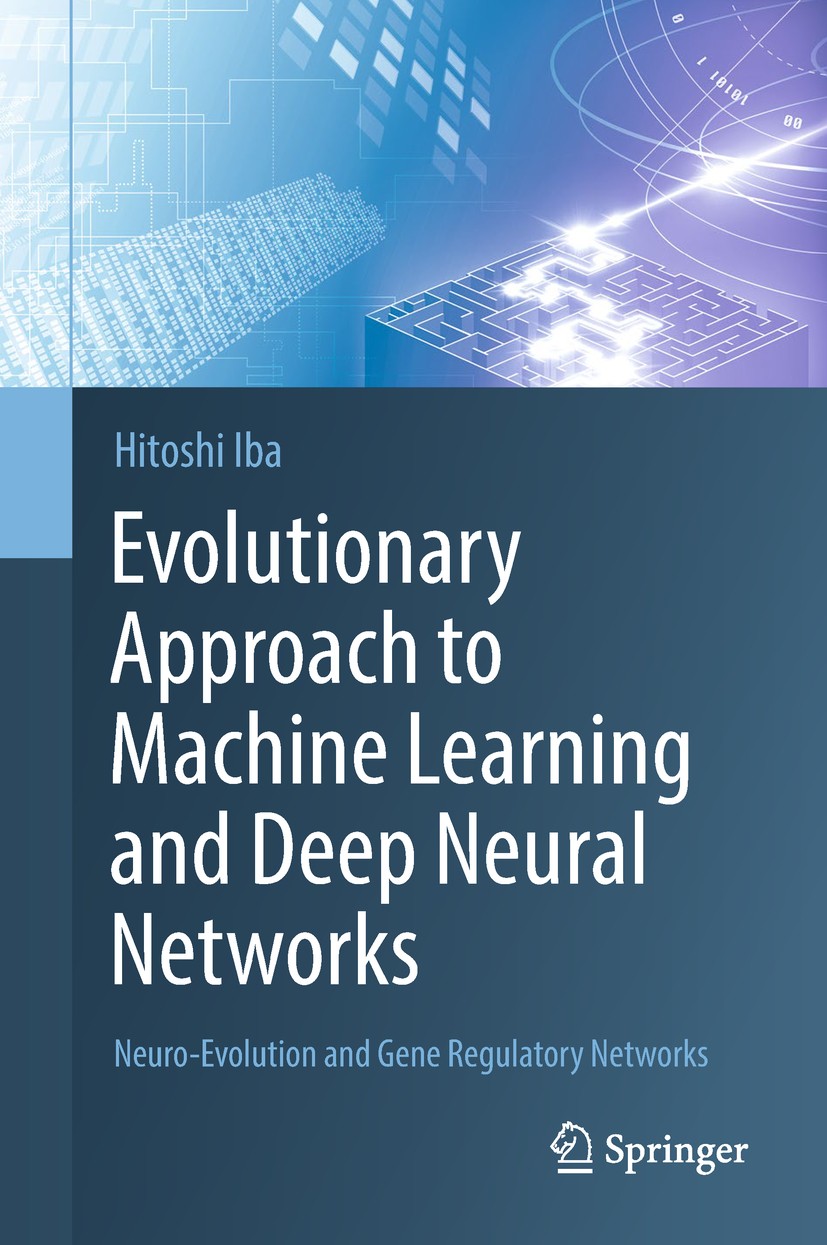 | 描述 | This book provides theoretical and practical knowledge about a methodology for evolutionary algorithm-based search strategy with the integration of several machine learning and deep learning techniques. These include convolutional neural networks, Gröbner bases, relevance vector machines, transfer learning, bagging and boosting methods, clustering techniques (affinity propagation), and belief networks, among others. The development of such tools contributes to better optimizing methodologies. Beginning with the essentials of evolutionary algorithms and covering interdisciplinary research topics, the contents of this book are valuable for different classes of readers: novice, intermediate, and also expert readers from related fields..Following the chapters on introduction and basic methods, Chapter 3 details a new research direction, i.e., neuro-evolution, an evolutionary method for the generation of deep neural networks, and also describes how evolutionary methods are extended in combination with machine learning techniques. Chapter 4 includes novel methods such as particle swarm optimization based on affinity propagation (PSOAP), and transfer learning for differential evolution (T | 出版日期 | Book 2018 | 关键词 | Evolutionary Computation; Evolutionary Computation; Meta-Heuristics; Deep Learning; Machine Learning; Gen | 版次 | 1 | doi | https://doi.org/10.1007/978-981-13-0200-8 | isbn_softcover | 978-981-13-4358-2 | isbn_ebook | 978-981-13-0200-8 | copyright | Springer Nature Singapore Pte Ltd. 2018 |
The information of publication is updating
|
|