书目名称 | Estimation and Testing Under Sparsity |
副标题 | École d‘Été de Proba |
编辑 | Sara van de Geer |
视频video | |
概述 | Starting with the popular Lasso method as its prime example, the book then extends to a broad family of estimation methods for high-dimensional data.A theoretical basis for sparsity-inducing methods i |
丛书名称 | Lecture Notes in Mathematics |
图书封面 | 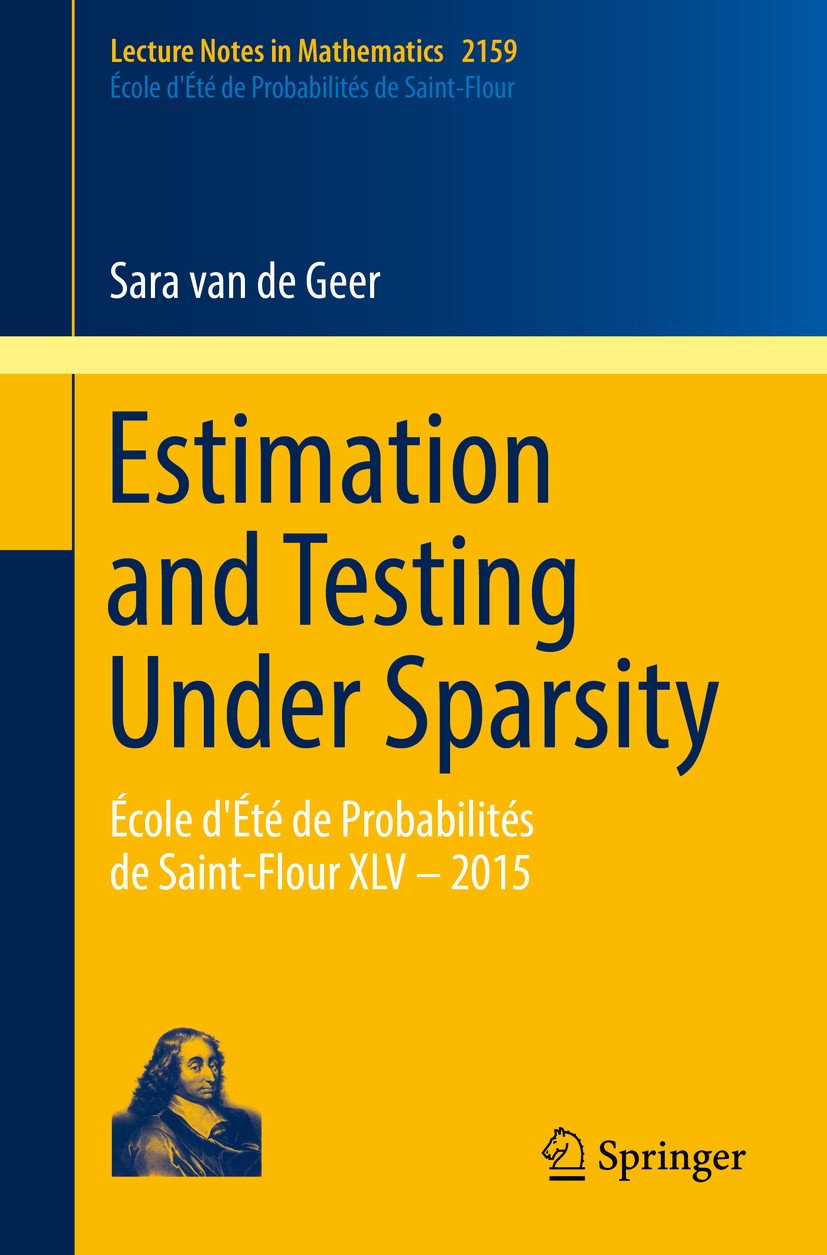 |
描述 | Taking the Lasso method as its starting point, this book describes the main ingredients needed to study general loss functions and sparsity-inducing regularizers. It also provides a semi-parametric approach to establishing confidence intervals and tests. Sparsity-inducing methods have proven to be very useful in the analysis of high-dimensional data. Examples include the Lasso and group Lasso methods, and the least squares method with other norm-penalties, such as the nuclear norm. The illustrations provided include generalized linear models, density estimation, matrix completion and sparse principal components. Each chapter ends with a problem section. The book can be used as a textbook for a graduate or PhD course. |
出版日期 | Book 2016 |
关键词 | 62-XX; 60-XX, 68Q87; high-dimensional statistics; sparsity; empirical risk minimization; oracle inequali |
版次 | 1 |
doi | https://doi.org/10.1007/978-3-319-32774-7 |
isbn_softcover | 978-3-319-32773-0 |
isbn_ebook | 978-3-319-32774-7Series ISSN 0075-8434 Series E-ISSN 1617-9692 |
issn_series | 0075-8434 |
copyright | Springer International Publishing Switzerland 2016 |