书目名称 | Embedded Machine Learning for Cyber-Physical, IoT, and Edge Computing | 副标题 | Use Cases and Emergi | 编辑 | Sudeep Pasricha,Muhammad Shafique | 视频video | | 概述 | Discusses efficient implementation of machine learning in embedded, CPS, IoT.Offers comprehensive coverage of hardware design, software design.Describes real applications to demonstrate how embedded, | 图书封面 | 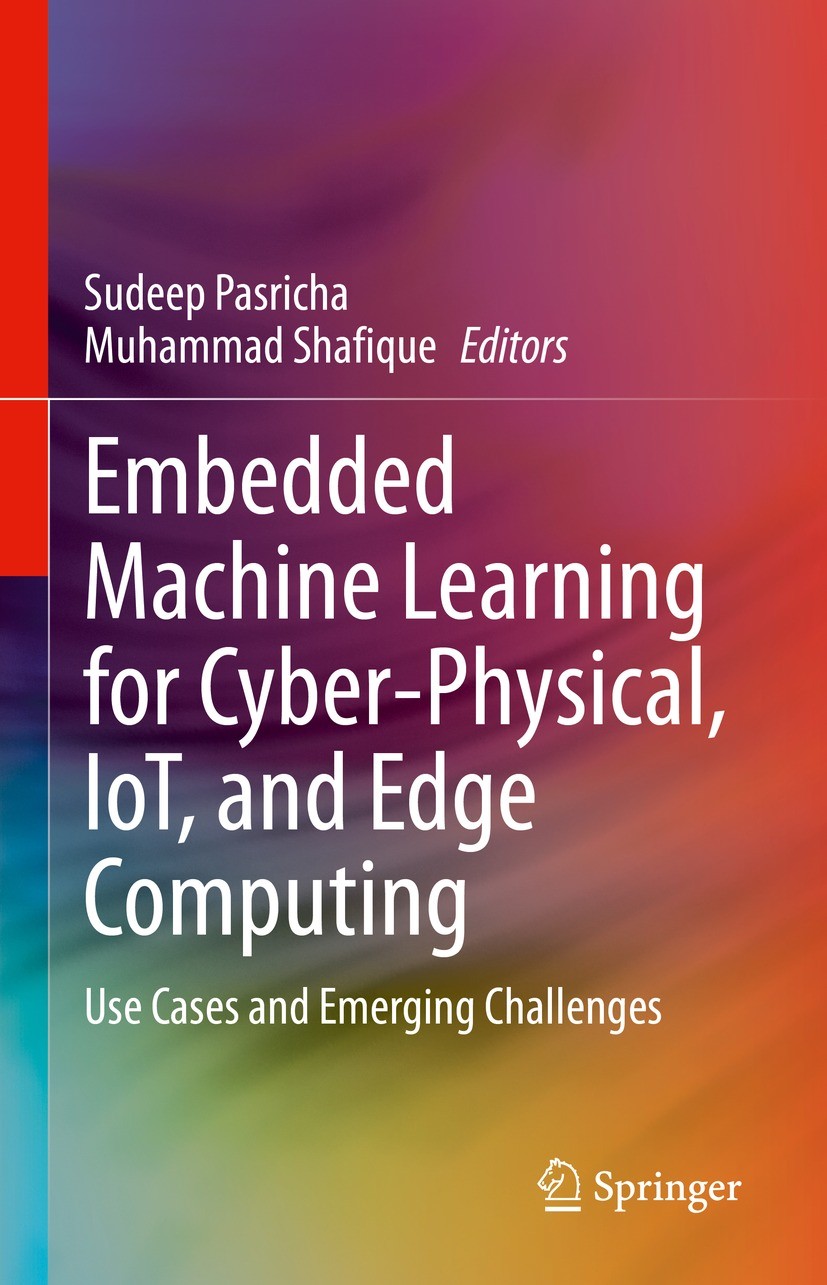 | 描述 | .This book presents recent advances towards the goal of enabling efficient implementation of machine learning models on resource-constrained systems, covering different application domains. The focus is on presenting interesting and new use cases of applying machine learning to innovative application domains, exploring the efficient hardware design of efficient machine learning accelerators, memory optimization techniques, illustrating model compression and neural architecture search techniques for energy-efficient and fast execution on resource-constrained hardware platforms, and understanding hardware-software codesign techniques for achieving even greater energy, reliability, and performance benefits..Discusses efficient implementation of machine learning in embedded, CPS, IoT, and edge computing; .Offers comprehensive coverage of hardware design, software design, and hardware/software co-design and co-optimization; .Describes real applications to demonstrate how embedded, CPS, IoT, and edge applications benefit from machine learning.. | 出版日期 | Book 2024 | 关键词 | Machine learning embedded systems; Machine learning IoT; Machine learning edge computing; Smart Cyber-P | 版次 | 1 | doi | https://doi.org/10.1007/978-3-031-40677-5 | isbn_softcover | 978-3-031-40679-9 | isbn_ebook | 978-3-031-40677-5 | copyright | The Editor(s) (if applicable) and The Author(s), under exclusive license to Springer Nature Switzerl |
The information of publication is updating
书目名称Embedded Machine Learning for Cyber-Physical, IoT, and Edge Computing影响因子(影响力) 
书目名称Embedded Machine Learning for Cyber-Physical, IoT, and Edge Computing影响因子(影响力)学科排名 
书目名称Embedded Machine Learning for Cyber-Physical, IoT, and Edge Computing网络公开度 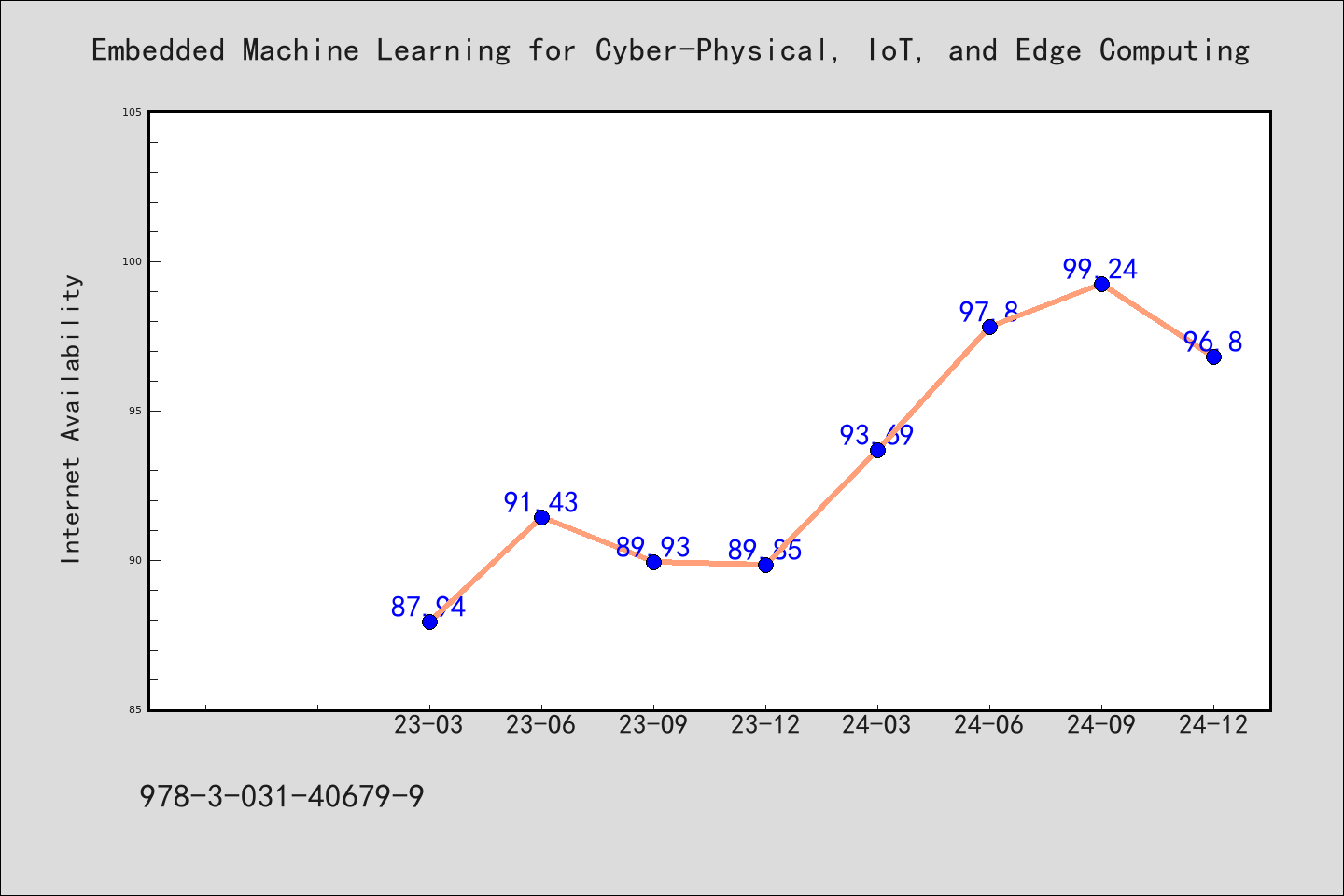
书目名称Embedded Machine Learning for Cyber-Physical, IoT, and Edge Computing网络公开度学科排名 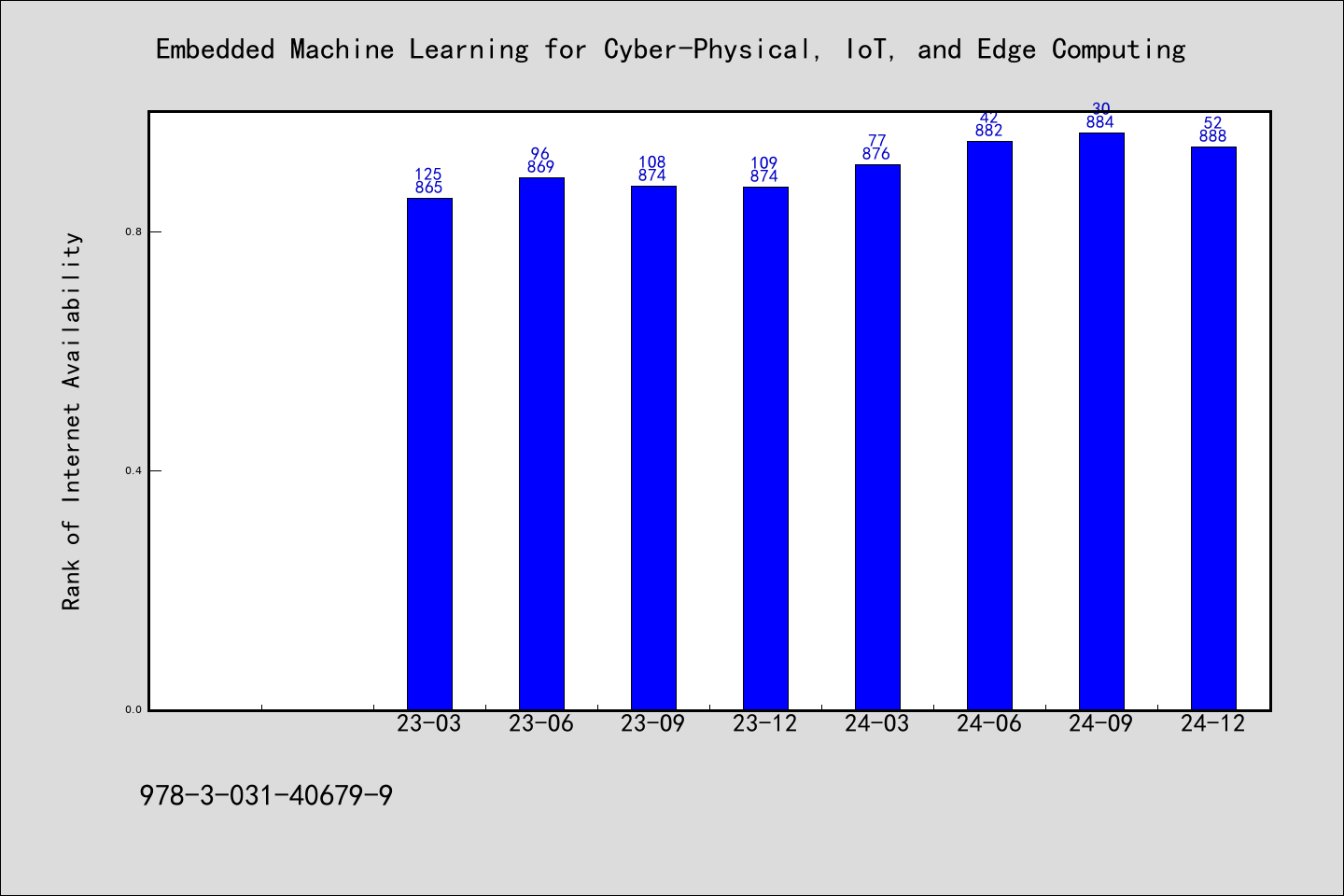
书目名称Embedded Machine Learning for Cyber-Physical, IoT, and Edge Computing被引频次 
书目名称Embedded Machine Learning for Cyber-Physical, IoT, and Edge Computing被引频次学科排名 
书目名称Embedded Machine Learning for Cyber-Physical, IoT, and Edge Computing年度引用 
书目名称Embedded Machine Learning for Cyber-Physical, IoT, and Edge Computing年度引用学科排名 
书目名称Embedded Machine Learning for Cyber-Physical, IoT, and Edge Computing读者反馈 
书目名称Embedded Machine Learning for Cyber-Physical, IoT, and Edge Computing读者反馈学科排名 
|
|
|