书目名称 | Efficient Processing of Deep Neural Networks | 编辑 | Vivienne Sze,Yu-Hsin Chen,Joel S. Emer | 视频video | | 丛书名称 | Synthesis Lectures on Computer Architecture | 图书封面 | 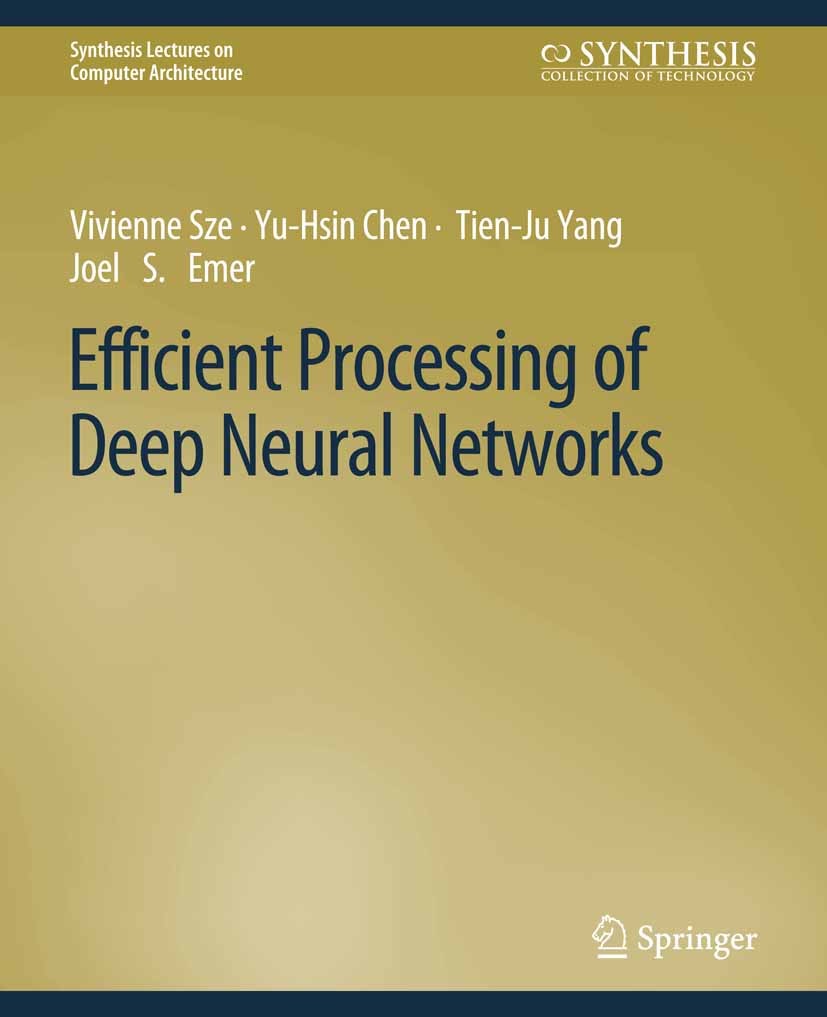 | 描述 | .This book provides a structured treatment of the key principles and techniques for enabling efficient processing of deep neural networks (DNNs).. DNNs are currently widely used for many artificial intelligence (AI) applications, including computer vision, speech recognition, and robotics. While DNNs deliver state-of-the-art accuracy on many AI tasks, it comes at the cost of high computational complexity. Therefore, techniques that enable efficient processing of deep neural networks to improve key metrics—such as energy-efficiency, throughput, and latency—without sacrificing accuracy or increasing hardware costs are critical to enabling the wide deployment of DNNs in AI systems...The book includes background on DNN processing; a description and taxonomy of hardware architectural approaches for designing DNN accelerators; key metrics for evaluating and comparing different designs; features of DNN processing that are amenable to hardware/algorithm co-design to improve energy efficiency and throughput; and opportunities for applying new technologies. Readers will find a structured introduction to the field as well as formalization and organization of key concepts from contemporary wor | 出版日期 | Book 2020 | 版次 | 1 | doi | https://doi.org/10.1007/978-3-031-01766-7 | isbn_softcover | 978-3-031-00638-8 | isbn_ebook | 978-3-031-01766-7Series ISSN 1935-3235 Series E-ISSN 1935-3243 | issn_series | 1935-3235 | copyright | Springer Nature Switzerland AG 2020 |
The information of publication is updating
|
|