书目名称 | Deep Reinforcement Learning with Python | 副标题 | RLHF for Chatbots an | 编辑 | Nimish Sanghi | 视频video | | 概述 | Explains deep reinforcement learning implementation using TensorFlow, PyTorch and OpenAI Gym.Comprehensive coverage on fine-tuning Large Language Models using RLHF with complete code examples.Every co | 图书封面 | 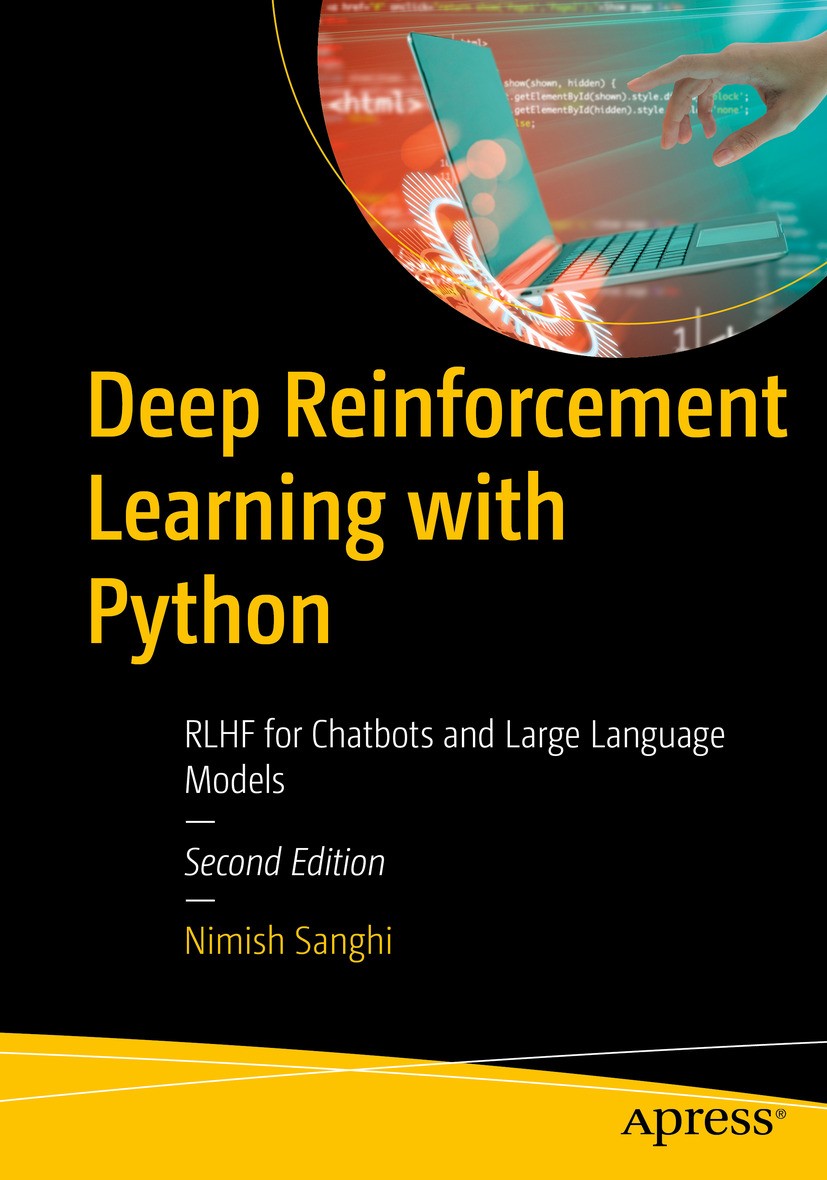 | 描述 | .Gain a theoretical understanding to the most popular libraries in deep reinforcement learning (deep RL). This new edition focuses on the latest advances in deep RL using a learn-by-coding approach, allowing readers to assimilate and replicate the latest research in this field. .New agent environments ranging from games, and robotics to finance are explained to help you try different ways to apply reinforcement learning. A chapter on multi-agent reinforcement learning covers how multiple agents compete, while another chapter focuses on the widely used deep RL algorithm, proximal policy optimization (PPO). You‘ll see how reinforcement learning with human feedback (RLHF) has been used by chatbots, built using Large Language Models, e.g. ChatGPT to improve conversational capabilities..You‘ll also review the steps for using the code on multiple cloud systems and deploying models on platforms such as Hugging Face Hub. The code is in Jupyter Notebook, which canbe run on Google Colab, and other similar deep learning cloud platforms, allowing you to tailor the code to your own needs. .Whether it’s for applications in gaming, robotics, or Generative AI, .Deep Reinforcement Learning with Py | 出版日期 | Book 2024Latest edition | 关键词 | Artificial Intelligence; Deep Reinforcement Learning; PyTorch; Neural Networks; Robotics; Autonomous Vehi | 版次 | 2 | doi | https://doi.org/10.1007/979-8-8688-0273-7 | isbn_softcover | 979-8-8688-0272-0 | isbn_ebook | 979-8-8688-0273-7 | copyright | Nimish Sanghi 2024 |
The information of publication is updating
|
|