书目名称 | Deep Generative Models | 副标题 | 4th MICCAI Workshop, | 编辑 | Anirban Mukhopadhyay,Ilkay Oksuz,Yixuan Yuan | 视频video | | 丛书名称 | Lecture Notes in Computer Science | 图书封面 | 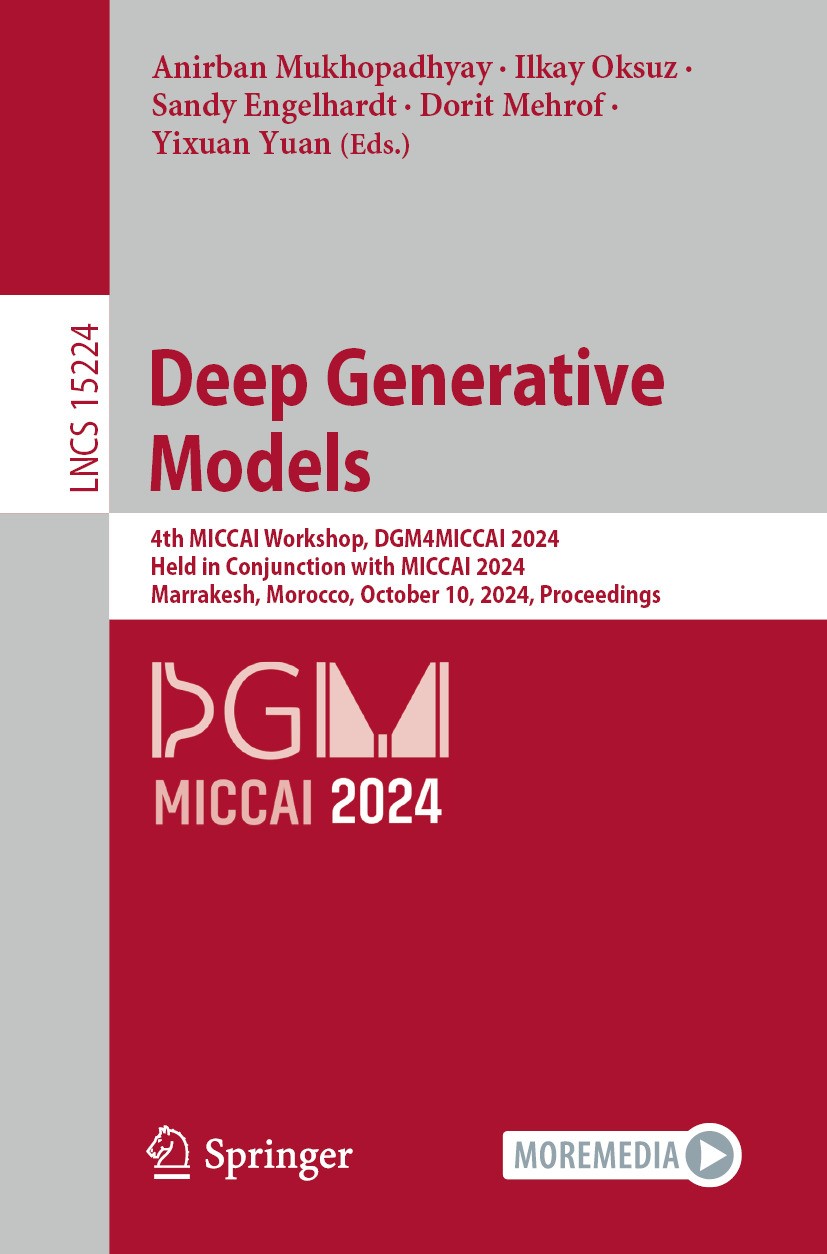 | 描述 | .This book constitutes the proceedings of the 4th workshop on Deep Generative Models for Medical Image Computing and Computer Assisted Intervention, DGM4MICCAI 2024, held in conjunction with the 27th International conference on Medical Image Computing and Computer Assisted Intervention, MICCAI 2024, in Marrakesh, Morocco in October 2024...The 21 papers presented here were carefully reviewed and selected from 40 submissions. These papers deal with a broad range of topics, ranging from methodology (such as Causal inference, Latent interpretation, Generative factor analysis) to Applications (such as Mammography, Vessel imaging, Surgical videos and more).. | 出版日期 | Conference proceedings 2025 | 关键词 | Diffusion; VAE; GAN; Flow; Synthesis; Privacy; GenAI | 版次 | 1 | doi | https://doi.org/10.1007/978-3-031-72744-3 | isbn_softcover | 978-3-031-72743-6 | isbn_ebook | 978-3-031-72744-3Series ISSN 0302-9743 Series E-ISSN 1611-3349 | issn_series | 0302-9743 | copyright | The Editor(s) (if applicable) and The Author(s), under exclusive license to Springer Nature Switzerl |
The information of publication is updating
|
|