书目名称 | Deep Generative Modeling | 编辑 | Jakub M. Tomczak | 视频video | | 概述 | Comprehensive explanation of Generative AI techniques, providing code snippets for all presented models.Revised and expanded edition with new chapters on LLMs, Gen AI systems, and Probabilistic Modeli | 图书封面 | 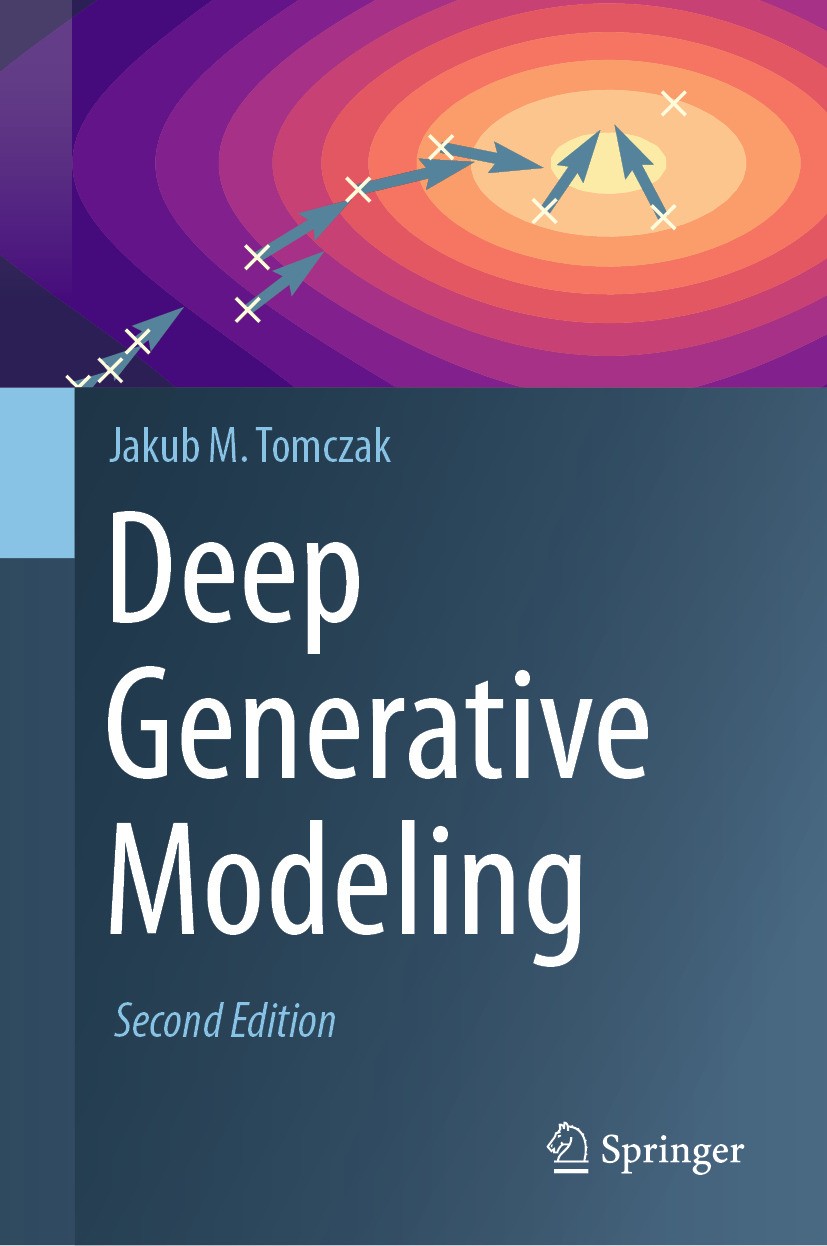 | 描述 | .This first comprehensive book on models behind Generative AI has been thoroughly revised to cover all major classes of deep generative models: mixture models, Probabilistic Circuits, Autoregressive Models, Flow-based Models, Latent Variable Models, GANs, Hybrid Models, Score-based Generative Models, Energy-based Models, and Large Language Models. In addition, Generative AI Systems are discussed, demonstrating how deep generative models can be used for neural compression, among others...Deep Generative Modeling is designed to appeal to curious students, engineers, and researchers with a modest mathematical background in undergraduate calculus, linear algebra, probability theory, and the basics of machine learning, deep learning, and programming in Python and PyTorch (or other deep learning libraries). It should find interest among students and researchers from a variety of backgrounds, including computer science, engineering, data science, physics, and bioinformatics who wish to get familiar with deep generative modeling..In order to engage with a reader, the book introduces fundamental concepts with specific examples and code snippets. The full code accompanying the book is availa | 出版日期 | Textbook 2024Latest edition | 关键词 | Generative AI; Large Language Models; Autoregressive models; Diffusion models; Score-based Generative Mo | 版次 | 2 | doi | https://doi.org/10.1007/978-3-031-64087-2 | isbn_softcover | 978-3-031-64089-6 | isbn_ebook | 978-3-031-64087-2 | copyright | The Editor(s) (if applicable) and The Author(s), under exclusive license to Springer Nature Switzerl |
The information of publication is updating
|
|