书目名称 | Data Engineering in Medical Imaging |
副标题 | Second MICCAI Worksh |
编辑 | Binod Bhattarai,Sharib Ali,Danail Stoyanov |
视频video | |
丛书名称 | Lecture Notes in Computer Science |
图书封面 | 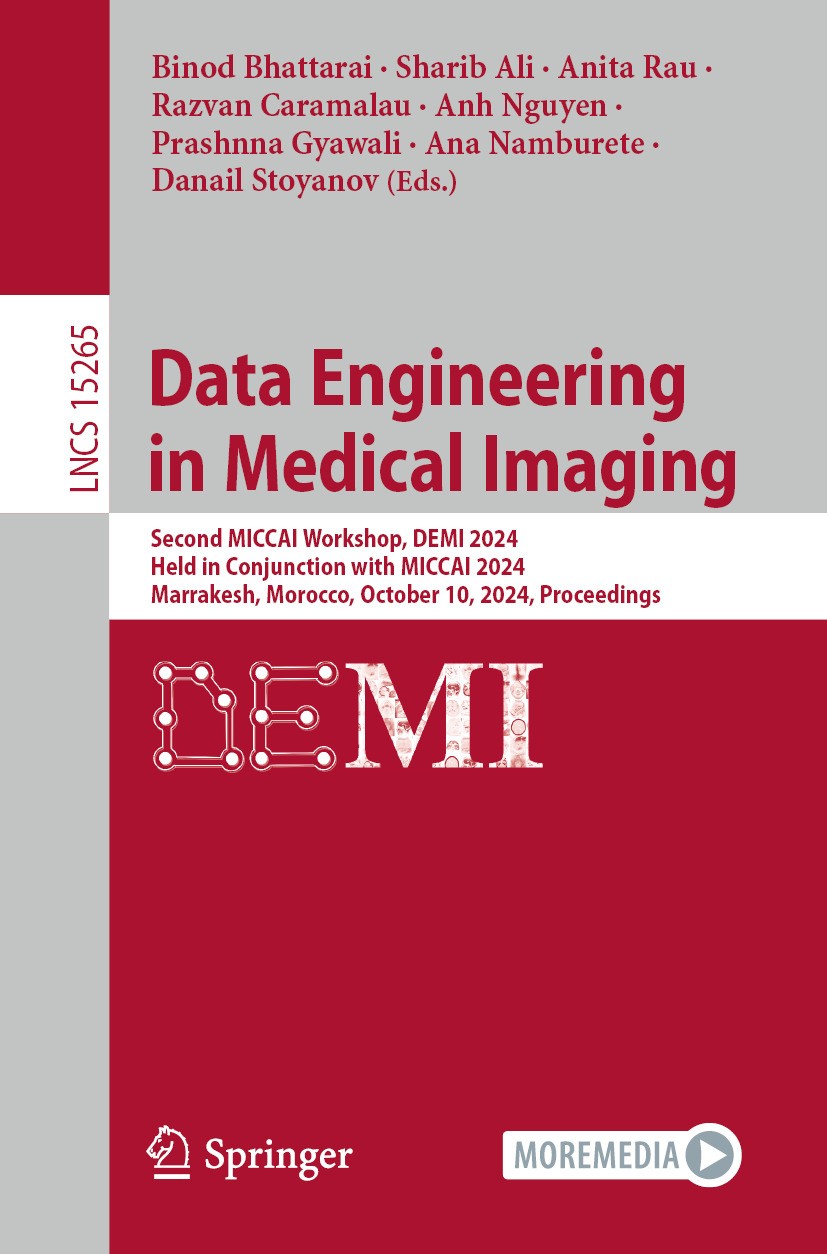 |
描述 | .This book constitutes the proceedings of the Second MICCAI Workshop on Data Engineering in Medical Imaging, DEMI 2024, held in conjunction with the 27th International conference on Medical Image Computing and Computer Assisted Intervention, MICCAI 2024, in Marrakesh, Morocco, on October 10, 2024...The 18 papers presented in this book were carefully reviewed and selected. These papers focus on the application of various Data engineering techniques in the field of Medical Imaging... .. . |
出版日期 | Conference proceedings 2025 |
关键词 | data augmentation; synthetic data; active learning; medical imaging; data synthesis; federated learning; m |
版次 | 1 |
doi | https://doi.org/10.1007/978-3-031-73748-0 |
isbn_softcover | 978-3-031-73747-3 |
isbn_ebook | 978-3-031-73748-0Series ISSN 0302-9743 Series E-ISSN 1611-3349 |
issn_series | 0302-9743 |
copyright | The Editor(s) (if applicable) and The Author(s), under exclusive license to Springer Nature Switzerl |