书目名称 | Dynamic Network Representation Based on Latent Factorization of Tensors | 编辑 | Hao Wu,Xuke Wu,Xin Luo | 视频video | | 概述 | Exposes readers to a novel research perspective regarding dynamic network representation.Presents four dynamic network representation methods based on latent factorization of tensors.Accomplishes accu | 丛书名称 | SpringerBriefs in Computer Science | 图书封面 | 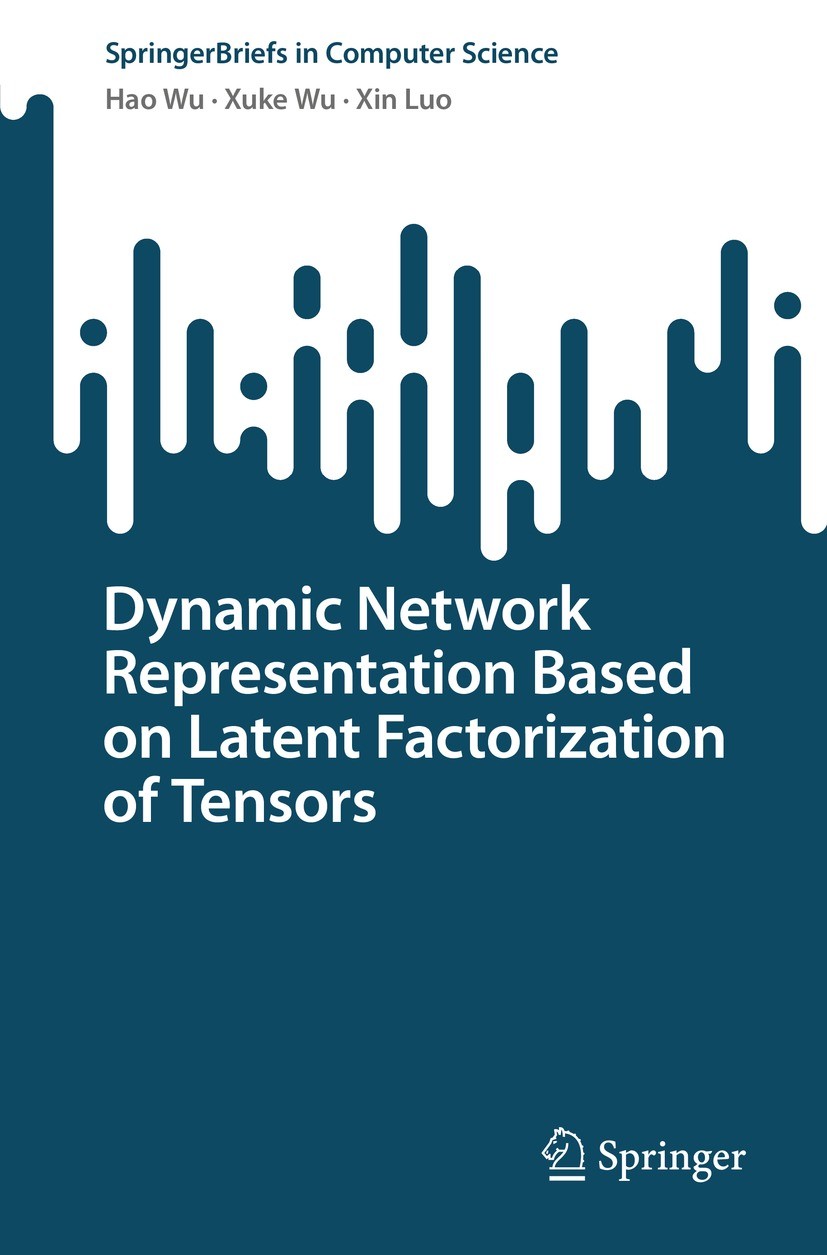 | 描述 | .A dynamic network is frequently encountered in various real industrial applications, such as the Internet of Things. It is composed of numerous nodes and large-scale dynamic real-time interactions among them, where each node indicates a specified entity, each directed link indicates a real-time interaction, and the strength of an interaction can be quantified as the weight of a link. As the involved nodes increase drastically, it becomes impossible to observe their full interactions at each time slot, making a resultant dynamic network High Dimensional and Incomplete (HDI). An HDI dynamic network with directed and weighted links, despite its HDI nature, contains rich knowledge regarding involved nodes’ various behavior patterns. Therefore, it is essential to study how to build efficient and effective representation learning models for acquiring useful knowledge...In this book, we first model a dynamic network into an HDI tensor and present the basic latent factorization of tensors (LFT) model. Then, we propose four representative LFT-based network representation methods. The first method integrates the short-time bias, long-time bias and preprocessing bias to precisely represent t | 出版日期 | Book 2023 | 关键词 | Dynamic network representation; Latent factorization of tensors; High-dimensional and incomplete tenso | 版次 | 1 | doi | https://doi.org/10.1007/978-981-19-8934-6 | isbn_softcover | 978-981-19-8933-9 | isbn_ebook | 978-981-19-8934-6Series ISSN 2191-5768 Series E-ISSN 2191-5776 | issn_series | 2191-5768 | copyright | The Editor(s) (if applicable) and The Author(s), under exclusive license to Springer Nature Singapor |
The information of publication is updating
|
|