书目名称 | Domain Adaptation for Visual Understanding | 编辑 | Richa Singh,Mayank Vatsa,Nalini Ratha | 视频video | | 概述 | Presents the latest research on domain adaptation for visual understanding.Provides perspectives from an international selection of authorities in the field.Reviews a variety of applications and techn | 图书封面 | 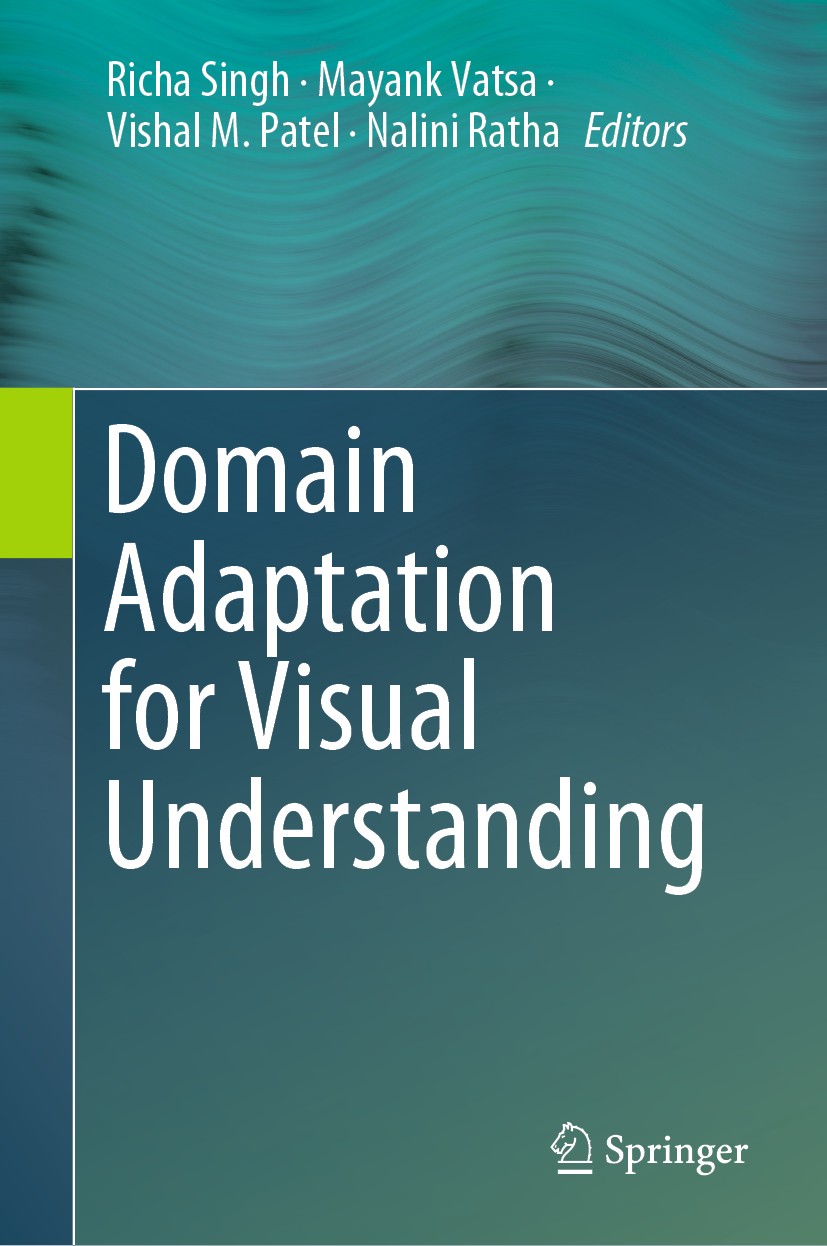 | 描述 | .This unique volume reviews the latest advances in domain adaptation in the training of machine learning algorithms for visual understanding, offering valuable insights from an international selection of experts in the field. The text presents a diverse selection of novel techniques, covering applications of object recognition, face recognition, and action and event recognition..Topics and features: reviews the domain adaptation-based machine learning algorithms available for visual understanding, and provides a deep metric learning approach; introduces a novel unsupervised method for image-to-image translation, and a video segment retrieval model that utilizes ensemble learning; proposes a unique way to determine which dataset is most useful in the base training, in order to improve the transferability of deep neural networks; describes a quantitative method for estimating the discrepancy between the source and target data to enhance image classification performance; presentsa technique for multi-modal fusion that enhances facial action recognition, and a framework for intuition learning in domain adaptation; examines an original interpolation-based approach to address the issue o | 出版日期 | Book 2020 | 关键词 | Domain Adaptation; Machine Learning; Computer Vision; Representation Learning; Transfer Learning; Generat | 版次 | 1 | doi | https://doi.org/10.1007/978-3-030-30671-7 | isbn_softcover | 978-3-030-30673-1 | isbn_ebook | 978-3-030-30671-7 | copyright | Springer Nature Switzerland AG 2020 |
The information of publication is updating
|
|