书目名称 | Deployable Machine Learning for Security Defense |
副标题 | First International |
编辑 | Gang Wang,Arridhana Ciptadi,Ali Ahmadzadeh |
视频video | |
丛书名称 | Communications in Computer and Information Science |
图书封面 | 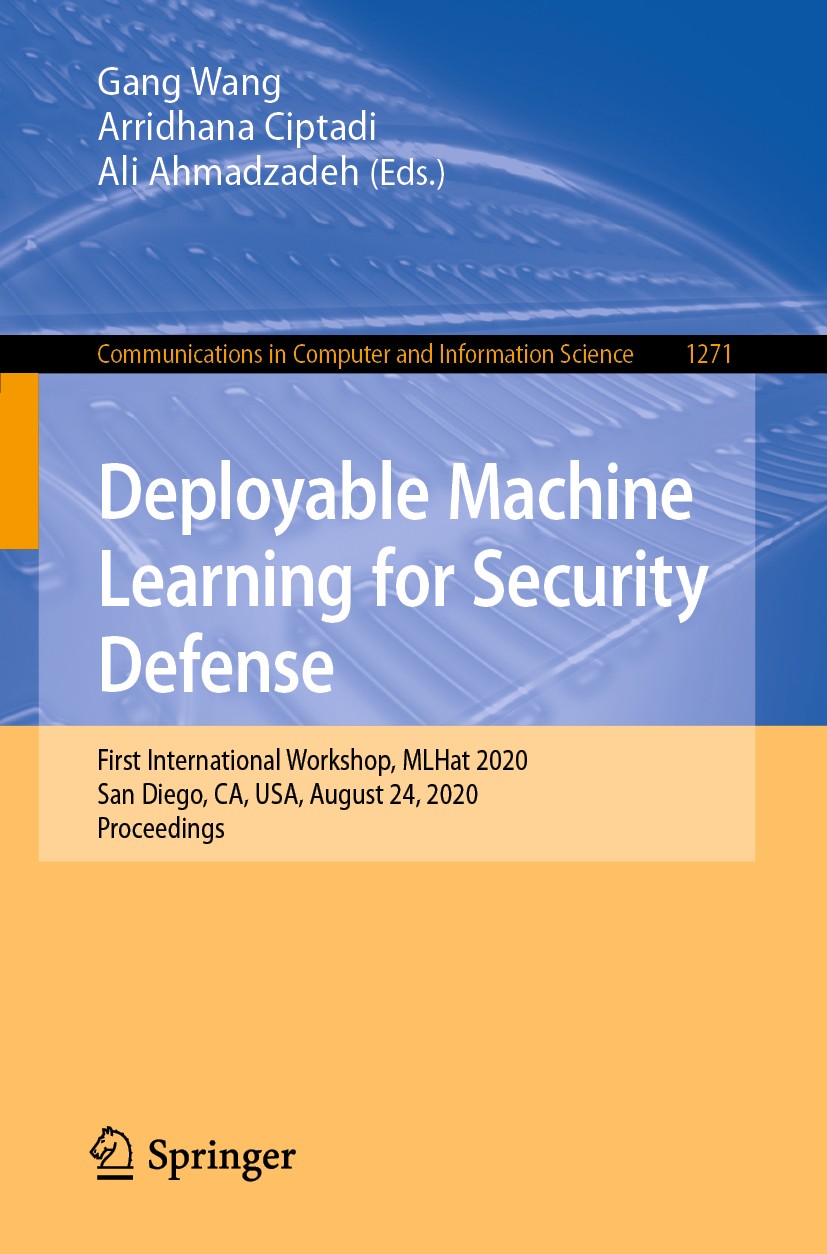 |
描述 | This book constitutes selected papers from the First International Workshop on Deployable Machine Learning for Security Defense, MLHat 2020, held in August 2020. Due to the COVID-19 pandemic the conference was held online. .The 8 full papers were thoroughly reviewed and selected from 13 qualified submissions. The papers are organized in the following topical sections: understanding the adversaries; adversarial ML for better security; threats on networks.. |
出版日期 | Conference proceedings 2020 |
关键词 | artificial intelligence; computer crime; computer security; computer systems; cryptography; cyber-attacks |
版次 | 1 |
doi | https://doi.org/10.1007/978-3-030-59621-7 |
isbn_softcover | 978-3-030-59620-0 |
isbn_ebook | 978-3-030-59621-7Series ISSN 1865-0929 Series E-ISSN 1865-0937 |
issn_series | 1865-0929 |
copyright | Springer Nature Switzerland AG 2020 |