书目名称 | Deep Neural Networks and Data for Automated Driving | 副标题 | Robustness, Uncertai | 编辑 | Tim Fingscheidt,Hanno Gottschalk,Sebastian Houben | 视频video | | 概述 | Presents the latest developments from industry and research on automated driving and artificial intelligence.Provides in introduction to current knowledge in neural networks and AI.Provides a basis fo | 图书封面 | 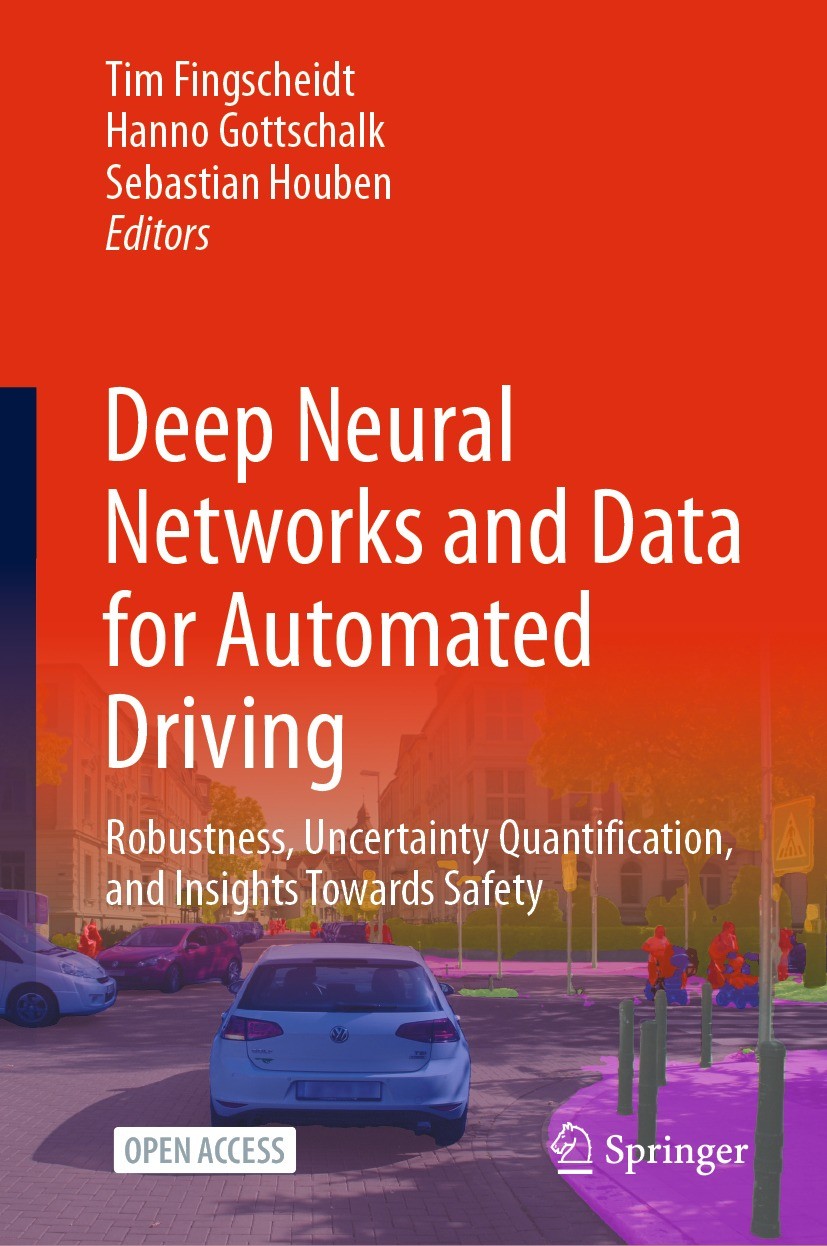 | 描述 | .This open access book brings together the latest developments from industry and research on automated driving and artificial intelligence..Environment perception for highly automated driving heavily employs deep neural networks, facing many challenges. How much data do we need for training and testing? How to use synthetic data to save labeling costs for training? How do we increase robustness and decrease memory usage? For inevitably poor conditions: How do we know that the network is uncertain about its decisions? Can we understand a bit more about what actually happens inside neural networks? This leads to a very practical problem particularly for DNNs employed in automated driving: What are useful validation techniques and how about safety?.This book unites the views from both academia and industry, where computer vision and machine learning meet environment perception for highly automated driving. Naturally, aspects of data, robustness, uncertainty quantification, and,last but not least, safety are at the core of it. This book is unique: In its first part, an extended survey of all the relevant aspects is provided. The second part contains the detailed technical elaboration o | 出版日期 | Book‘‘‘‘‘‘‘‘ 2022 | 关键词 | Highly Automated Driving; Autonomous Driving; Environment Perception; Deep Learning; Safety; Open Access | 版次 | 1 | doi | https://doi.org/10.1007/978-3-031-01233-4 | isbn_softcover | 978-3-031-01235-8 | isbn_ebook | 978-3-031-01233-4 | copyright | The Editor(s) (if applicable) and The Author(s) 2022 |
The information of publication is updating
|
|