书目名称 | Deep Learning in Solar Astronomy | 编辑 | Long Xu,Yihua Yan,Xin Huang | 视频video | | 概述 | Explore techniques of deep learning to scientific research of solar astronomy, including applications of deep learning..Present datasets of solar activity events and training samples for training deep | 丛书名称 | SpringerBriefs in Computer Science | 图书封面 | 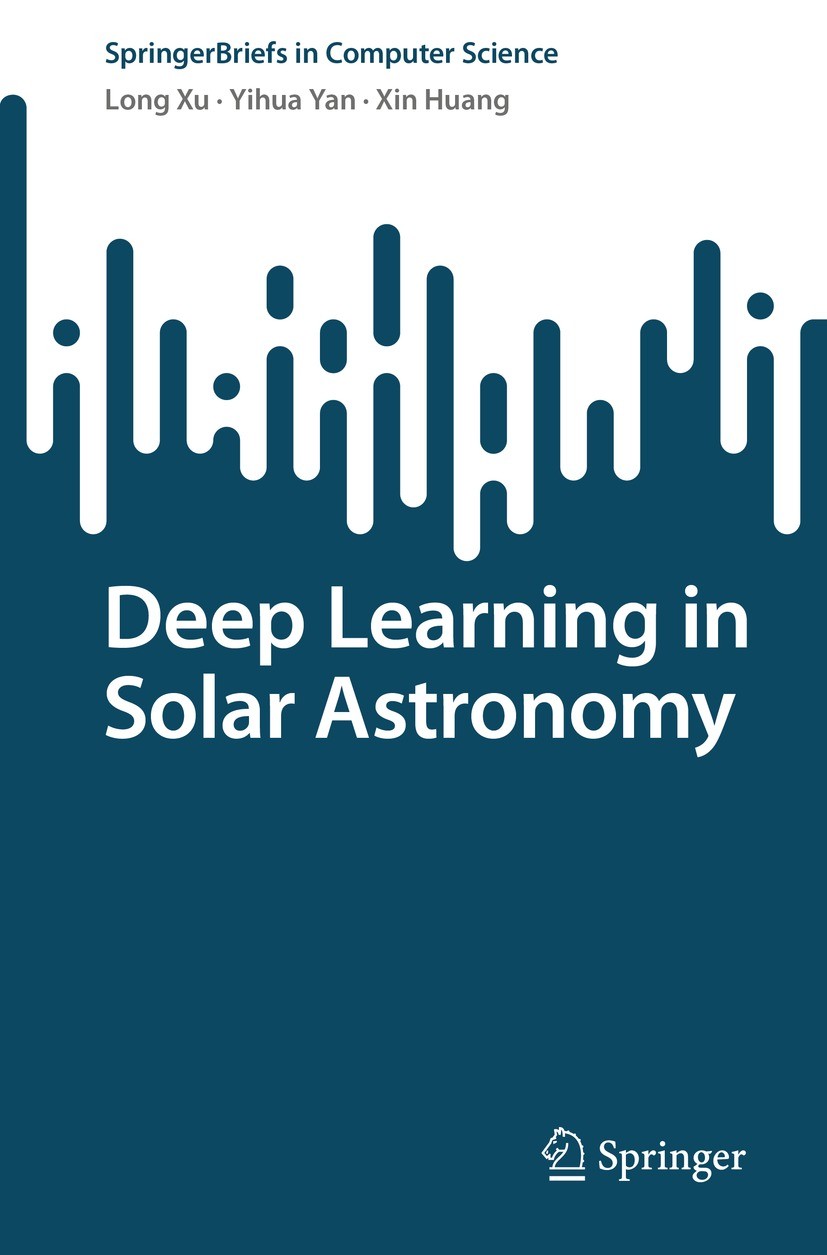 | 描述 | .The volume of data being collected in solar astronomy has exponentially increased over the past decade and we will be entering the age of petabyte solar data. Deep learning has been an invaluable tool exploited to efficiently extract key information from the massive solar observation data, to solve the tasks of data archiving/classification, object detection and recognition...Astronomical study starts with imaging from recorded raw data, followed by image processing, such as image reconstruction, inpainting and generation, to enhance imaging quality. We study deep learning for solar image processing. First, image deconvolution is investigated for synthesis aperture imaging. Second, image inpainting is explored to repair over-saturated solar image due to light intensity beyond threshold of optical lens. Third, image translation among UV/EUV observation of the chromosphere/corona, Ha observation of the chromosphere and magnetogram of the photosphere is realized by using GAN, exhibiting powerful image domain transfer ability among multiple wavebands and different observation devices. It can compensate the lack of observation time or waveband. In addition, time series model, e.g., LST | 出版日期 | Book 2022 | 关键词 | solar astronomy; solar radio spectrum; solar image classification; solar image generation; deep Learning | 版次 | 1 | doi | https://doi.org/10.1007/978-981-19-2746-1 | isbn_softcover | 978-981-19-2745-4 | isbn_ebook | 978-981-19-2746-1Series ISSN 2191-5768 Series E-ISSN 2191-5776 | issn_series | 2191-5768 | copyright | The Editor(s) (if applicable) and The Author(s), under exclusive license to Springer Nature Singapor |
The information of publication is updating
|
|