书目名称 | Deep Learning in Computational Mechanics |
副标题 | An Introductory Cour |
编辑 | Stefan Kollmannsberger,Davide D‘Angella,Leon Herrm |
视频video | |
概述 | Introduces to the adaption of learning-based methods in the domain of computational mechanics.Presents fundamental concepts of Machine Learning, Neural Networks and their corresponding algorithms.Revi |
丛书名称 | Studies in Computational Intelligence |
图书封面 | 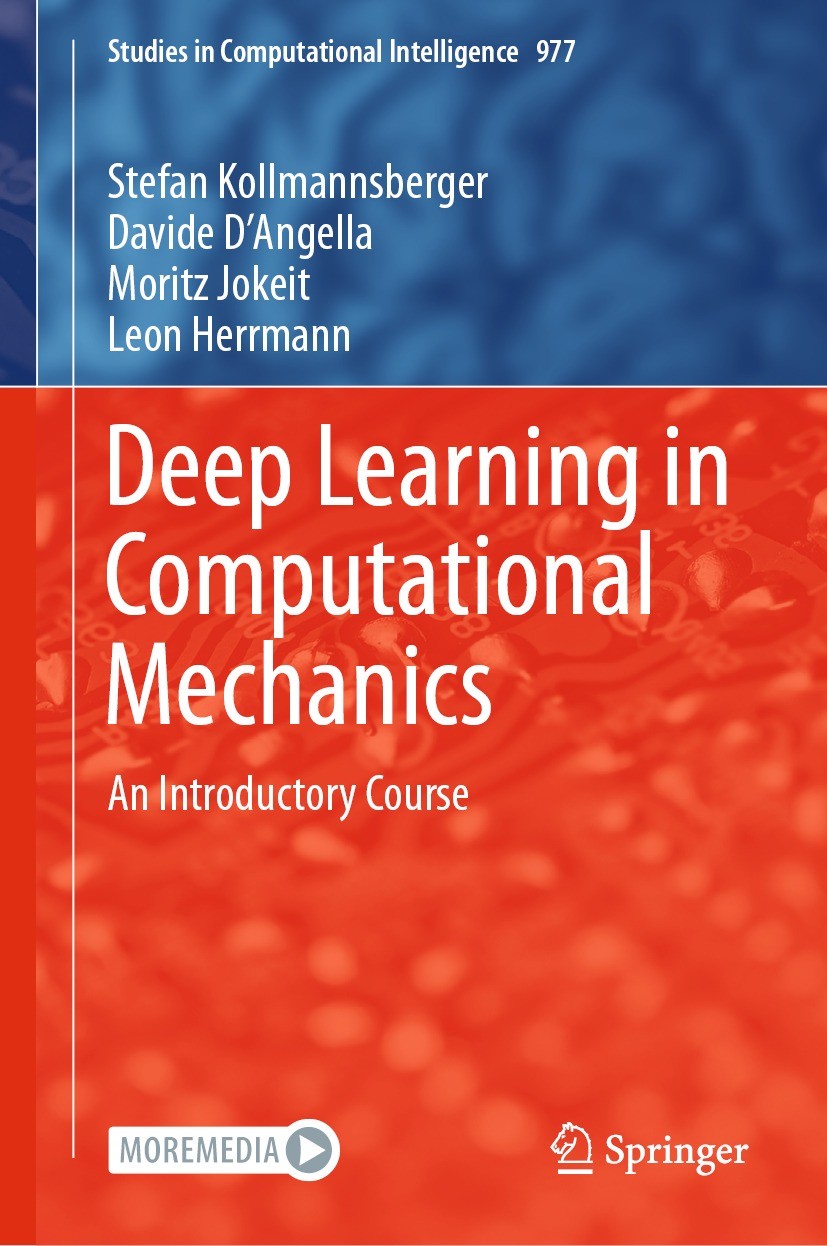 |
描述 | .This book provides a first course on deep learning in computational mechanics. The book starts with a short introduction to machine learning’s fundamental concepts before neural networks are explained thoroughly. It then provides an overview of current topics in physics and engineering, setting the stage for the book’s main topics: physics-informed neural networks and the deep energy method..The idea of the book is to provide the basic concepts in a mathematically sound manner and yet to stay as simple as possible. To achieve this goal, mostly one-dimensional examples are investigated, such as approximating functions by neural networks or the simulation of the temperature’s evolution in a one-dimensional bar..Each chapter contains examples and exercises which are either solved analytically or in PyTorch, an open-source machine learning framework for python.. . . |
出版日期 | Textbook 2021 |
关键词 | Computational Intelligence; Artificial Intelligence; Computational Mechanics; Neural Networks; Machine L |
版次 | 1 |
doi | https://doi.org/10.1007/978-3-030-76587-3 |
isbn_softcover | 978-3-030-76589-7 |
isbn_ebook | 978-3-030-76587-3Series ISSN 1860-949X Series E-ISSN 1860-9503 |
issn_series | 1860-949X |
copyright | The Editor(s) (if applicable) and The Author(s), under exclusive license to Springer Nature Switzerl |