书目名称 | Deep Learning and Missing Data in Engineering Systems |
编辑 | Collins Achepsah Leke,Tshilidzi Marwala |
视频video | |
概述 | Adopts and applies swarm intelligence algorithms to address critical questions such as model selection and model parameter estimation.Proposes new paradigms of machine learning and computational intel |
丛书名称 | Studies in Big Data |
图书封面 | 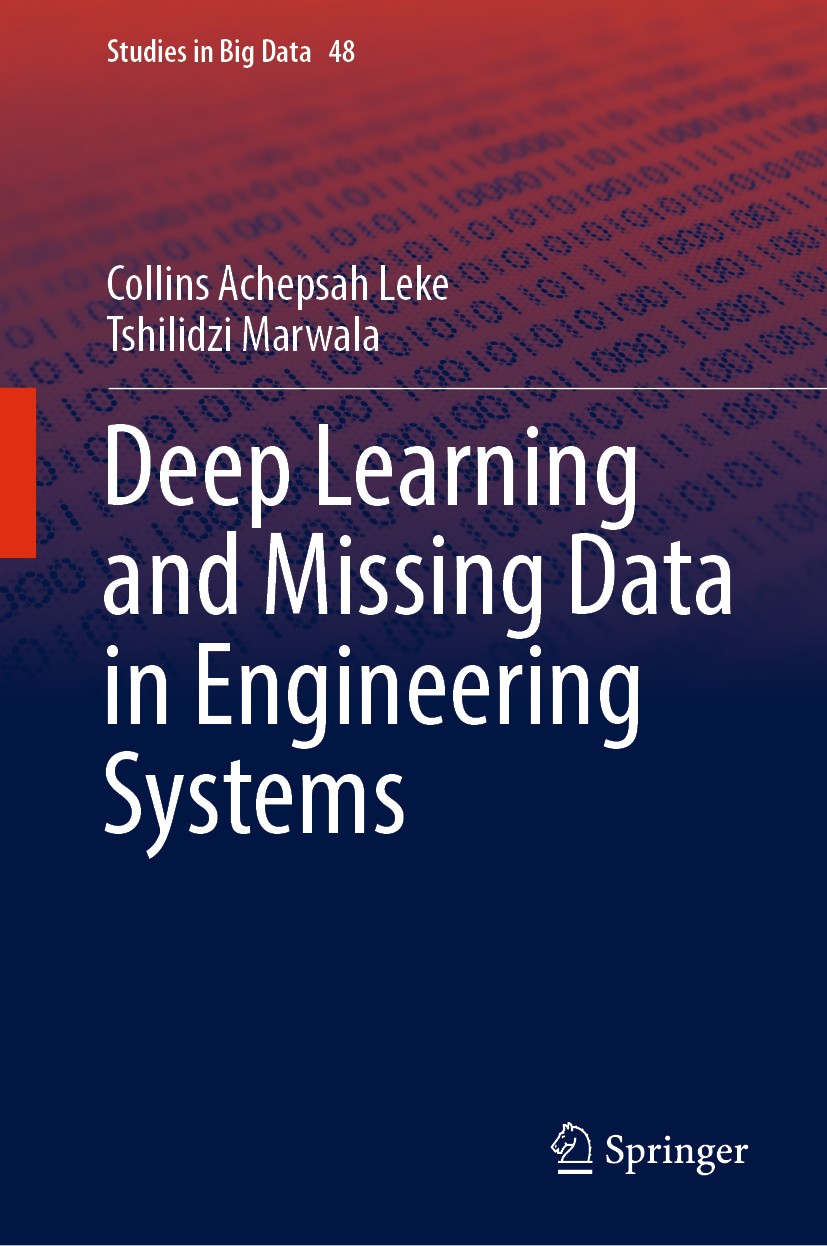 |
描述 | .Deep Learning and Missing Data in Engineering Systems. uses deep learning and swarm intelligence methods to cover missing data estimation in engineering systems. The missing data estimation processes proposed in the book can be applied in image recognition and reconstruction. To facilitate the imputation of missing data, several artificial intelligence approaches are presented, including:.deep autoencoder neural networks;.deep denoising autoencoder networks;.the bat algorithm;.the cuckoo search algorithm; and.the firefly algorithm.. .The hybrid models proposed are used to estimate the missing data in high-dimensional data settings more accurately. Swarm intelligence algorithms are applied to address critical questions such as model selection and model parameter estimation. The authors address feature extraction for the purpose of reconstructing the input data from reduced dimensions by the use of deep autoencoder neural networks. They illustrate new models diagrammatically, report their findings in tables, so as to put their methods on a sound statistical basis. The methods proposed speed up the process of data estimation while preserving known features of the data matrix. ..This |
出版日期 | Book 2019 |
关键词 | Artificial Intelligence; Missing Data Estimation; Deep Learning; Swarm Intelligence; Machine Learning; Mo |
版次 | 1 |
doi | https://doi.org/10.1007/978-3-030-01180-2 |
isbn_ebook | 978-3-030-01180-2Series ISSN 2197-6503 Series E-ISSN 2197-6511 |
issn_series | 2197-6503 |
copyright | Springer Nature Switzerland AG 2019 |