书目名称 | Deep Learning Techniques for Music Generation | 编辑 | Jean-Pierre Briot,Gaëtan Hadjeres,François-David P | 视频video | | 概述 | Authors‘ analysis based on five dimensions: objective, representation, architecture, challenge, and strategy.Important application of deep learning, for AI researchers and composers.Research was condu | 丛书名称 | Computational Synthesis and Creative Systems | 图书封面 | 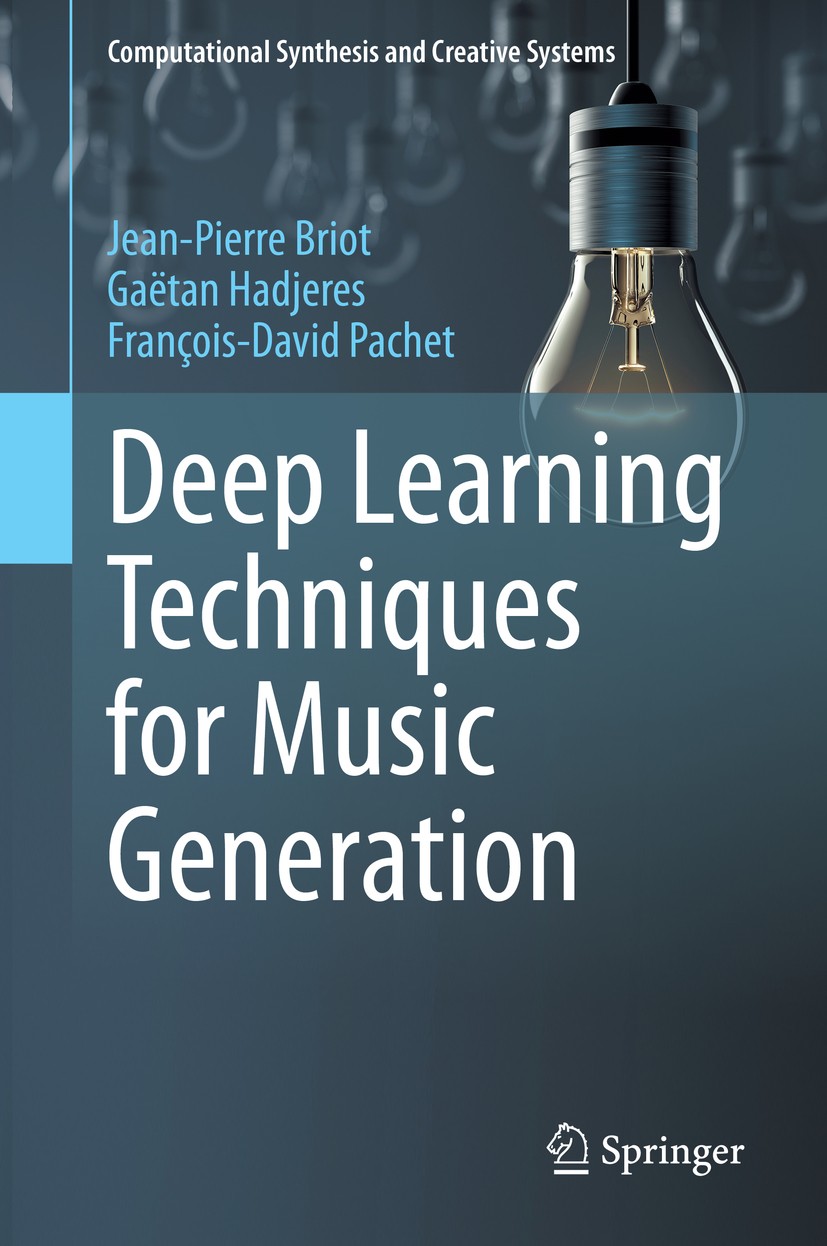 | 描述 | .This book is a survey and analysis of how deep learning can be used to generate musical content. The authors offer a comprehensive presentation of the foundations of deep learning techniques for music generation. They also develop a conceptual framework used to classify and analyze various types of architecture, encoding models, generation strategies, and ways to control the generation. The five dimensions of this framework are: objective (the kind of musical content to be generated, e.g., melody, accompaniment); representation (the musical elements to be considered and how to encode them, e.g., chord, silence, piano roll, one-hot encoding); architecture (the structure organizing neurons, their connexions, and the flow of their activations, e.g., feedforward, recurrent, variational autoencoder); challenge (the desired properties and issues, e.g., variability, incrementality, adaptability); and strategy (the way to model and control the process of generation, e.g., single-step feedforward, iterative feedforward, decoder feedforward, sampling). To illustrate the possible design decisions and to allow comparison and correlation analysis they analyze and classify more than 40 systems, | 出版日期 | Book 2020 | 关键词 | Music Generation; Machine Learning; Deep Learning; Neural Networks; Representation; Artificial Intelligen | 版次 | 1 | doi | https://doi.org/10.1007/978-3-319-70163-9 | isbn_ebook | 978-3-319-70163-9Series ISSN 2509-6575 Series E-ISSN 2509-6583 | issn_series | 2509-6575 | copyright | Springer Nature Switzerland AG 2020 |
The information of publication is updating
|
|