书目名称 | Deep Generative Models |
副标题 | Second MICCAI Worksh |
编辑 | Anirban Mukhopadhyay,Ilkay Oksuz,Yixuan Yuan |
视频video | |
丛书名称 | Lecture Notes in Computer Science |
图书封面 | 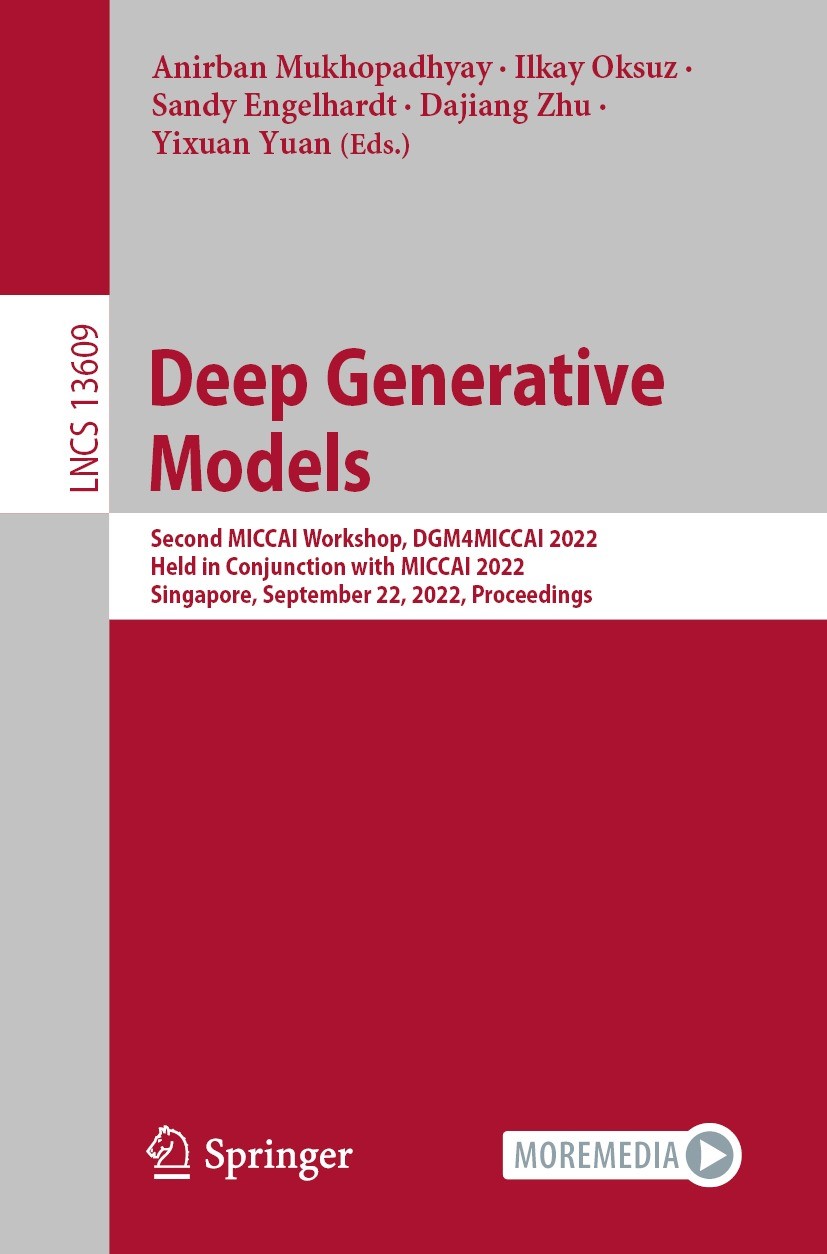 |
描述 | This book constitutes the refereed proceedings of the Second MICCAI Workshop on Deep Generative Models, DG4MICCAI 2022, held in conjunction with MICCAI 2022, in September 2022. The workshops took place in Singapore. .DG4MICCAI 2022 accepted 12 papers from the 15 submissions received. The workshop focusses on recent algorithmic developments, new results, and promising future directions in Deep Generative Models. Deep generative models such as Generative Adversarial Network (GAN) and Variational Auto-Encoder (VAE) are currently receiving widespread attention from not only the computer vision and machine learning communities, but also in the MIC and CAI community.. |
出版日期 | Conference proceedings 2022 |
关键词 | artificial intelligence; bioinformatics; color image processing; color images; computer vision; digital i |
版次 | 1 |
doi | https://doi.org/10.1007/978-3-031-18576-2 |
isbn_softcover | 978-3-031-18575-5 |
isbn_ebook | 978-3-031-18576-2Series ISSN 0302-9743 Series E-ISSN 1611-3349 |
issn_series | 0302-9743 |
copyright | The Editor(s) (if applicable) and The Author(s), under exclusive license to Springer Nature Switzerl |