书目名称 | Data Science and Productivity Analytics | 编辑 | Vincent Charles,Juan Aparicio,Joe Zhu | 视频video | | 概述 | First book to combine DEA and Data Science.Editors and Contributors at the forefront of field worldwide.Illustrates how Data Science techniques can unleash value and drive productivity | 丛书名称 | International Series in Operations Research & Management Science | 图书封面 | 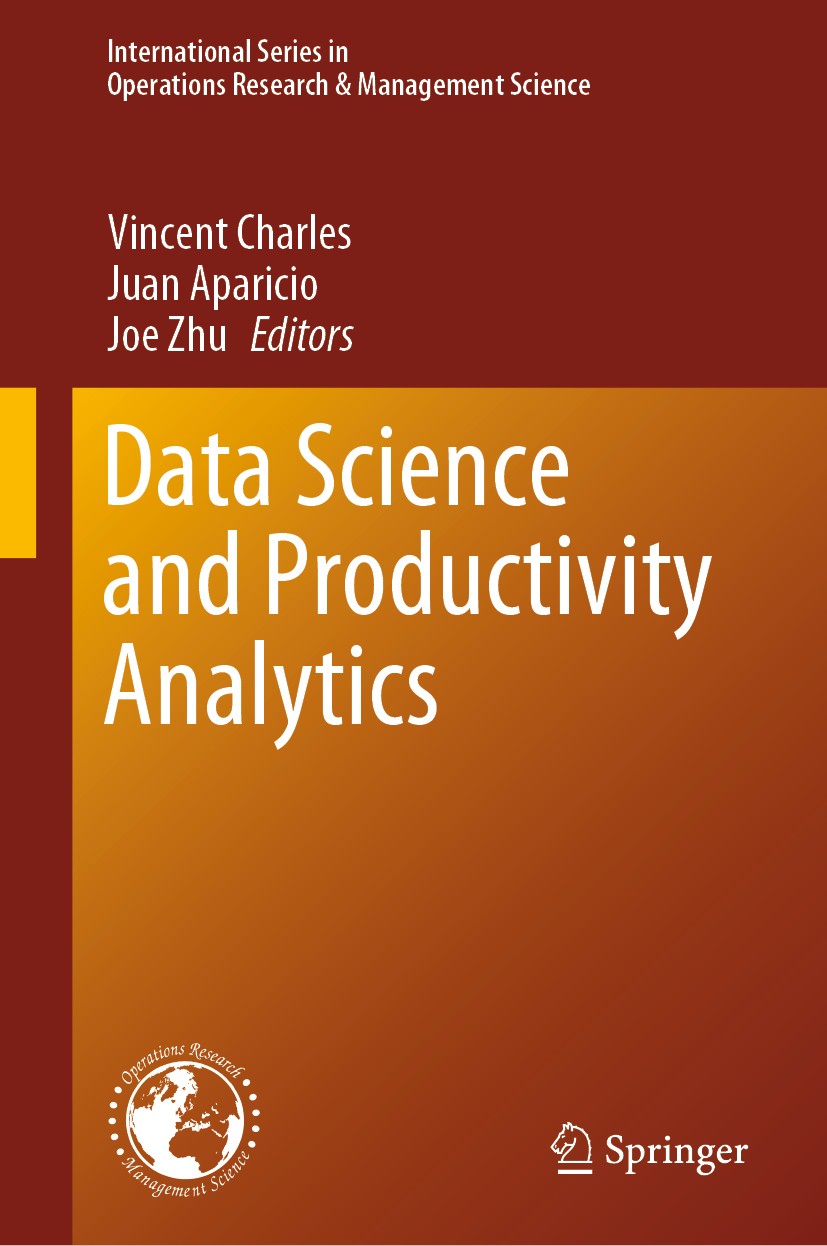 | 描述 | .This book includes a spectrum of concepts, such as performance, productivity, operations research, econometrics, and data science, for the practically and theoretically important areas of ‘productivity analysis/data envelopment analysis’ and ‘data science/big data’. Data science is defined as the collection of scientific methods, processes, and systems dedicated to extracting knowledge or insights from data and it develops on concepts from various domains, containing mathematics and statistical methods, operations research, machine learning, computer programming, pattern recognition, and data visualisation, among others...Examples of data science techniques include linear and logistic regressions, decision trees, Naïve Bayesian classifier, principal component analysis, neural networks, predictive modelling, deep learning, text analysis, survival analysis, and so on, all of which allow using the data to make more intelligent decisions. On the other hand, it is without a doubtthat nowadays the amount of data is exponentially increasing, and analysing large data sets has become a key basis of competition and innovation, underpinning new waves of productivity growth. This book aims to | 出版日期 | Book 2020 | 关键词 | Productivity Analysis; Data Science; Data Envelopment Analysis (DEA); Big Data; Efficiency; Parametric An | 版次 | 1 | doi | https://doi.org/10.1007/978-3-030-43384-0 | isbn_softcover | 978-3-030-43386-4 | isbn_ebook | 978-3-030-43384-0Series ISSN 0884-8289 Series E-ISSN 2214-7934 | issn_series | 0884-8289 | copyright | Springer Nature Switzerland AG 2020 |
The information of publication is updating
|
|