书目名称 | Data Augmentation, Labelling, and Imperfections | 副标题 | Third MICCAI Worksho | 编辑 | Yuan Xue,Chen Chen,Yihao Liu | 视频video | | 丛书名称 | Lecture Notes in Computer Science | 图书封面 | 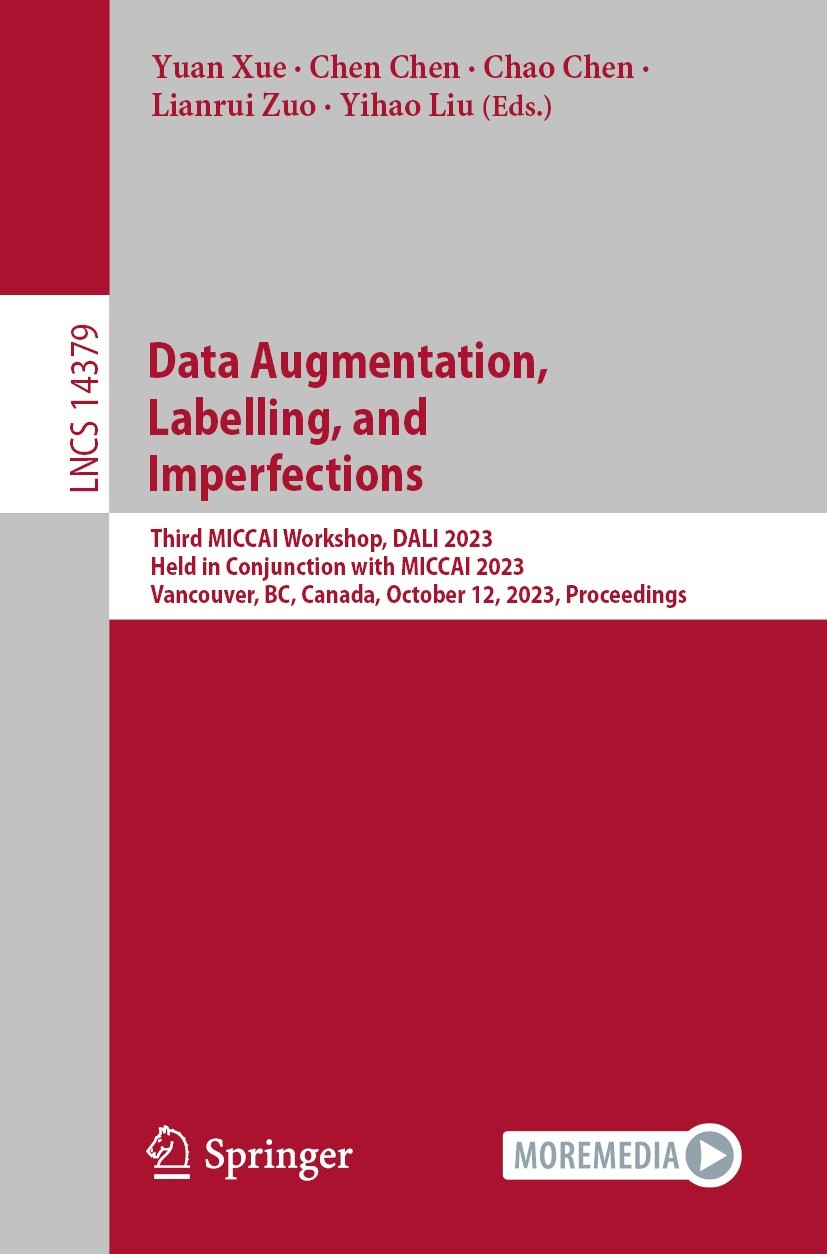 | 描述 | .This LNCS conference volume constitutes the proceedings of the 3rd International Workshop on..Data Augmentation, Labeling, and Imperfections (DALI 2023), held on October 12, 2023, in Vancouver, Canada, in conjunction with the 26th International..Conference on Medical Image Computing and Computer Assisted Intervention..(MICCAI 2023). The 16 full papers together in this volume were carefully reviewed and selected from 23 submissions...The conference fosters a collaborative environment for addressing the critical challenges associated with medical data, particularly focusing on data, labeling, and dealing with data imperfections in the context of medical image analysis.. | 出版日期 | Conference proceedings 2024 | 关键词 | artificial intelligence; bioinformatics; color image processing; color images; computer systems; computer | 版次 | 1 | doi | https://doi.org/10.1007/978-3-031-58171-7 | isbn_softcover | 978-3-031-58170-0 | isbn_ebook | 978-3-031-58171-7Series ISSN 0302-9743 Series E-ISSN 1611-3349 | issn_series | 0302-9743 | copyright | The Editor(s) (if applicable) and The Author(s), under exclusive license to Springer Nature Switzerl |
The information of publication is updating
书目名称Data Augmentation, Labelling, and Imperfections影响因子(影响力) 
书目名称Data Augmentation, Labelling, and Imperfections影响因子(影响力)学科排名 
书目名称Data Augmentation, Labelling, and Imperfections网络公开度 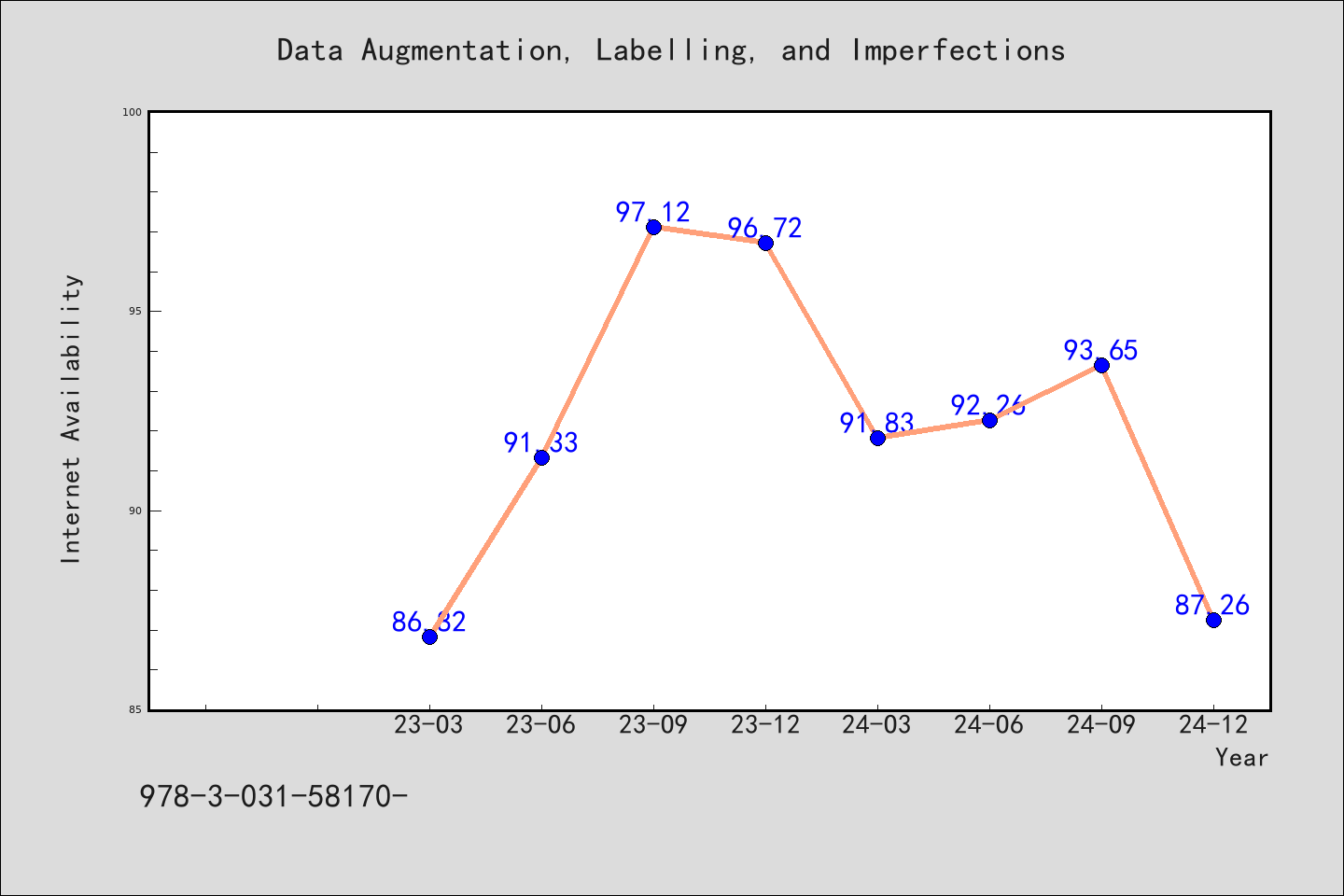
书目名称Data Augmentation, Labelling, and Imperfections网络公开度学科排名 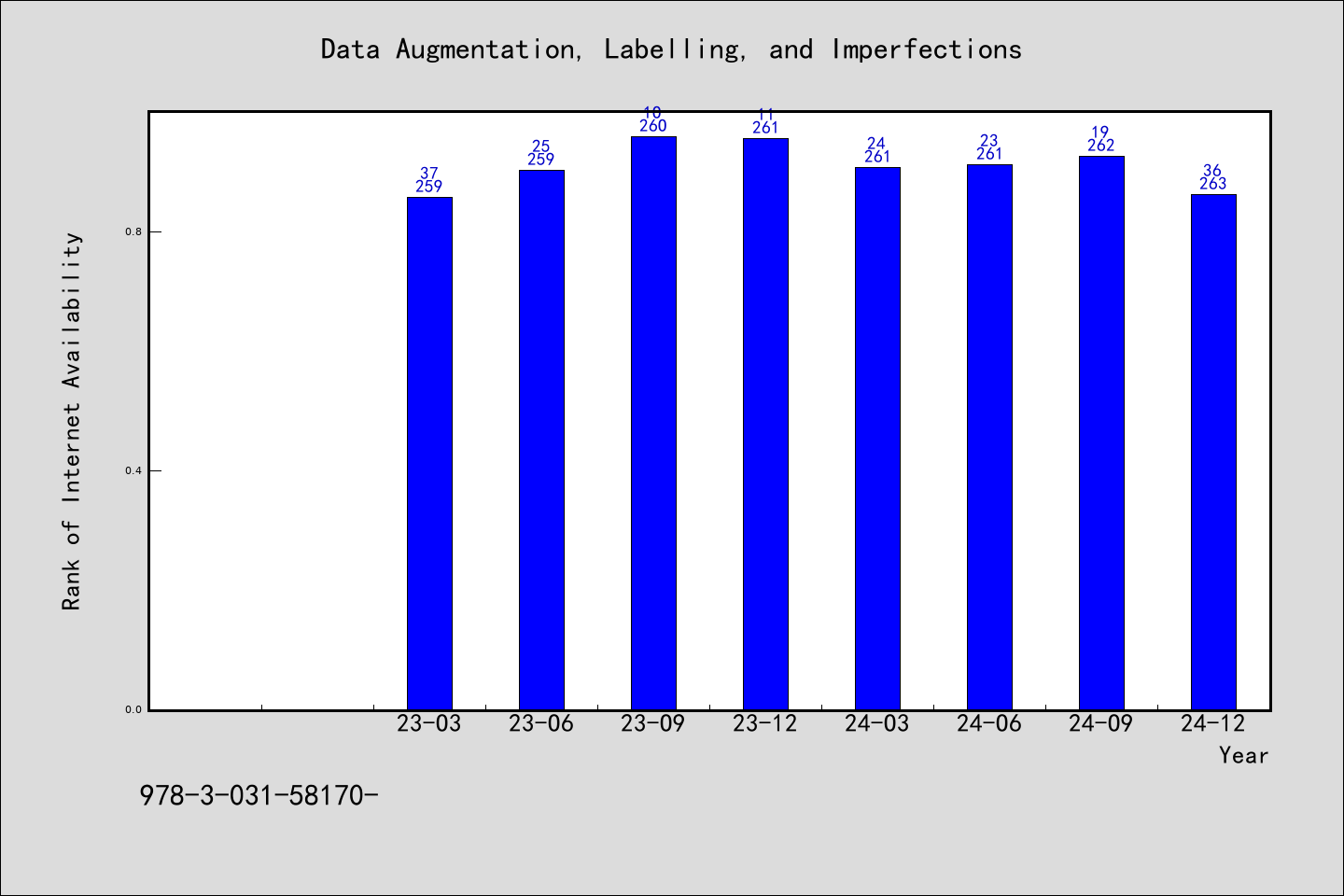
书目名称Data Augmentation, Labelling, and Imperfections被引频次 
书目名称Data Augmentation, Labelling, and Imperfections被引频次学科排名 
书目名称Data Augmentation, Labelling, and Imperfections年度引用 
书目名称Data Augmentation, Labelling, and Imperfections年度引用学科排名 
书目名称Data Augmentation, Labelling, and Imperfections读者反馈 
书目名称Data Augmentation, Labelling, and Imperfections读者反馈学科排名 
|
|
|