期刊全称 | Asymptotic Statistical Inference | 期刊简称 | A Basic Course Using | 影响因子2023 | Shailaja Deshmukh,Madhuri Kulkarni | 视频video | http://file.papertrans.cn/164/163829/163829.mp4 | 发行地址 | Presents fundamental concepts from asymptotic statistical inference theory, illustrated by R software.Contains numerous examples, conceptual and computational exercises based on R, and MCQs to clarify | 图书封面 | 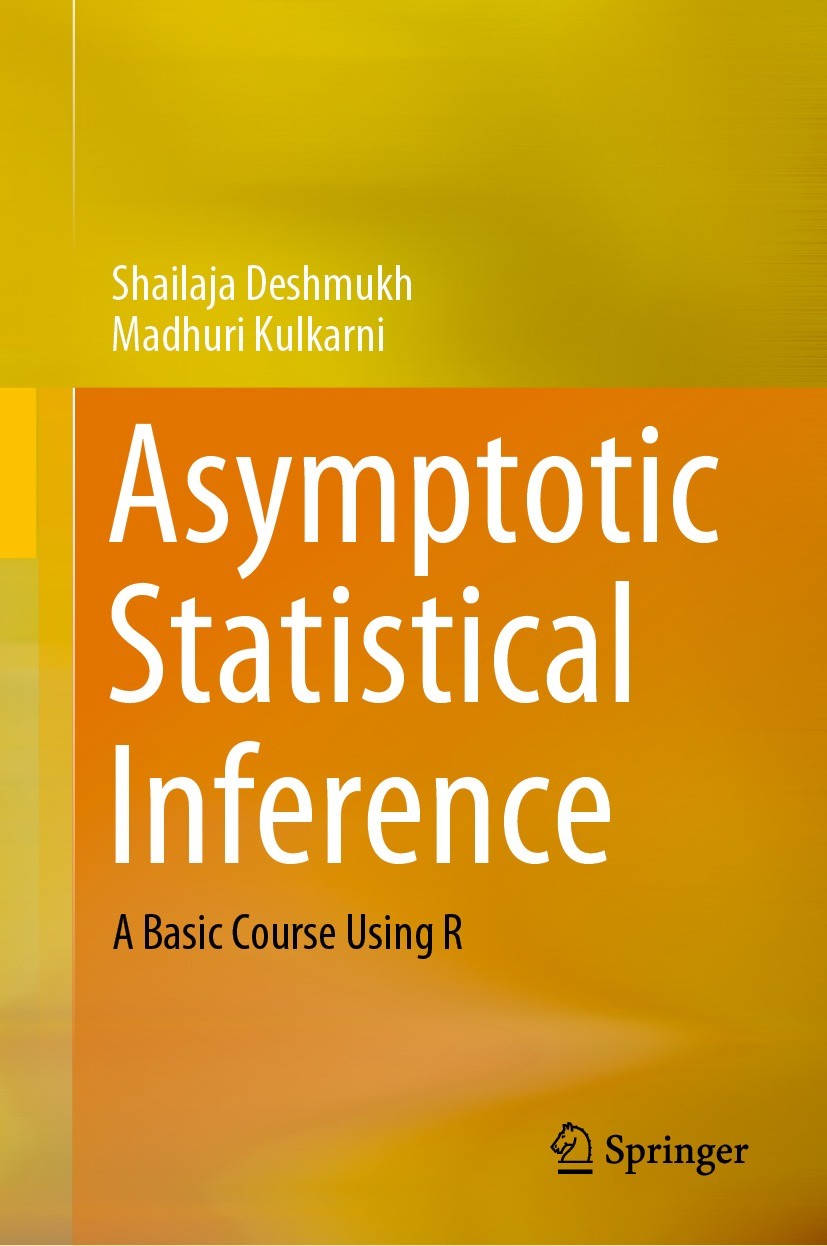 | 影响因子 | .The book presents the fundamental concepts from asymptotic statistical inference theory, elaborating on some basic large sample optimality properties of estimators and some test procedures. The most desirable property of consistency of an estimator and its large sample distribution, with suitable normalization, are discussed, the focus being on the consistent and asymptotically normal (CAN) estimators. It is shown that for the probability models belonging to an exponential family and a Cramer family, the maximum likelihood estimators of the indexing parameters are CAN. The book describes some large sample test procedures, in particular, the most frequently used likelihood ratio test procedure. Various applications of the likelihood ratio test procedure are addressed, when the underlying probability model is a multinomial distribution. These include tests for the goodness of fit and tests for contingency tables. The book also discusses a score test and Wald’s test, their relationship with the likelihood ratio test and Karl Pearson’s chi-square test. An important finding is that, while testing any hypothesis about the parameters of a multinomial distribution, a score test statistic | Pindex | Textbook 2021 |
The information of publication is updating
|
|