期刊全称 | Artificial Neural Networks and Machine Learning – ICANN 2020 | 期刊简称 | 29th International C | 影响因子2023 | Igor Farkaš,Paolo Masulli,Stefan Wermter | 视频video | | 学科分类 | Lecture Notes in Computer Science | 图书封面 | 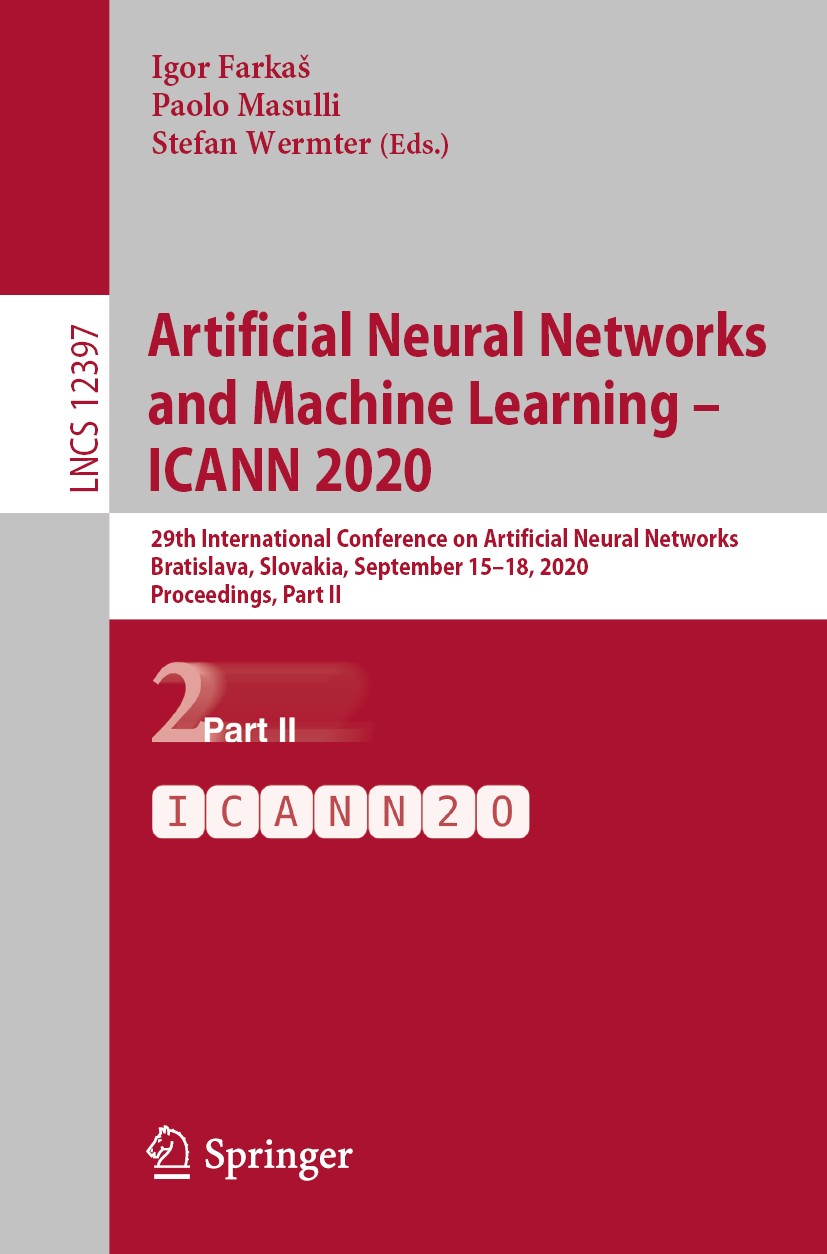 | 影响因子 | The proceedings set LNCS 12396 and 12397 constitute the proceedings of the 29th International Conference on Artificial Neural Networks, ICANN 2020, held in Bratislava, Slovakia, in September 2020.*.The total of 139 full papers presented in these proceedings was carefully reviewed and selected from 249 submissions. They were organized in 2 volumes focusing on topics such as adversarial machine learning, bioinformatics and biosignal analysis, cognitive models, neural network theory and information theoretic learning, and robotics and neural models of perception and action...*The conference was postponed to 2021 due to the COVID-19 pandemic.. | Pindex | Conference proceedings 2020 |
The information of publication is updating
|
|