期刊全称 | Artificial Intelligence in Medicine | 期刊简称 | 21st International C | 影响因子2023 | Jose M. Juarez,Mar Marcos,Allan Tucker | 视频video | | 学科分类 | Lecture Notes in Computer Science | 图书封面 | 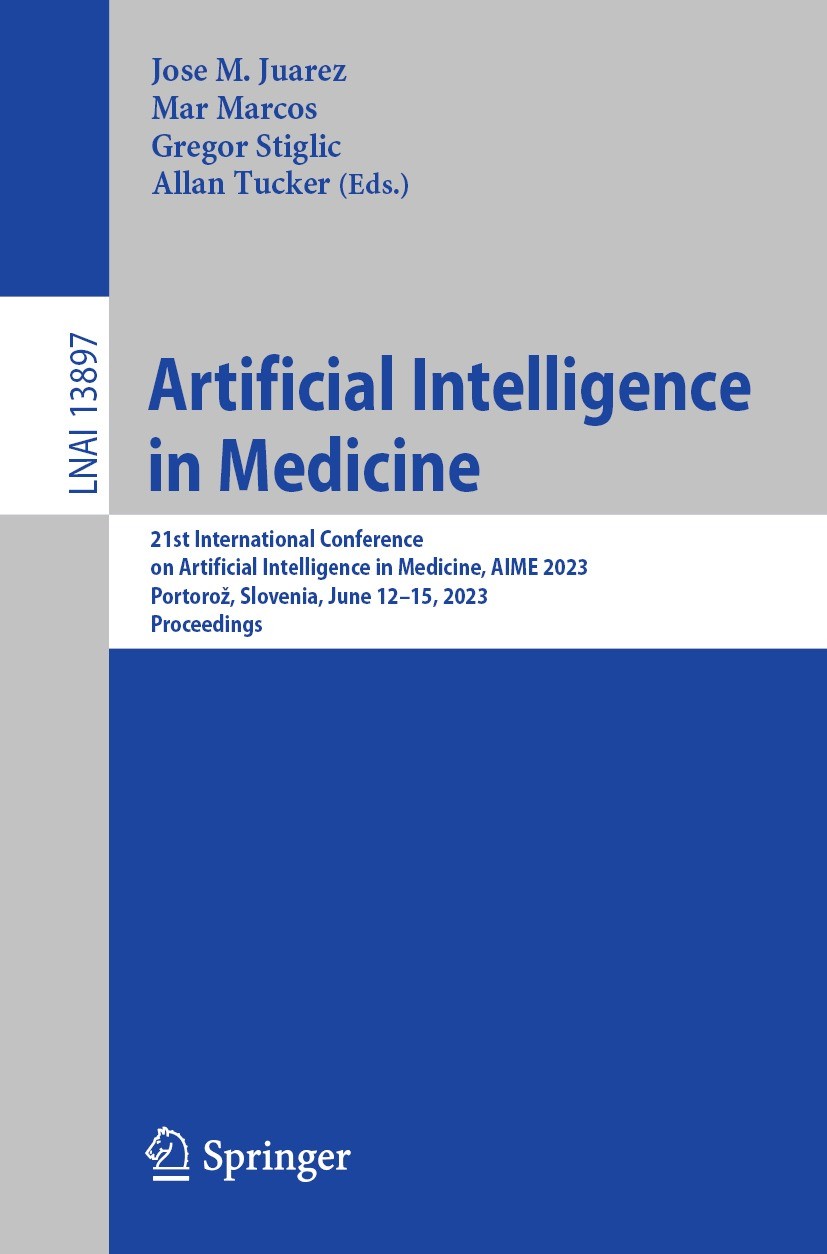 | 影响因子 | .This book constitutes the refereed proceedings of the 21st International Conference on Artificial Intelligence in Medicine, AIME 2023, held in Portoroz, Slovenia, in June12–15, 2023..The 23 full papers and 21 short papers presented together with 3 demonstration papers were selected from 108 submissions. The papers are grouped in topical sections on: machine learning and deep learning; explainability and transfer learning; natural language processing; image analysis and signal analysis; data analysis and statistical models; knowledge representation and decision support.. | Pindex | Conference proceedings 2023 |
The information of publication is updating
|
|