期刊全称 | Applied Deep Learning with TensorFlow 2 | 期刊简称 | Learn to Implement A | 影响因子2023 | Umberto Michelucci | 视频video | | 发行地址 | Covers Debugging and optimization of deep learning techniques with TensorFlow 2.0 and Python.Covers recent advances in autoencoders and multitask learning.Explains how to build models and deploy them | 图书封面 | 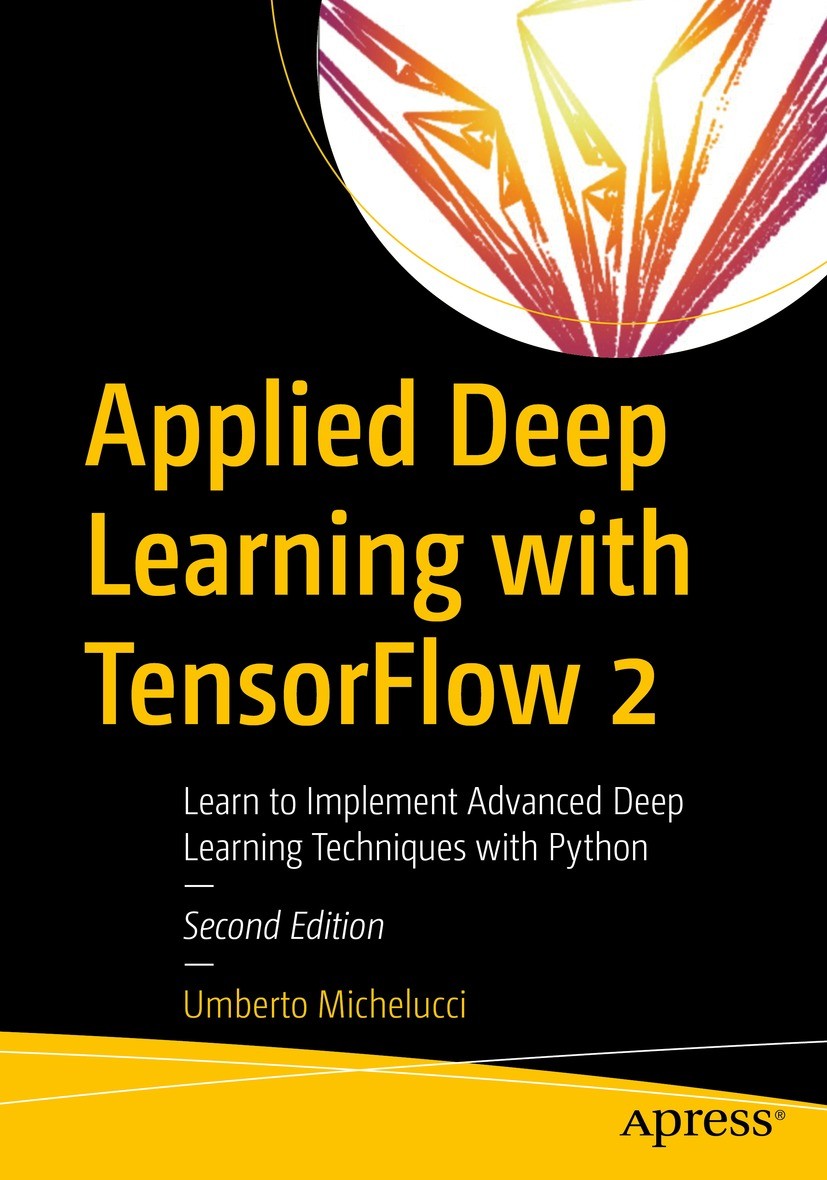 | 影响因子 | .Understand how neural networks work and learn how to implement them using TensorFlow 2.0 and Keras. This new edition focuses on the fundamental concepts and at the same time on practical aspects of implementing neural networks and deep learning for your research projects..This book is designed so that you can focus on the parts you are interested in. You will explore topics as regularization, optimizers, optimization, metric analysis, and hyper-parameter tuning. In addition, you will learn the fundamentals ideas behind autoencoders and generative adversarial networks..All the code presented in the book will be available in the form of Jupyter notebooks which would allow you to try out all examples and extend them in interesting ways. A companion online book is available with the complete code for all examples discussed in the book and additional material more related to TensorFlow and Keras. All the code will be available in Jupyter notebook format and can be openeddirectly in Google Colab (no need to install anything locally) or downloaded on your own machine and tested locally..You will: .• Understand the fundamental concepts of how neural networks work.• Learn the fundamental i | Pindex | Book 2022Latest edition |
The information of publication is updating
|
|