书目名称 | Unsupervised Feature Extraction Applied to Bioinformatics |
副标题 | A PCA Based and TD B |
编辑 | Y-h. Taguchi |
视频video | |
概述 | Allows readers to analyze data sets with small samples and many features.Provides a fast algorithm, based upon linear algebra, to analyze big data.Includes several applications to multi-view data anal |
丛书名称 | Unsupervised and Semi-Supervised Learning |
图书封面 | 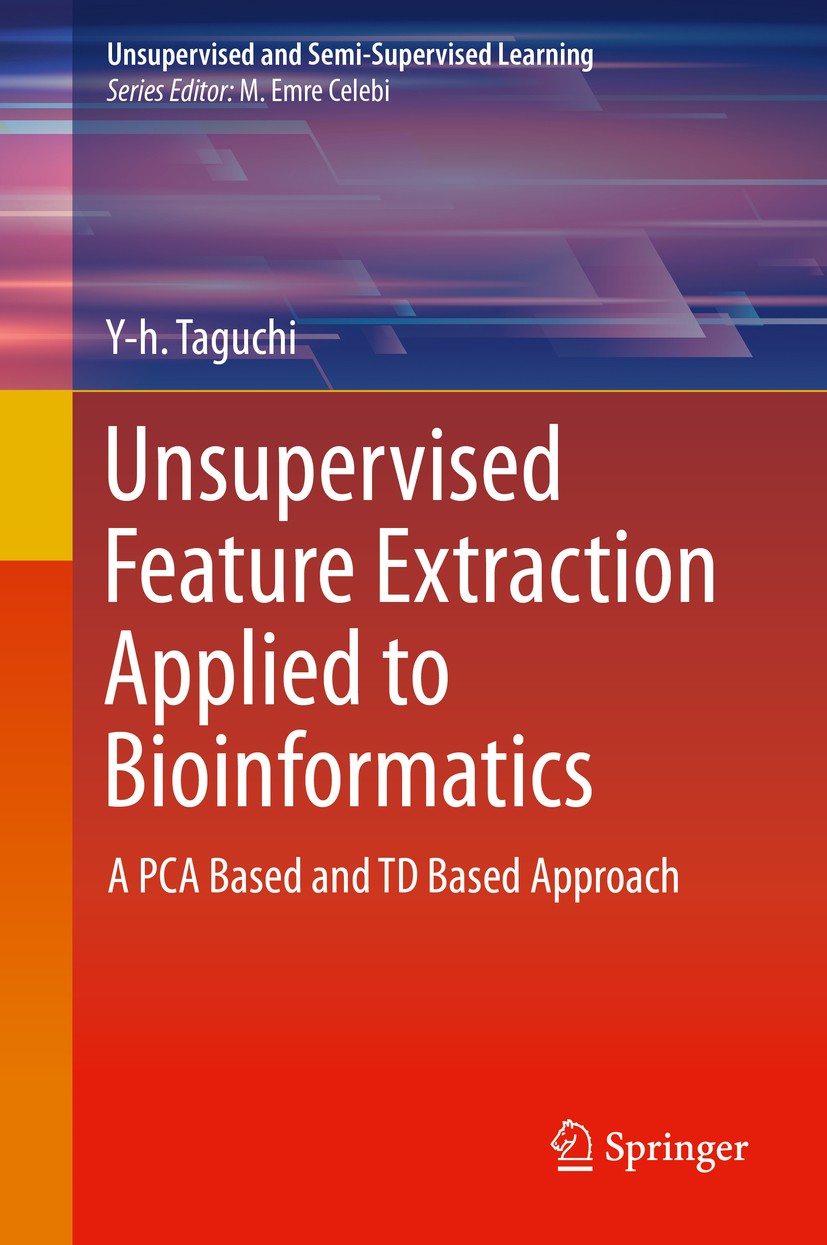 |
描述 | .This book proposes applications of tensor decomposition to unsupervised feature extraction and feature selection. The author posits that although supervised methods including deep learning have become popular, unsupervised methods have their own advantages. He argues that this is the case because unsupervised methods are easy to learn since tensor decomposition is a conventional linear methodology. This book starts from very basic linear algebra and reaches the cutting edge methodologies applied to difficult situations when there are many features (variables) while only small number of samples are available. The author includes advanced descriptions about tensor decomposition including Tucker decomposition using high order singular value decomposition as well as higher order orthogonal iteration, and train tenor decomposition. The author concludes by showing unsupervised methods and their application to a wide range of topics. .Allows readers to analyze data sets with small samples and many features;.Provides a fast algorithm, based upon linear algebra, to analyze big data;.Includes several applications to multi-view data analyses, with a focus on bioinformatics.. |
出版日期 | Book 20201st edition |
关键词 | Matrix factorization; Tensor decompositions; PCA based unsupervised FE; TD based unsupervised FE; PCA/TD |
版次 | 1 |
doi | https://doi.org/10.1007/978-3-030-22456-1 |
isbn_softcover | 978-3-030-22458-5 |
isbn_ebook | 978-3-030-22456-1Series ISSN 2522-848X Series E-ISSN 2522-8498 |
issn_series | 2522-848X |
copyright | Springer Nature Switzerland AG 2020 |