书目名称 | Uncertainty Modeling for Data Mining |
副标题 | A Label Semantics Ap |
编辑 | Zengchang Qin,Yongchuan Tang |
视频video | |
概述 | A new research direction of fuzzy set theory in data mining.One of the first monographs of studying the transparency of data mining models.Contains more than 60 figures and illustrations in order to e |
丛书名称 | Advanced Topics in Science and Technology in China |
图书封面 | 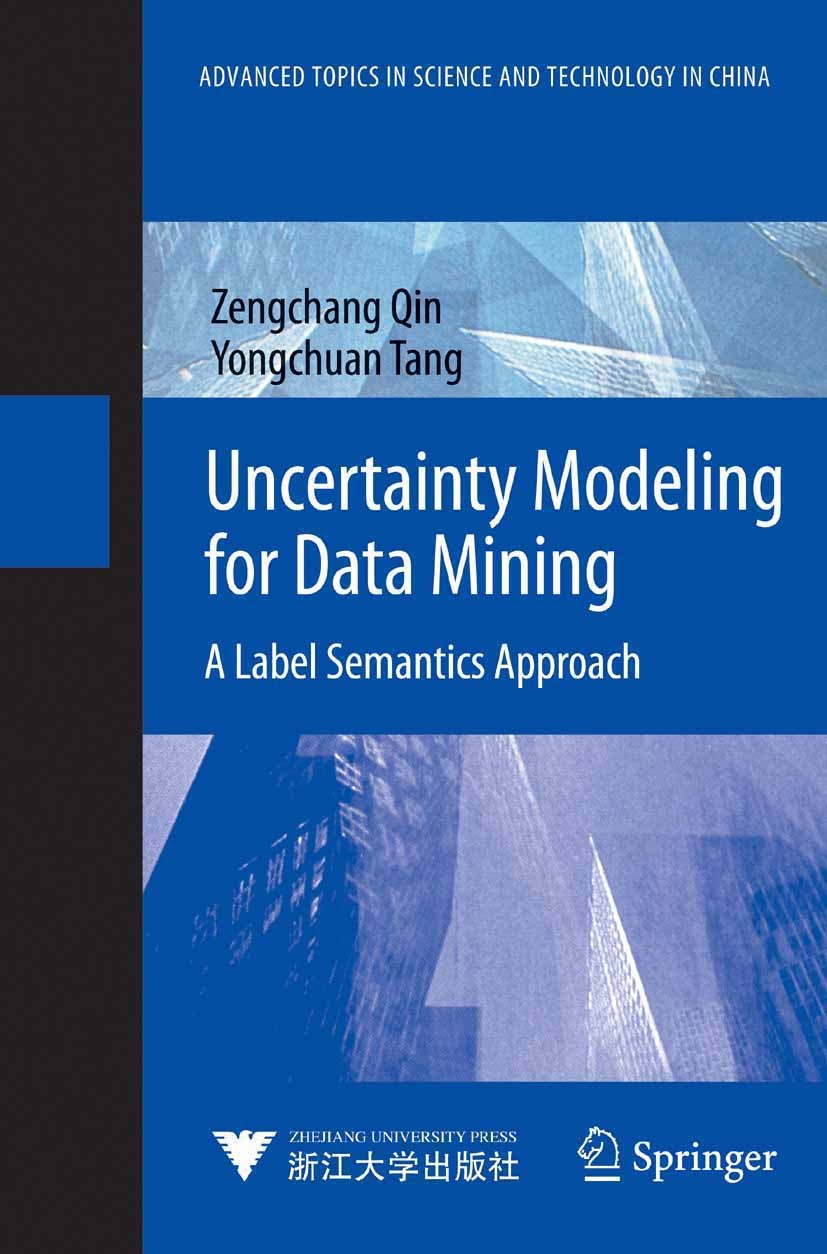 |
描述 | .Machine learning and data mining are inseparably connected with uncertainty. The observable data for learning is usually imprecise, incomplete or noisy. .Uncertainty Modeling for Data Mining: A Label Semantics Approach. introduces ‘label semantics‘, a fuzzy-logic-based theory for modeling uncertainty. Several new data mining algorithms based on label semantics are proposed and tested on real-world datasets. A prototype interpretation of label semantics and new prototype-based data mining algorithms are also discussed. This book offers a valuable resource for postgraduates, researchers and other professionals in the fields of data mining, fuzzy computing and uncertainty reasoning..Zengchang Qin. is an associate professor at the School of Automation Science and Electrical Engineering, Beihang University, China; .Yongchuan Tang. is an associate professor at the College of Computer Science, Zhejiang University, China.. |
出版日期 | Book 2014 |
关键词 | Computational Intelligence; Computational Intelligence; Data Mining; Data Mining; Fuzzy Logic; Fuzzy Logi |
版次 | 1 |
doi | https://doi.org/10.1007/978-3-642-41251-6 |
isbn_ebook | 978-3-642-41251-6Series ISSN 1995-6819 Series E-ISSN 1995-6827 |
issn_series | 1995-6819 |
copyright | The Editor(s) (if applicable) and The Author(s), under exclusive license to Springer-Verlag GmbH, DE |