书目名称 | Transfer Learning for Multiagent Reinforcement Learning Systems | 编辑 | Felipe Leno Silva,Anna Helena Reali Costa | 视频video | | 丛书名称 | Synthesis Lectures on Artificial Intelligence and Machine Learning | 图书封面 | 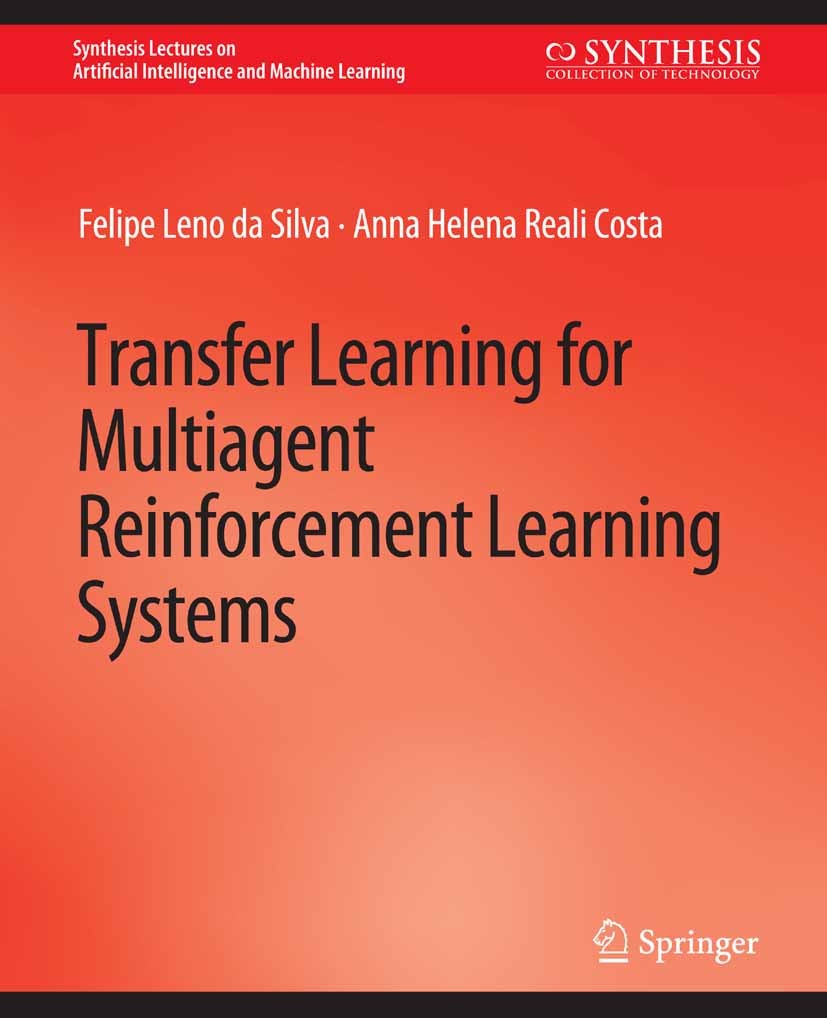 | 描述 | .Learning. to solve sequential decision-making tasks is difficult. Humans take years exploring the environment essentially in a random way until they are able to reason, solve difficult tasks, and collaborate with other humans towards a common goal. Artificial Intelligent agents are like humans in this aspect. Reinforcement Learning (RL) is a well-known technique to train autonomous agents through interactions with the environment. Unfortunately, the learning process has a high sample complexity to infer an effective actuation policy, especially when multiple agents are simultaneously actuating in the environment...However, previous knowledge can be leveraged to accelerate learning and enable solving harder tasks. In the same way humans build skills and reuse them by relating different tasks, RL agents might reuse knowledge from previously solved tasks and from the exchange of knowledge with other agents in the environment. In fact, virtually all of the most challenging tasks currently solved by RL rely on embedded knowledge reuse techniques, such as Imitation Learning, Learning from Demonstration, and Curriculum Learning...This book surveys the literature on knowledge reuse in mul | 出版日期 | Book 2021 | 版次 | 1 | doi | https://doi.org/10.1007/978-3-031-01591-5 | isbn_softcover | 978-3-031-00463-6 | isbn_ebook | 978-3-031-01591-5Series ISSN 1939-4608 Series E-ISSN 1939-4616 | issn_series | 1939-4608 | copyright | Springer Nature Switzerland AG 2021 |
The information of publication is updating
|
|