书目名称 | Shallow Learning vs. Deep Learning | 副标题 | A Practical Guide fo | 编辑 | Ömer Faruk Ertuğrul,Josep M Guerrero,Musa Yilmaz | 视频video | | 概述 | Compares and contrasts shallow learning and deep learning techniques, exploring their applications in various fields.Emphasizes real-world applications of machine learning, exploring the strengths and | 丛书名称 | The Springer Series in Applied Machine Learning | 图书封面 | 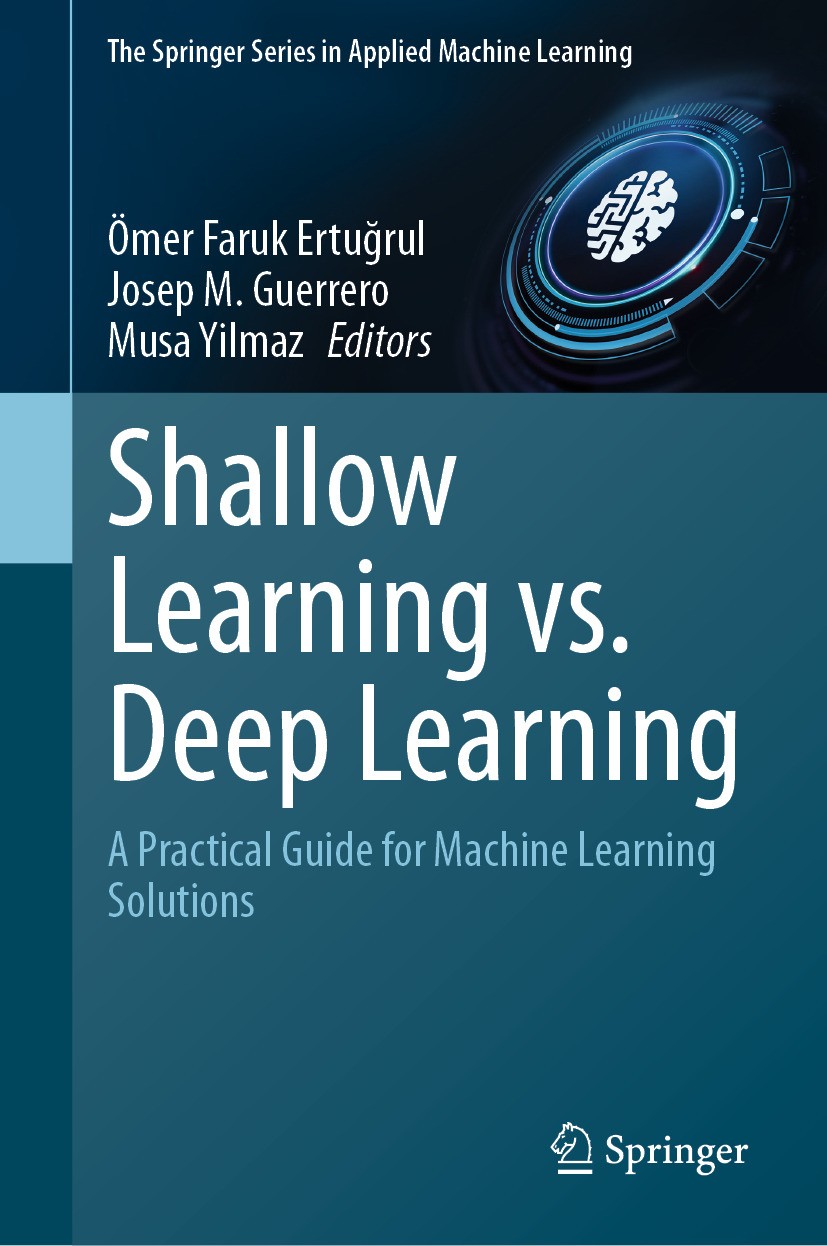 | 描述 | .This book explores the ongoing debate between shallow and deep learning in the field of machine learning. It provides a comprehensive survey of machine learning methods, from shallow learning to deep learning, and examines their applications across various domains. .Shallow Learning vs Deep Learning: A Practical Guide for Machine Learning Solutions. emphasizes that the choice of a machine learning approach should be informed by the specific characteristics of the dataset, the operational environment, and the unique requirements of each application, rather than being influenced by prevailing trends...In each chapter, the book delves into different application areas, such as engineering, real-world scenarios, social applications, image processing, biomedical applications, anomaly detection, natural language processing, speech recognition, recommendation systems, autonomous systems, and smart grid applications. By comparing and contrasting the effectiveness of shallow and deep learning in these areas, the book provides a framework for thoughtful selection and application of machine learning strategies. This guide is designed for researchers, practitioners, and students who seek to de | 出版日期 | Book 2024 | 关键词 | Artificial intelligence; Shallow Learning; Deep Learning; Machine Learning; Engineering applications; Con | 版次 | 1 | doi | https://doi.org/10.1007/978-3-031-69499-8 | isbn_softcover | 978-3-031-69501-8 | isbn_ebook | 978-3-031-69499-8Series ISSN 2520-1298 Series E-ISSN 2520-1301 | issn_series | 2520-1298 | copyright | The Editor(s) (if applicable) and The Author(s), under exclusive license to Springer Nature Switzerl |
The information of publication is updating
|
|