书目名称 | Statistical Field Theory for Neural Networks |
编辑 | Moritz Helias,David Dahmen |
视频video | |
概述 | Provides the first self-contained introduction to field theory for neuronal networks.Presents the main concepts from field theory that are relevant for network dynamics, including diagrammatic techniq |
丛书名称 | Lecture Notes in Physics |
图书封面 | 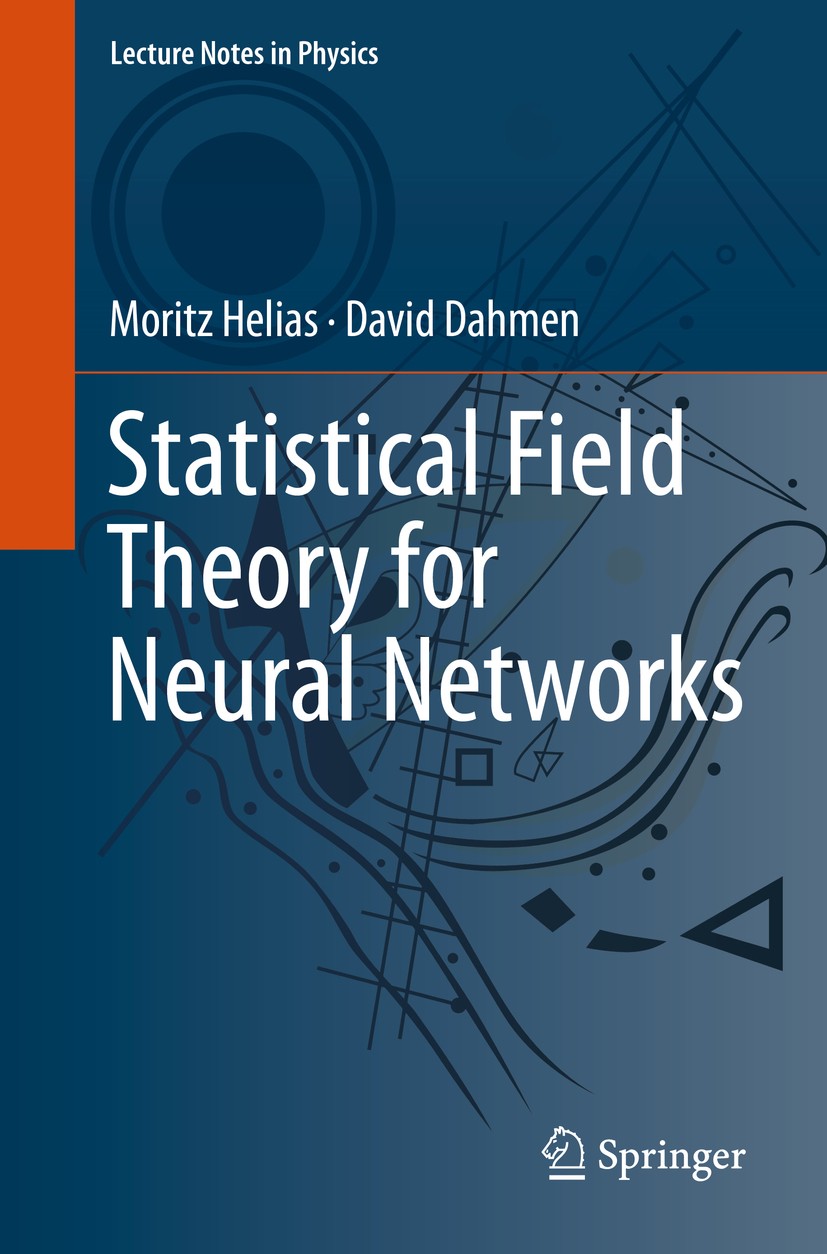 |
描述 | .This book presents a self-contained introduction to techniques from field theory applied to stochastic and collective dynamics in neuronal networks. These powerful analytical techniques, which are well established in other fields of physics, are the basis of current developments and offer solutions to pressing open problems in theoretical neuroscience and also machine learning. They enable a systematic and quantitative understanding of the dynamics in recurrent and stochastic neuronal networks. ..This book is intended for physicists, mathematicians, and computer scientists and it is designed for self-study by researchers who want to enter the field or as the main text for a one semester course at advanced undergraduate or graduate level. The theoretical concepts presented in this book are systematically developed from the very beginning, which only requires basic knowledge of analysis and linear algebra.. |
出版日期 | Book 2020 |
关键词 | Statistical physics; Neuronal networks; Dynamic mean-field theory; Diagrammatic techniques; Chaotic netw |
版次 | 1 |
doi | https://doi.org/10.1007/978-3-030-46444-8 |
isbn_softcover | 978-3-030-46443-1 |
isbn_ebook | 978-3-030-46444-8Series ISSN 0075-8450 Series E-ISSN 1616-6361 |
issn_series | 0075-8450 |
copyright | Springer Nature Switzerland AG 2020 |