书目名称 | State Space Modeling of Time Series | 编辑 | Masanao Aoki | 视频video | | 丛书名称 | Universitext | 图书封面 | 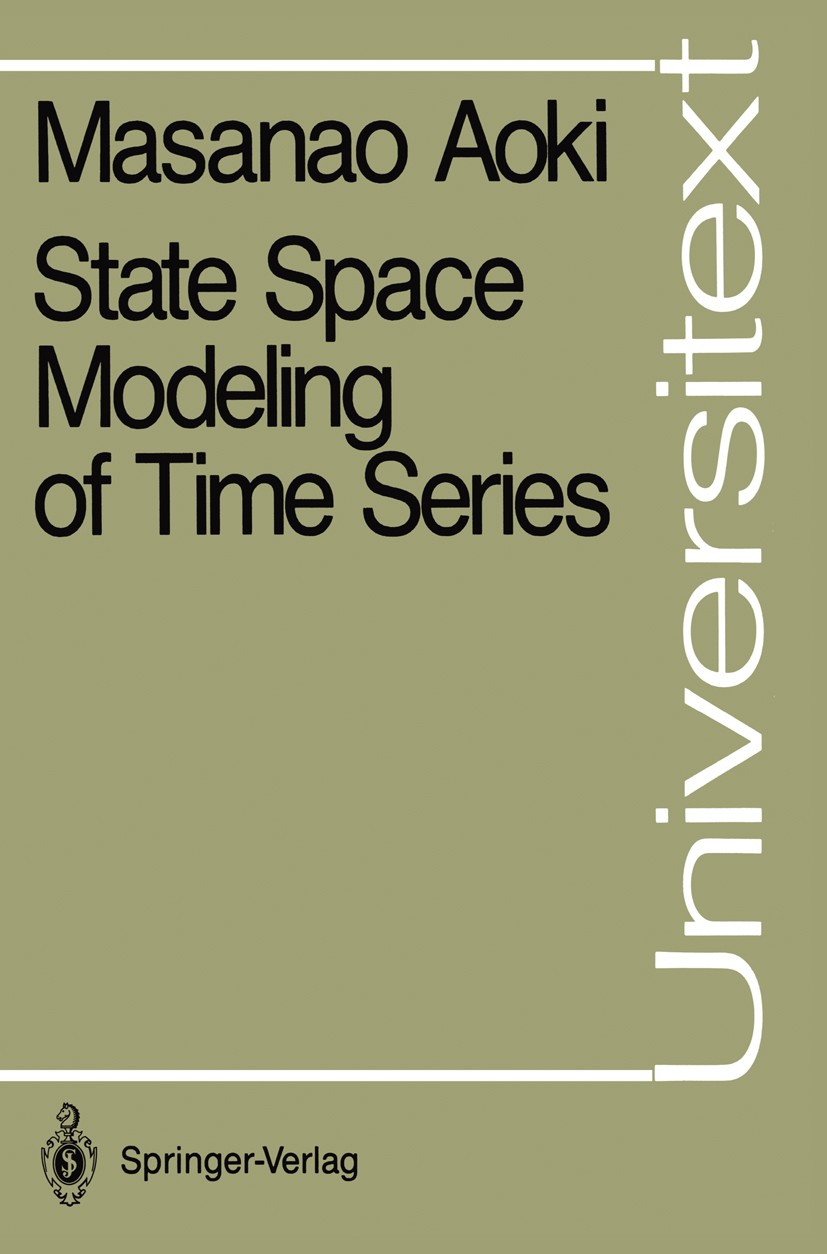 | 描述 | model‘s predictive capability? These are some of the questions that need to be answered in proposing any time series model construction method. This book addresses these questions in Part II. Briefly, the covariance matrices between past data and future realizations of time series are used to build a matrix called the Hankel matrix. Information needed for constructing models is extracted from the Hankel matrix. For example, its numerically determined rank will be the di mension of the state model. Thus the model dimension is determined by the data, after balancing several sources of error for such model construction. The covariance matrix of the model forecasting error vector is determined by solving a certain matrix Riccati equation. This matrix is also the covariance matrix of the innovation process which drives the model in generating model forecasts. In these model construction steps, a particular model representation, here referred to as balanced, is used extensively. This mode of model representation facilitates error analysis, such as assessing the error of using a lower dimensional model than that indicated by the rank of the Hankel matrix. The well-known Akaike‘s canonica | 出版日期 | Book 19871st edition | 关键词 | Instrumental variables; Instrumentalvariablen; Time series; Zeitreihe; algorithms; dynamic programming; fo | 版次 | 1 | doi | https://doi.org/10.1007/978-3-642-96985-0 | isbn_ebook | 978-3-642-96985-0Series ISSN 0172-5939 Series E-ISSN 2191-6675 | issn_series | 0172-5939 | copyright | Springer-Verlag Berlin Heidelberg 1987 |
The information of publication is updating
|
|