书目名称 | Similarity Search and Applications | 副标题 | 16th International C | 编辑 | Oscar Pedreira,Vladimir Estivill-Castro | 视频video | | 丛书名称 | Lecture Notes in Computer Science | 图书封面 | 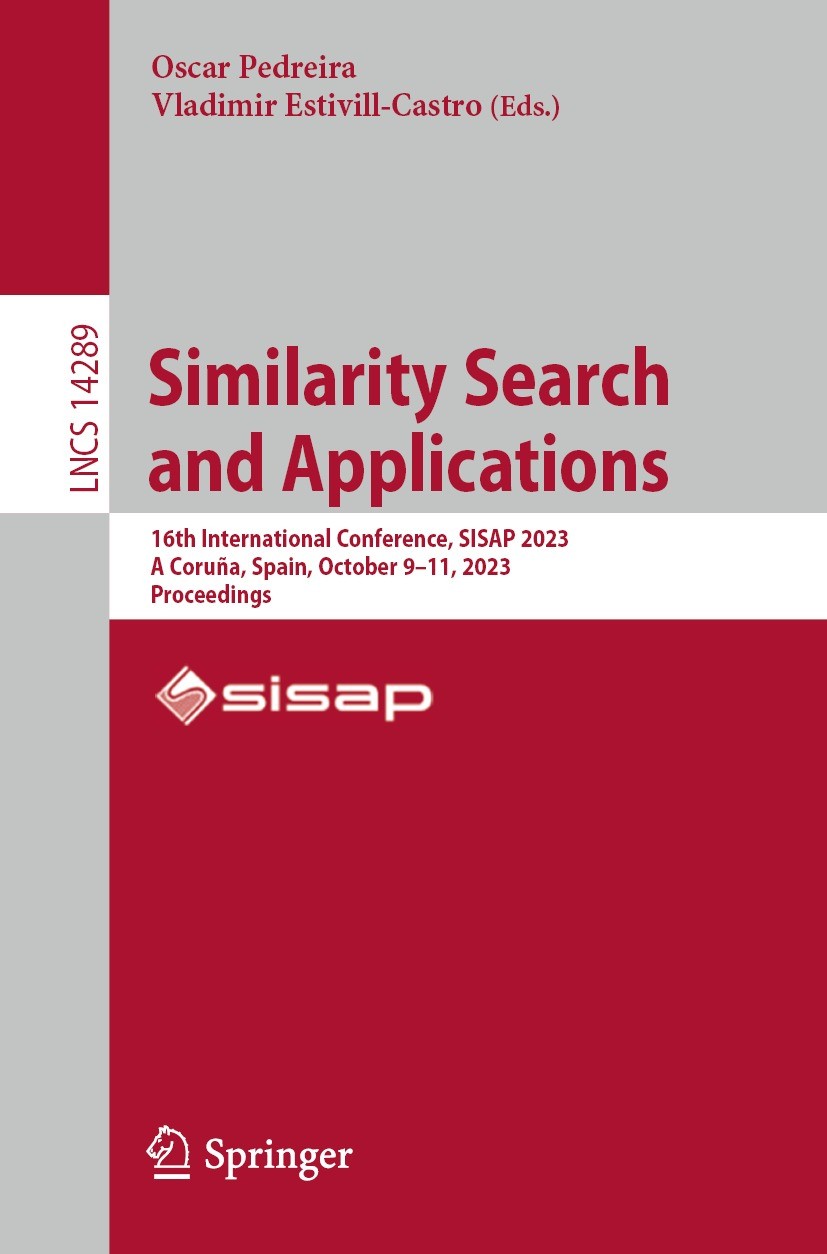 | 描述 | This book constitutes the refereed proceedings of the 16th International Conference on Similarity Search and Applications, SISAP 2023, held in A Coruña, Spain, during October 9–11, 2023..The 16 full papers and 4 short papers included in this book were carefully reviewed and selected from 33 submissions. They were organized in topical sections as follows: similarity queries, similarity measures, indexing and retrieval, data management, feature extraction, intrinsic dimensionality, efficient algorithms, similarity in machine learning and data mining.. | 出版日期 | Conference proceedings 2023 | 关键词 | clustering algorithms; computer networks; Computer systems; computer vision; data mining; databases; image | 版次 | 1 | doi | https://doi.org/10.1007/978-3-031-46994-7 | isbn_softcover | 978-3-031-46993-0 | isbn_ebook | 978-3-031-46994-7Series ISSN 0302-9743 Series E-ISSN 1611-3349 | issn_series | 0302-9743 | copyright | The Editor(s) (if applicable) and The Author(s), under exclusive license to Springer Nature Switzerl |
The information of publication is updating
|
|