书目名称 | Semi-Supervised Learning and Domain Adaptation in Natural Language Processing | 编辑 | Anders Søgaard | 视频video | | 丛书名称 | Synthesis Lectures on Human Language Technologies | 图书封面 | 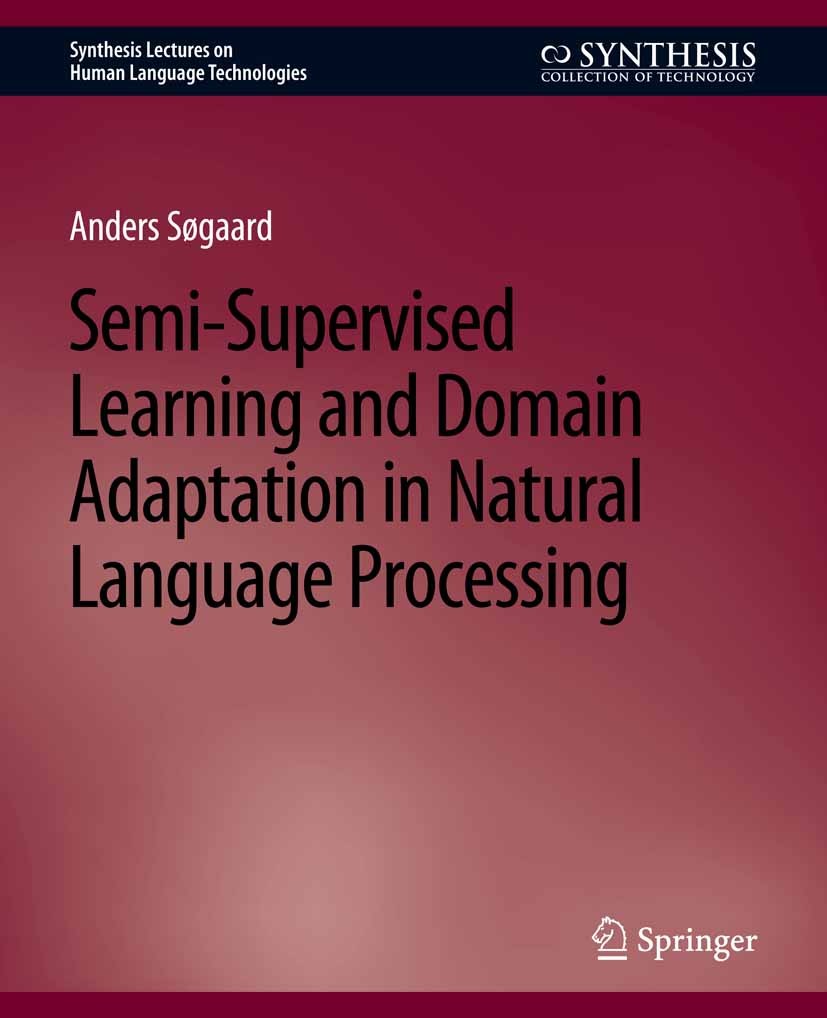 | 描述 | This book introduces basic supervised learning algorithms applicable to natural language processing (NLP) and shows how the performance of these algorithms can often be improved by exploiting the marginal distribution of large amounts of unlabeled data. One reason for that is data sparsity, i.e., the limited amounts of data we have available in NLP. However, in most real-world NLP applications our labeled data is also heavily biased. This book introduces extensions of supervised learning algorithms to cope with data sparsity and different kinds of sampling bias. This book is intended to be both readable by first-year students and interesting to the expert audience. My intention was to introduce what is necessary to appreciate the major challenges we face in contemporary NLP related to data sparsity and sampling bias, without wasting too much time on details about supervised learning algorithms or particular NLP applications. I use text classification, part-of-speech tagging, and dependency parsing as running examples, and limit myself to a small set of cardinal learning algorithms. I have worried less about theoretical guarantees ("this algorithm never does too badly") than about u | 出版日期 | Book 2013 | 版次 | 1 | doi | https://doi.org/10.1007/978-3-031-02149-7 | isbn_softcover | 978-3-031-01021-7 | isbn_ebook | 978-3-031-02149-7Series ISSN 1947-4040 Series E-ISSN 1947-4059 | issn_series | 1947-4040 | copyright | Springer Nature Switzerland AG 2013 |
The information of publication is updating
|
|