书目名称 | Sample Size Determination in Clinical Trials with Multiple Endpoints |
编辑 | Takashi Sozu,Tomoyuki Sugimoto,Scott R. Evans |
视频video | |
概述 | Reviews statistical issues in clinical trials with multiple endpoints.Describes methods for power and sample size calculations in clinical trials with multiple endpoints including recently developed a |
丛书名称 | SpringerBriefs in Statistics |
图书封面 | 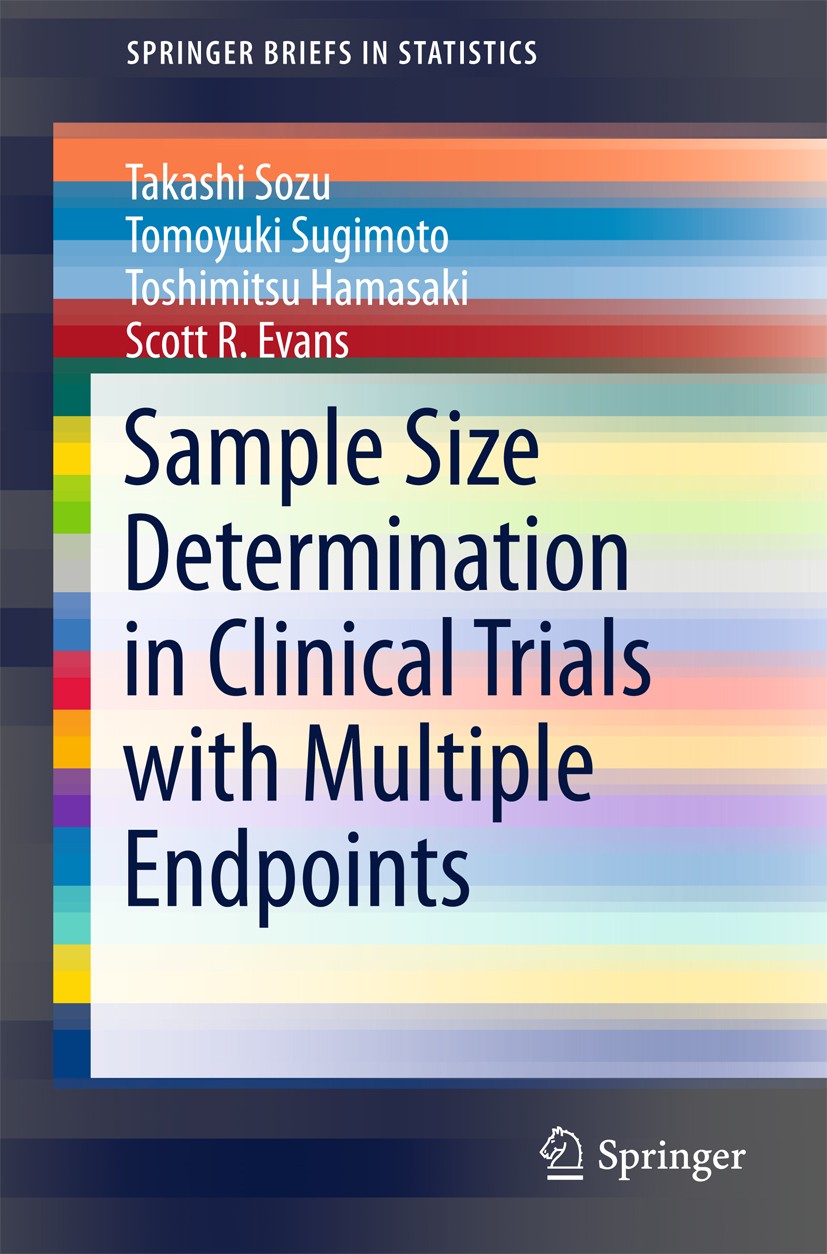 |
描述 | .This book integrates recent methodological developments for calculating the sample size and power in trials with more than one endpoint considered as multiple primary or co-primary, offering an important reference work for statisticians working in this area..The determination of sample size and the evaluation of power are fundamental and critical elements in the design of clinical trials. If the sample size is too small, important effects may go unnoticed; if the sample size is too large, it represents a waste of resources and unethically puts more participants at risk than necessary. Recently many clinical trials have been designed with more than one endpoint considered as multiple primary or co-primary, creating a need for new approaches to the design and analysis of these clinical trials. The book focuses on the evaluation of power and sample size determination when comparing the effects of two interventions in superiority clinical trials with multiple endpoints. Methods for sample size calculation in clinical trials where the alternative hypothesis is that there are effects on ALL endpoints are discussed in detail. The book also briefly examines trials designed with an alternat |
出版日期 | Book 2015 |
关键词 | Binary endpoints; Clinical tirals; Multiple endpoints; Power calculation; Sample size |
版次 | 1 |
doi | https://doi.org/10.1007/978-3-319-22005-5 |
isbn_softcover | 978-3-319-22004-8 |
isbn_ebook | 978-3-319-22005-5Series ISSN 2191-544X Series E-ISSN 2191-5458 |
issn_series | 2191-544X |
copyright | The Author(s) 2015 |