书目名称 | Quantum Machine Learning | 副标题 | Thinking and Explora | 编辑 | Claudio Conti | 视频video | | 概述 | Presents a new way of thinking about quantum physics by introducing machine learning from the beginning.Places coding at the forefront, with plenty of open-source examples.Shows how neural networks ca | 丛书名称 | Quantum Science and Technology | 图书封面 | 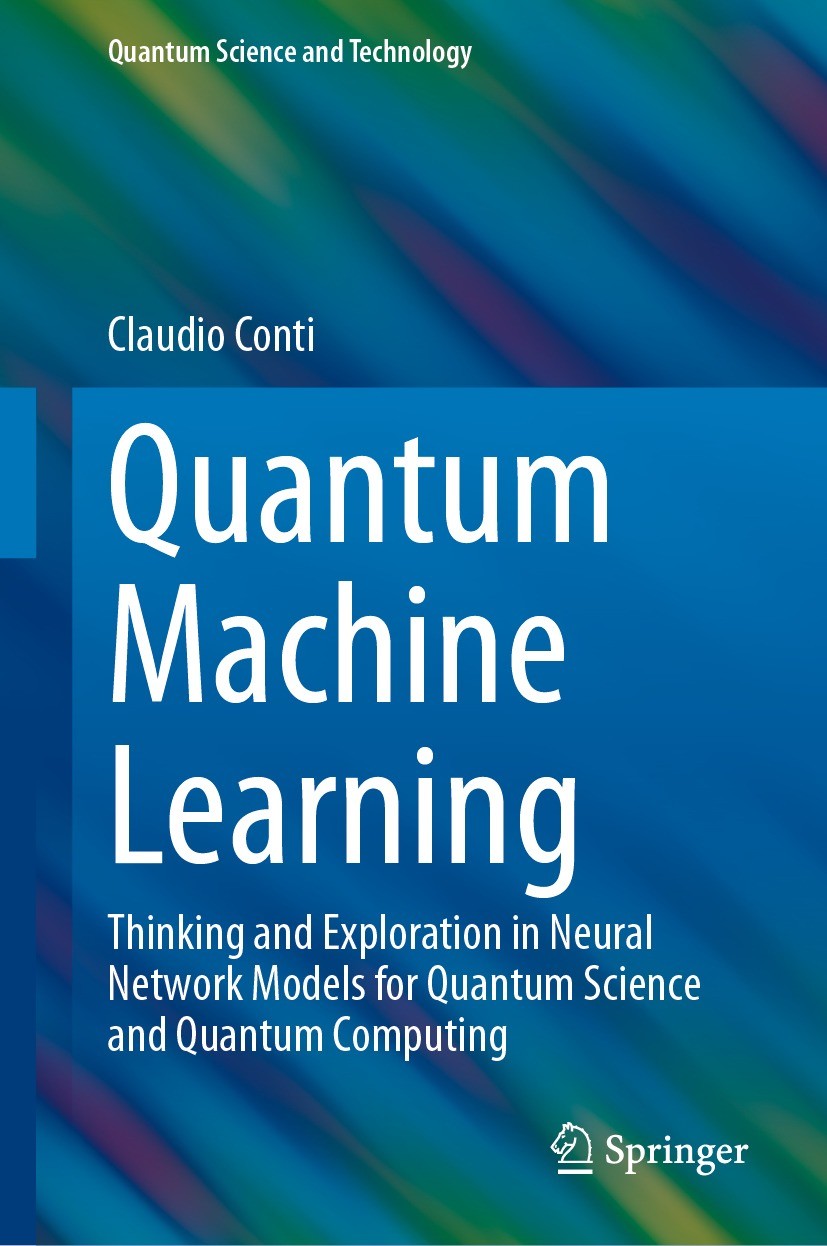 | 描述 | This book presents a new way of thinking about quantum mechanics and machine learning by merging the two. Quantum mechanics and machine learning may seem theoretically disparate, but their link becomes clear through the density matrix operator which can be readily approximated by neural network models, permitting a formulation of quantum physics in which physical observables can be computed via neural networks. As well as demonstrating the natural affinity of quantum physics and machine learning, this viewpoint opens rich possibilities in terms of computation, efficient hardware, and scalability. One can also obtain trainable models to optimize applications and fine-tune theories, such as approximation of the ground state in many body systems, and boosting quantum circuits’ performance. The book begins with the introduction of programming tools and basic concepts of machine learning, with necessary background material from quantum mechanics and quantum information also provided. This enables the basic building blocks, neural network models for vacuum states, to be introduced. The highlights that follow include: non-classical state representations, with squeezers and beam splitters | 出版日期 | Book 2024 | 关键词 | data-driven quantum physics; neural networks for quantum mechanics; boson sampling; machine learning in | 版次 | 1 | doi | https://doi.org/10.1007/978-3-031-44226-1 | isbn_softcover | 978-3-031-44228-5 | isbn_ebook | 978-3-031-44226-1Series ISSN 2364-9054 Series E-ISSN 2364-9062 | issn_series | 2364-9054 | copyright | The Editor(s) (if applicable) and The Author(s), under exclusive license to Springer Nature Switzerl |
The information of publication is updating
|
|