书目名称 | Provenance in Data Science | 副标题 | From Data Models to | 编辑 | Leslie F. Sikos,Oshani W. Seneviratne,Deborah L. M | 视频video | | 概述 | Presents a collection of provenance techniques and state-of-the-art metadata-enhanced, provenance-aware, knowledge graph-based representations to be used for information processing, management, aggreg | 丛书名称 | Advanced Information and Knowledge Processing | 图书封面 | 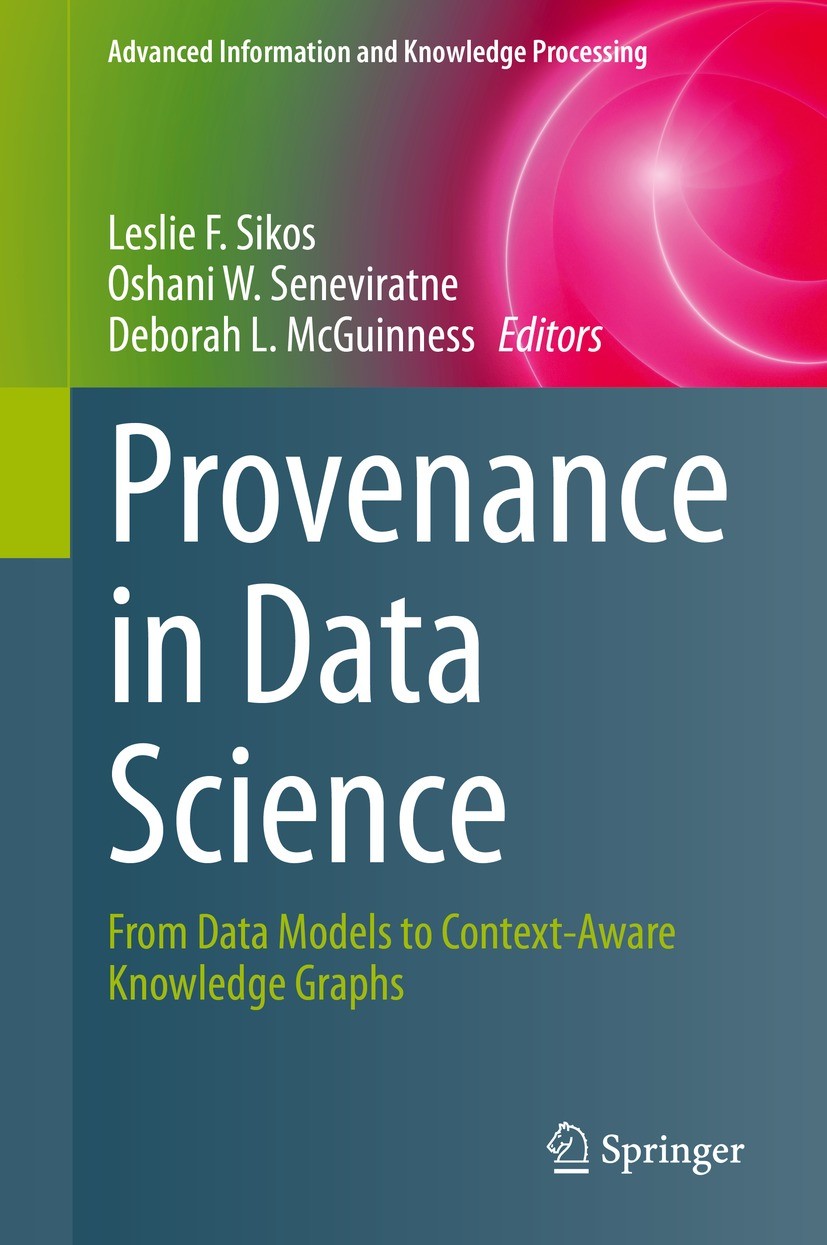 | 描述 | RDF-based knowledge graphs require additional formalisms to be fully context-aware, which is presented in this book. This book also provides a collection of provenance techniques and state-of-the-art metadata-enhanced, provenance-aware, knowledge graph-based representations across multiple application domains, in order to demonstrate how to combine graph-based data models and provenance representations. This is important to make statements authoritative, verifiable, and reproducible, such as in biomedical, pharmaceutical, and cybersecurity applications, where the data source and generator can be just as important as the data itself.. .Capturing provenance is critical to ensure sound experimental results and rigorously designed research studies for patient and drug safety, pathology reports, and medical evidence generation. Similarly, provenance is needed for cyberthreat intelligence dashboards and attack mapsthat aggregate and/or fuse heterogeneous data from disparate data sources to differentiate between unimportant online events and dangerous cyberattacks, which is demonstrated in this book. Without provenance, data reliability and trustworthiness might be limited, c | 出版日期 | Book 2021 | 关键词 | Knowledge graph; Contextualized Knowledge graph; Data science; Data provenance; Provenance ontology; Scie | 版次 | 1 | doi | https://doi.org/10.1007/978-3-030-67681-0 | isbn_softcover | 978-3-030-67683-4 | isbn_ebook | 978-3-030-67681-0Series ISSN 1610-3947 Series E-ISSN 2197-8441 | issn_series | 1610-3947 | copyright | Springer Nature Switzerland AG 2021 |
The information of publication is updating
|
|