书目名称 | Outlier Detection for Temporal Data | 编辑 | Manish Gupta,Jing Gao,Jiawei Han | 视频video | | 丛书名称 | Synthesis Lectures on Data Mining and Knowledge Discovery | 图书封面 | 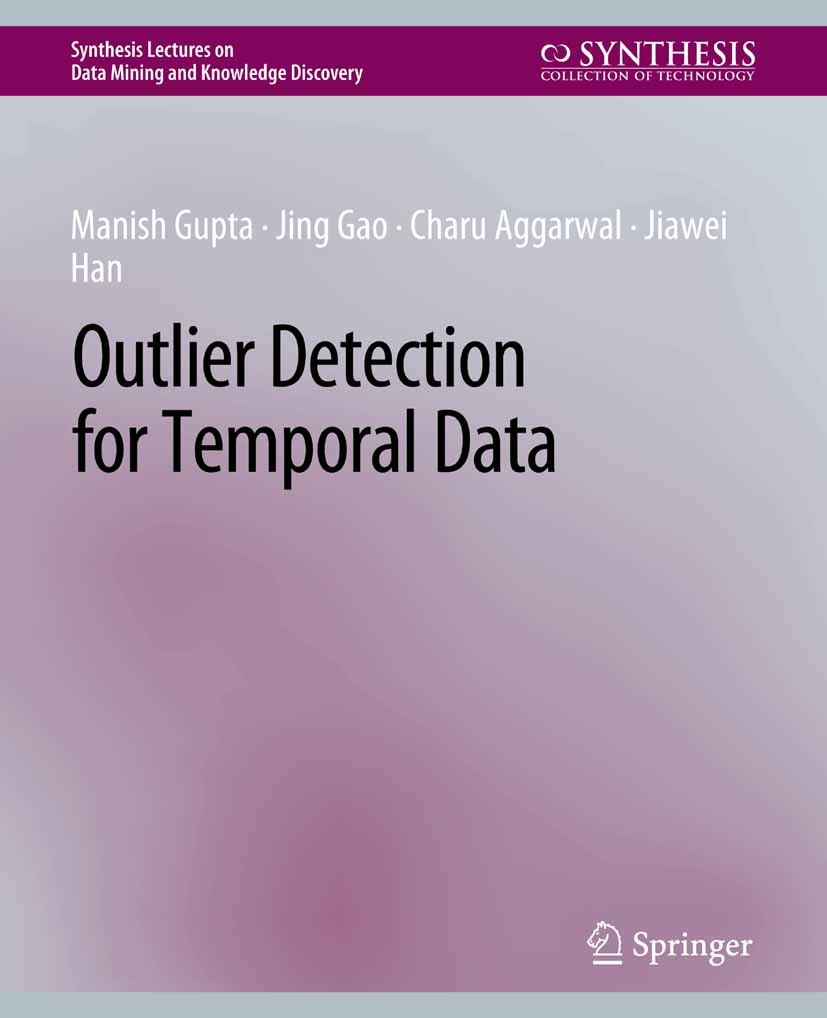 | 描述 | Outlier (or anomaly) detection is a very broad field which has been studied in the context of a large number of research areas like statistics, data mining, sensor networks, environmental science, distributed systems, spatio-temporal mining, etc. Initial research in outlier detection focused on time series-based outliers (in statistics). Since then, outlier detection has been studied on a large variety of data types including high-dimensional data, uncertain data, stream data, network data, time series data, spatial data, and spatio-temporal data. While there have been many tutorials and surveys for general outlier detection, we focus on outlier detection for temporal data in this book. A large number of applications generate temporal datasets. For example, in our everyday life, various kinds of records like credit, personnel, financial, judicial, medical, etc., are all temporal. This stresses the need for an organized and detailed study of outliers with respect to such temporal data.In the past decade, there has been a lot of research on various forms of temporal data including consecutive data snapshots, series of data snapshots and data streams. Besides the initial work on time | 出版日期 | Book 2014 | 版次 | 1 | doi | https://doi.org/10.1007/978-3-031-01905-0 | isbn_softcover | 978-3-031-00777-4 | isbn_ebook | 978-3-031-01905-0Series ISSN 2151-0067 Series E-ISSN 2151-0075 | issn_series | 2151-0067 | copyright | Springer Nature Switzerland AG 2014 |
The information of publication is updating
|
|