书目名称 | Online Machine Learning |
副标题 | A Practical Guide wi |
编辑 | Eva Bartz,Thomas Bartz-Beielstein |
视频video | |
概述 | Presents systematic comparison of OML and BML in terms of performance, time and memory requirements.Explains how OML can be customized by hyperparameter tuning.Accompanied with continuously-updated co |
丛书名称 | Machine Learning: Foundations, Methodologies, and Applications |
图书封面 | 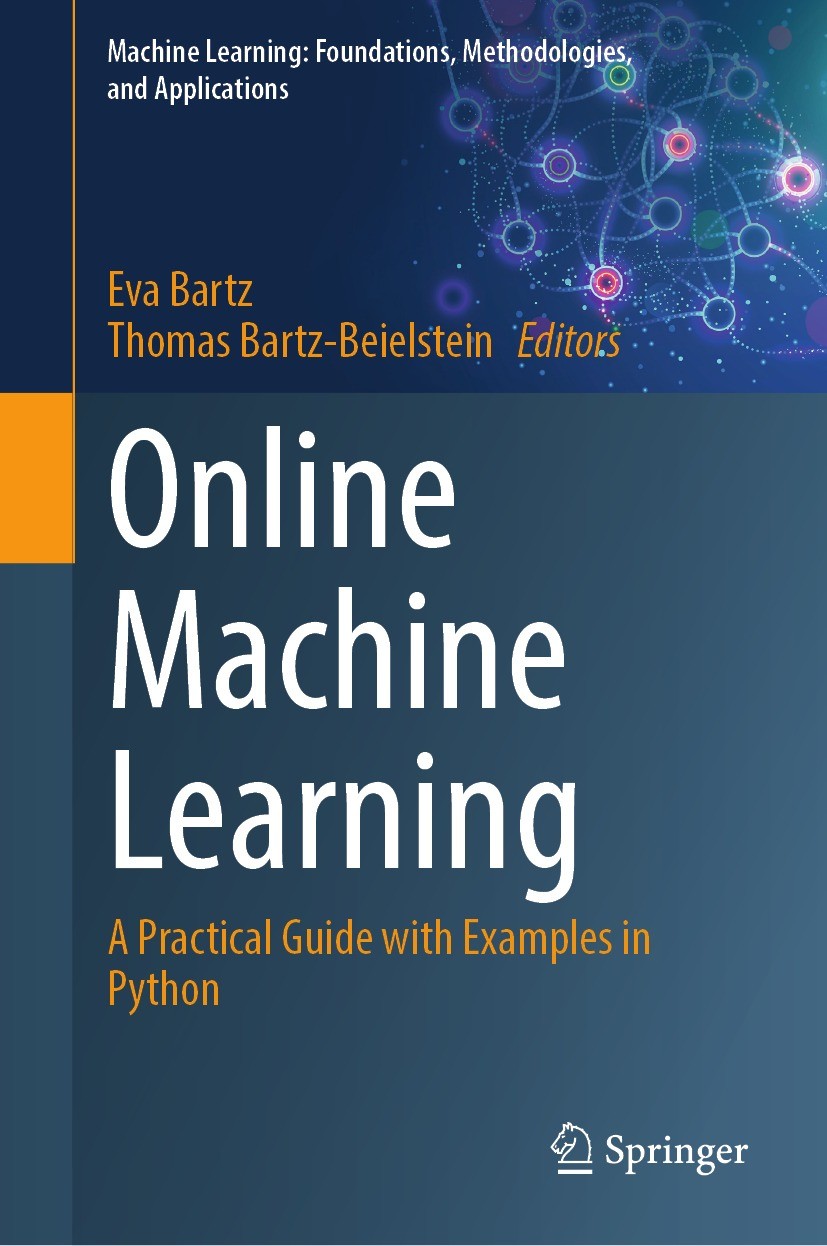 |
描述 | . .This book deals with the exciting, seminal topic of Online Machine Learning (OML). The content is divided into three parts: the first part looks in detail at the theoretical foundations of OML, comparing it to Batch Machine Learning (BML) and discussing what criteria should be developed for a meaningful comparison. The second part provides practical considerations, and the third part substantiates them with concrete practical applications...The book is equally suitable as a reference manual for experts dealing with OML, as a textbook for beginners who want to deal with OML, and as a scientific publication for scientists dealing with OML since it reflects the latest state of research. But it can also serve as quasi OML consulting since decision-makers and practitioners can use the explanations to tailor OML to their needs and use it for their application and ask whether the benefits of OML might outweigh the costs...OML will soon become practical; it is worthwhile to get involved with it now. This book already presents some tools that will facilitate the practice of OML in the future. A promising breakthrough is expected because practice shows that due to the large amounts of dat |
出版日期 | Book 2024 |
关键词 | Online Machine Learning; Machine Learning; Artificial Intelligence; Drift Detection; Supervised Learning |
版次 | 1 |
doi | https://doi.org/10.1007/978-981-99-7007-0 |
isbn_softcover | 978-981-99-7009-4 |
isbn_ebook | 978-981-99-7007-0Series ISSN 2730-9908 Series E-ISSN 2730-9916 |
issn_series | 2730-9908 |
copyright | The Editor(s) (if applicable) and The Author(s), under exclusive license to Springer Nature Singapor |