书目名称 | On Spatio-Temporal Data Modelling and Uncertainty Quantification Using Machine Learning and Informat |
编辑 | Fabian Guignard |
视频video | |
概述 | Follows a comprehensive end-to-end data analysis workflow.Covers concrete applications in environmental modelling and renewable energy assessement.Benefits environmental analysts and researchers worki |
丛书名称 | Springer Theses |
图书封面 | 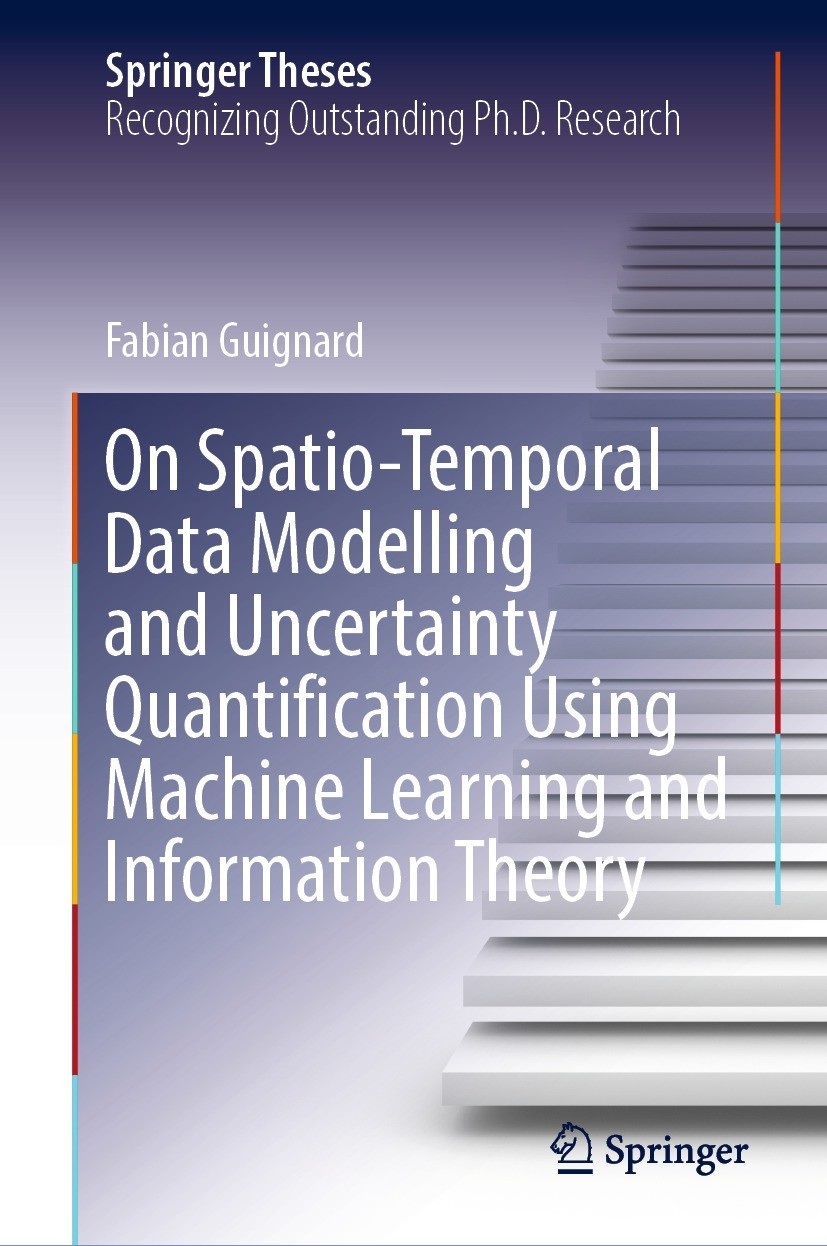 |
描述 | .The gathering and storage of data indexed in space and time are experiencing unprecedented growth, demanding for advanced and adapted tools to analyse them. This thesis deals with the exploration and modelling of complex high-frequency and non-stationary spatio-temporal data. It proposes an efficient framework in modelling with machine learning algorithms spatio-temporal fields measured on irregular monitoring networks, accounting for high dimensional input space and large data sets. The uncertainty quantification is enabled by specifying this framework with the extreme learning machine, a particular type of artificial neural network for which analytical results, variance estimation and confidence intervals are developed. Particular attention is also paid to a highly versatile exploratory data analysis tool based on information theory, the Fisher-Shannon analysis, which can be used to assess the complexity of distributional properties of temporal, spatial and spatio-temporal data sets. Examples of the proposed methodologies are concentrated on data from environmental sciences, with an emphasis on wind speed modelling in complex mountainous terrain and the resulting renewable energ |
出版日期 | Book 2022 |
关键词 | Machine Learning; Deep Learning; Uncertainty Quantification; Model Variance; Artificial Neural Network; S |
版次 | 1 |
doi | https://doi.org/10.1007/978-3-030-95231-0 |
isbn_softcover | 978-3-030-95233-4 |
isbn_ebook | 978-3-030-95231-0Series ISSN 2190-5053 Series E-ISSN 2190-5061 |
issn_series | 2190-5053 |
copyright | The Editor(s) (if applicable) and The Author(s), under exclusive license to Springer Nature Switzerl |