书目名称 | Non-negative Matrix Factorization Techniques |
副标题 | Advances in Theory a |
编辑 | Ganesh R. Naik |
视频video | |
概述 | Covers the latest cutting edge topics on NMF and emphasis on open problems on NMF.Balance on both theory and applications with examples.Offers in-depth analysis of NMF topics simply not covered elsewh |
丛书名称 | Signals and Communication Technology |
图书封面 | 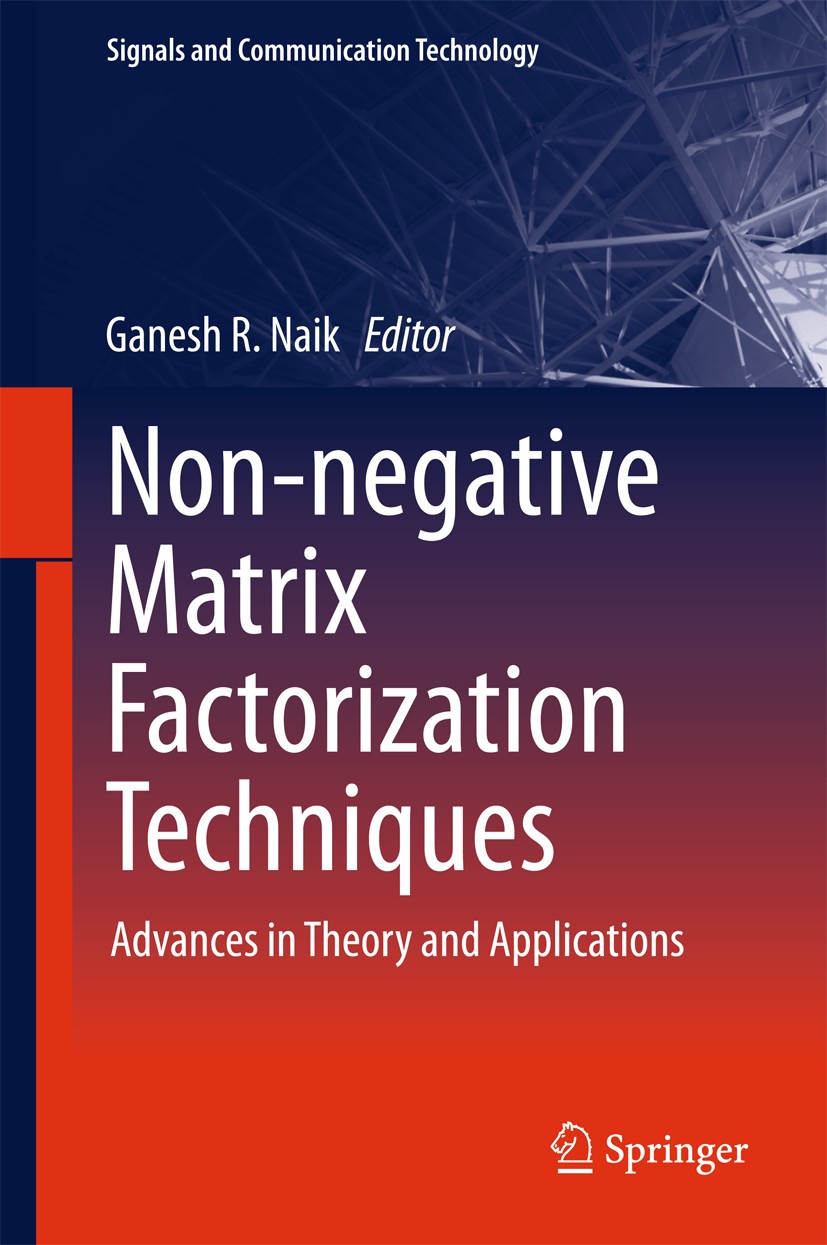 |
描述 | .This book collects new results, concepts and further developments of NMF. The open problems discussed include, e.g. in bioinformatics: NMF and its extensions applied to gene expression, sequence analysis, the functional characterization of genes, clustering and text mining etc. The research results previously scattered in different scientific journals and conference proceedings are methodically collected and presented in a unified form. While readers can read the book chapters sequentially, each chapter is also self-contained. This book can be a good reference work for researchers and engineers interested in NMF, and can also be used as a handbook for students and professionals seeking to gain a better understanding of the latest applications of NMF.. |
出版日期 | Book 2016 |
关键词 | Blind Source Separation; Multi-layer NMF; Non-negative Matrix Factorisation (NMF); Pattern Recognition; |
版次 | 1 |
doi | https://doi.org/10.1007/978-3-662-48331-2 |
isbn_softcover | 978-3-662-51700-0 |
isbn_ebook | 978-3-662-48331-2Series ISSN 1860-4862 Series E-ISSN 1860-4870 |
issn_series | 1860-4862 |
copyright | Springer-Verlag Berlin Heidelberg 2016 |