书目名称 | Neural Information Processing | 副标题 | 25th International C | 编辑 | Long Cheng,Andrew Chi Sing Leung,Seiichi Ozawa | 视频video | | 丛书名称 | Lecture Notes in Computer Science | 图书封面 | 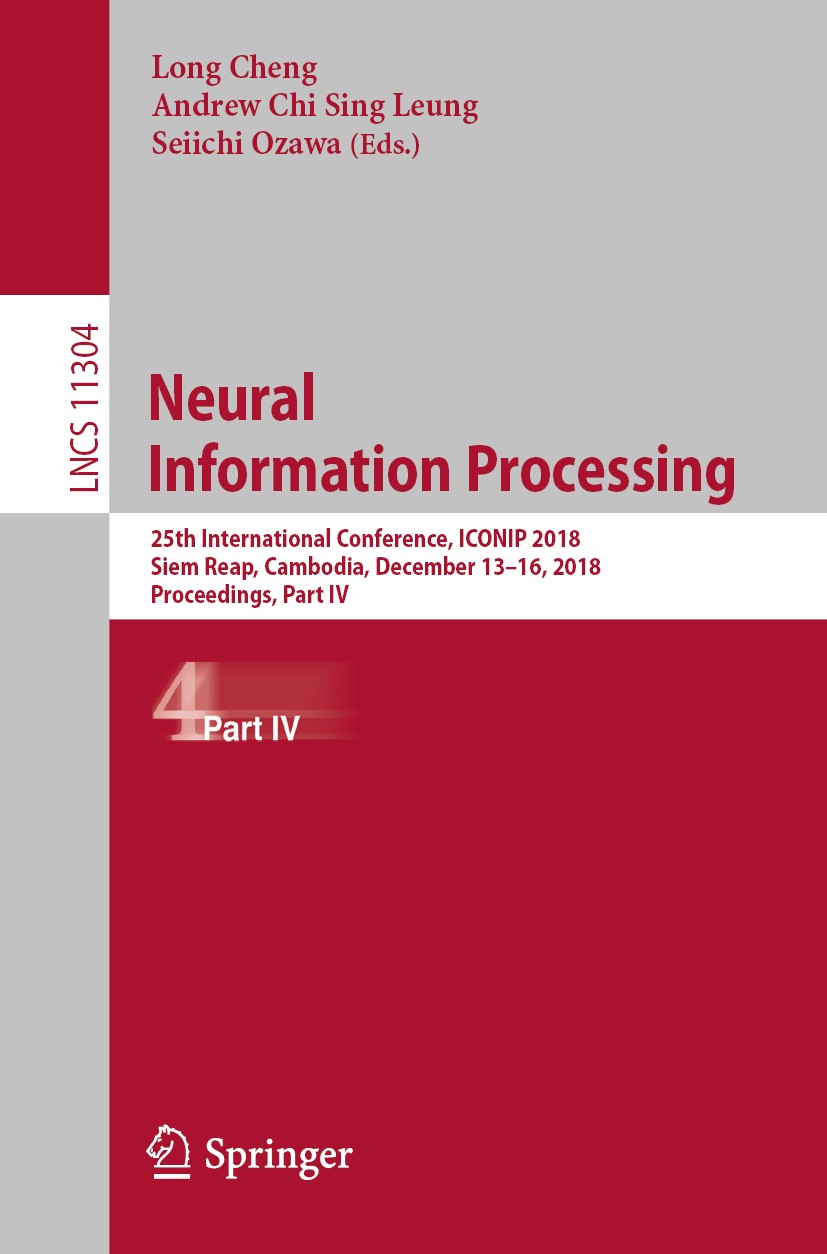 | 描述 | .The seven-volume set of LNCS 11301-11307, constitutes the proceedings of the 25th International Conference on Neural Information Processing, ICONIP 2018, held in Siem Reap, Cambodia, in December 2018..The 401 full papers presented were carefully reviewed and selected from 575 submissions. The papers address the emerging topics of theoretical research, empirical studies, and applications of neural information processing techniques across different domains. The 4th volume, LNCS 11304, is organized in topical sections on feature selection, clustering, classification, and detection. . | 出版日期 | Conference proceedings 2018 | 关键词 | artificial intelligence; biomedical engineering; data mining; deep learning; hci; human-computer interact | 版次 | 1 | doi | https://doi.org/10.1007/978-3-030-04212-7 | isbn_softcover | 978-3-030-04211-0 | isbn_ebook | 978-3-030-04212-7Series ISSN 0302-9743 Series E-ISSN 1611-3349 | issn_series | 0302-9743 | copyright | Springer Nature Switzerland AG 2018 |
The information of publication is updating
|
|