书目名称 | Model Selection and Inference | 副标题 | A Practical Informat | 编辑 | Kenneth P. Burnham,David R. Anderson | 视频video | | 图书封面 | 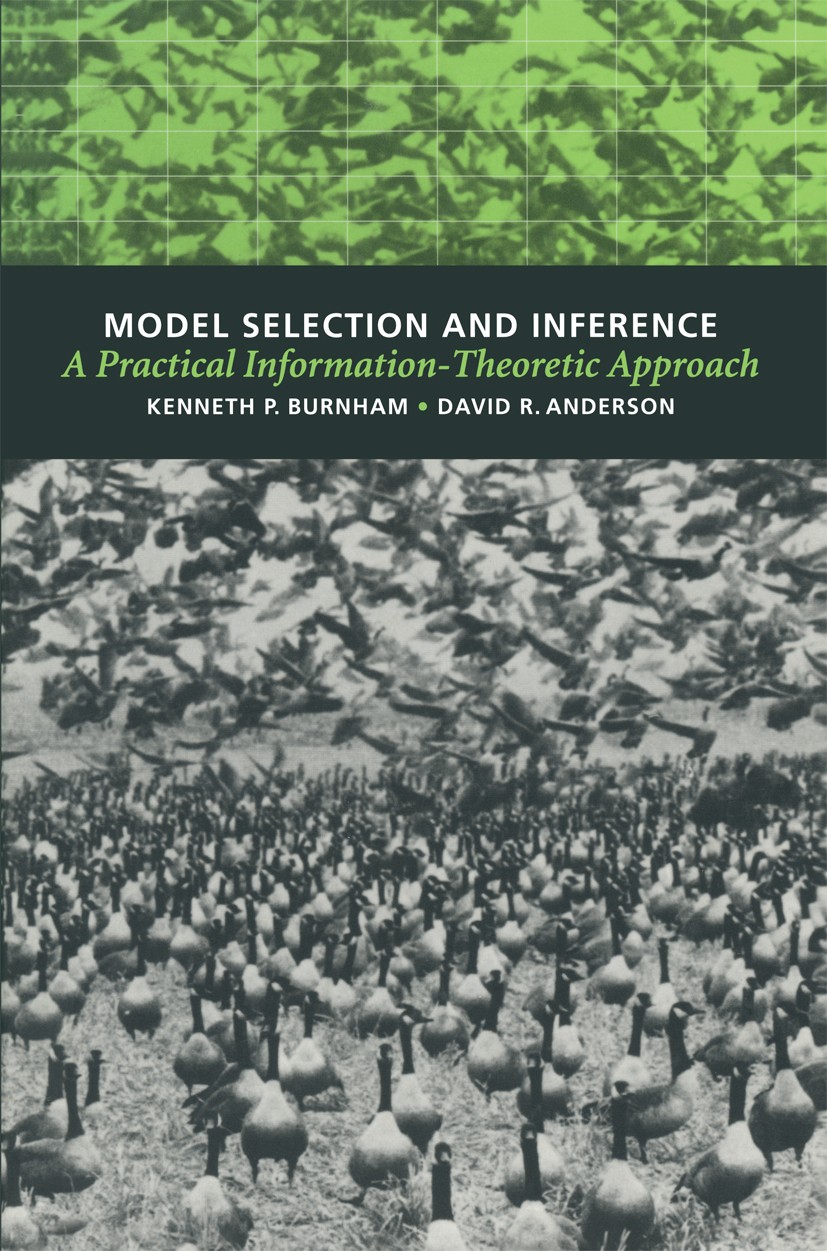 | 描述 | We wrote this book to introduce graduate students and research workers in var ious scientific disciplines to the use of information-theoretic approaches in the analysis of empirical data. In its fully developed form, the information-theoretic approach allows inference based on more than one model (including estimates of unconditional precision); in its initial form, it is useful in selecting a "best" model and ranking the remaining models. We believe that often the critical issue in data analysis is the selection of a good approximating model that best represents the inference supported by the data (an estimated "best approximating model"). In formation theory includes the well-known Kullback-Leibler "distance" between two models (actually, probability distributions), and this represents a fundamental quantity in science. In 1973, Hirotugu Akaike derived an estimator of the (relative) Kullback-Leibler distance based on Fisher‘s maximized log-likelihood. His mea sure, now called Akaike ‘s information criterion (AIC), provided a new paradigm for model selection in the analysis of empirical data. His approach, with a funda mental link to information theory, is relatively simple an | 出版日期 | Book 19981st edition | 关键词 | Inference; Likelihood; Model Selection; data analysis; information theory; optimization; statistics | 版次 | 1 | doi | https://doi.org/10.1007/978-1-4757-2917-7 | isbn_ebook | 978-1-4757-2917-7 | copyright | Springer Science+Business Media New York 1998 |
The information of publication is updating
|
|