书目名称 | Maximum Penalized Likelihood Estimation |
副标题 | Volume I: Density Es |
编辑 | P. P. B. Eggermont,V. N. LaRiccia |
视频video | |
概述 | Reference Book intended for grad students and researchers in statistics, industrial and Engineering mathematics, and operations research.Convexity and convex optimization receive special attention.Inc |
丛书名称 | Springer Series in Statistics |
图书封面 | 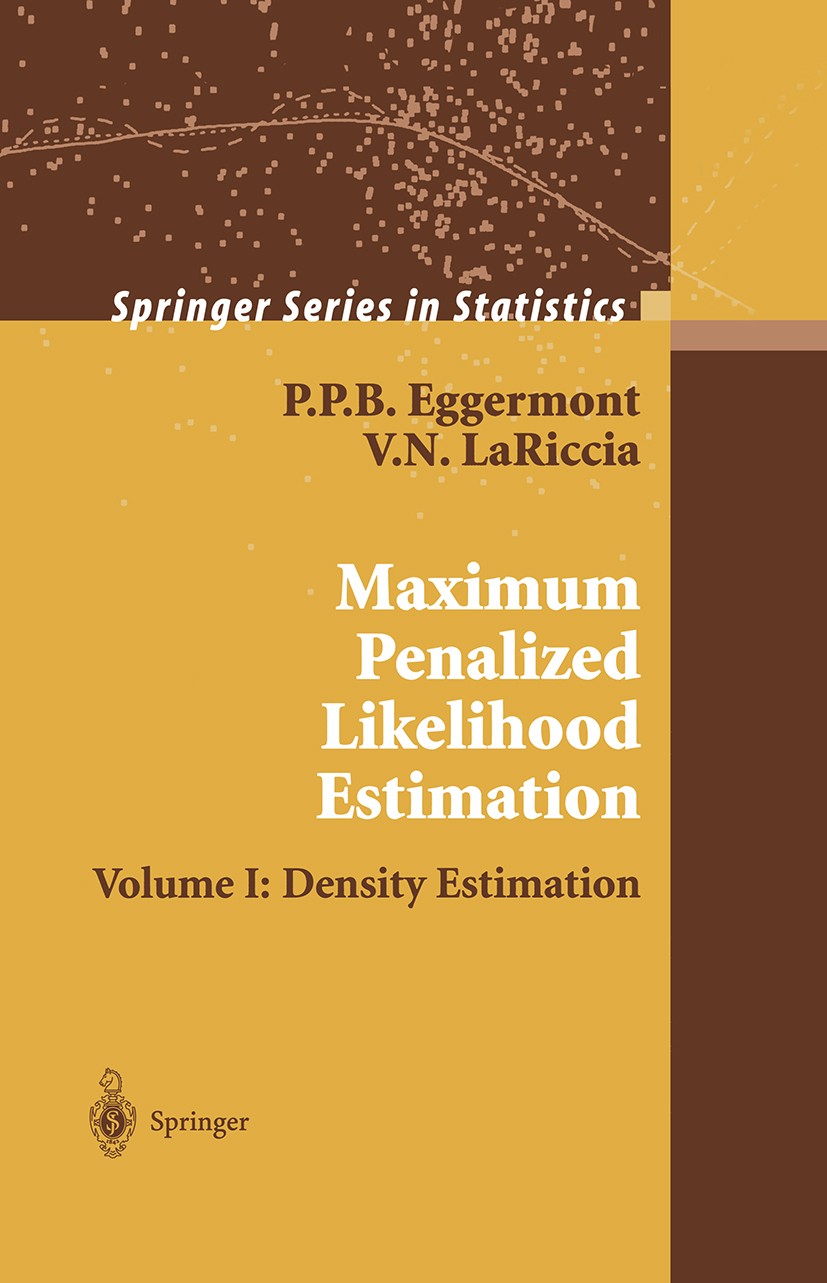 |
描述 | This book is intended for graduate students in statistics and industrial mathematics, as well as researchers and practitioners in the field. We cover both theory and practice of nonparametric estimation. The text is novel in its use of maximum penalized likelihood estimation, and the theory of convex minimization problems (fully developed in the text) to obtain convergence rates. We also use (and develop from an elementary view point) discrete parameter submartingales and exponential inequalities. A substantial effort has been made to discuss computational details, and to include simulation studies and analyses of some classical data sets using fully automatic (data driven) procedures. Some theoretical topics that appear in textbook form for the first time are definitive treatments of I.J. Good‘s roughness penalization, monotone and unimodal density estimation, asymptotic optimality of generalized cross validation for spline smoothing and analogous methods for ill-posed least squares problems, and convergence proofs of EM algorithms for random sampling problems. |
出版日期 | Book 2001 |
关键词 | Density Estimation; Maximum Likelihood; Maximum Penalized Likelihood; nonparametric estimation; convex m |
版次 | 1 |
doi | https://doi.org/10.1007/978-1-0716-1244-6 |
isbn_softcover | 978-1-4419-2928-0 |
isbn_ebook | 978-1-0716-1244-6Series ISSN 0172-7397 Series E-ISSN 2197-568X |
issn_series | 0172-7397 |
copyright | Springer Science+Business Media, LLC, part of Springer Nature 2001 |