书目名称 | Maximum Penalized Likelihood Estimation |
副标题 | Volume II: Regressio |
编辑 | Vincent N. LaRiccia,Paul P.‘Eggermont |
视频video | |
概述 | Fully develops the theory of convex minimization problems to obtain convergence rates.Includes simulation studies and analyses of classical data sets using fully automatic (data driven) procedures.Man |
丛书名称 | Springer Series in Statistics |
图书封面 | 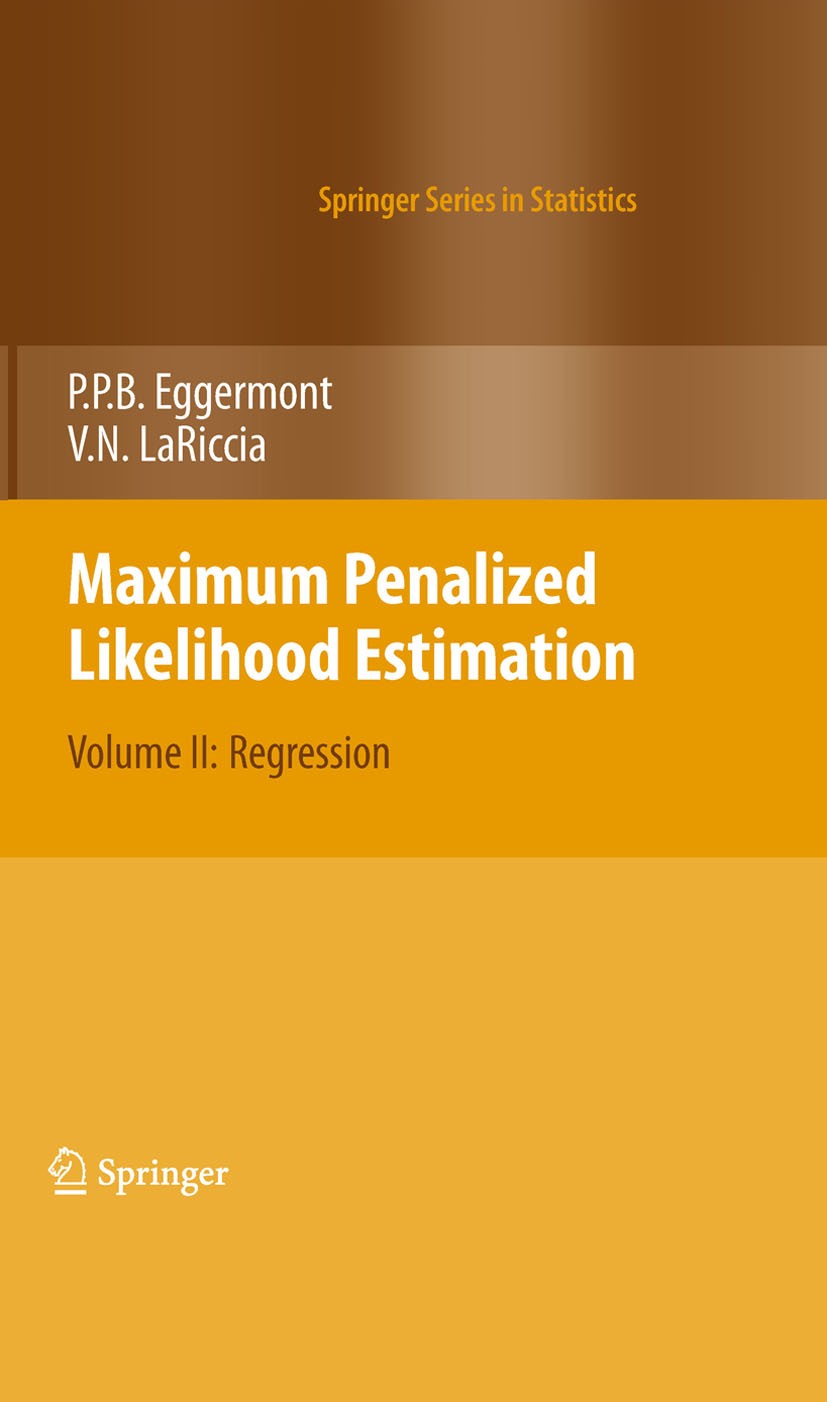 |
描述 | This is the second volume of a text on the theory and practice of maximum penalized likelihood estimation. It is intended for graduate students in s- tistics, operationsresearch, andappliedmathematics, aswellasresearchers and practitioners in the ?eld. The present volume was supposed to have a short chapter on nonparametric regression but was intended to deal mainly with inverse problems. However, the chapter on nonparametric regression kept growing to the point where it is now the only topic covered. Perhaps there will be a Volume III. It might even deal with inverse problems. But for now we are happy to have ?nished Volume II. The emphasis in this volume is on smoothing splines of arbitrary order, but other estimators (kernels, local and global polynomials) pass review as well. We study smoothing splines and local polynomials in the context of reproducing kernel Hilbert spaces. The connection between smoothing splines and reproducing kernels is of course well-known. The new twist is thatlettingtheinnerproductdependonthesmoothingparameteropensup new possibilities: It leads to asymptotically equivalent reproducing kernel estimators (without quali?cations) and thence, via uniform er |
出版日期 | Book 2009 |
关键词 | Confidence bands; Estimator; Kalman filter for smoothing splines; ; Likelihood; Local polynomials; Nonpara |
版次 | 1 |
doi | https://doi.org/10.1007/b12285 |
isbn_softcover | 978-1-4614-1712-5 |
isbn_ebook | 978-0-387-68902-9Series ISSN 0172-7397 Series E-ISSN 2197-568X |
issn_series | 0172-7397 |
copyright | Springer-Verlag New York 2009 |