书目名称 | Mathematical Foundations of Big Data Analytics | 编辑 | Vladimir Shikhman,David Müller | 视频video | | 概述 | Covers all relevant techniques commonly used in Big Data Analytics?.Standardized structure and size of the chapters: motivation, results, case-study, exercises.Recommended and developed for university | 图书封面 | 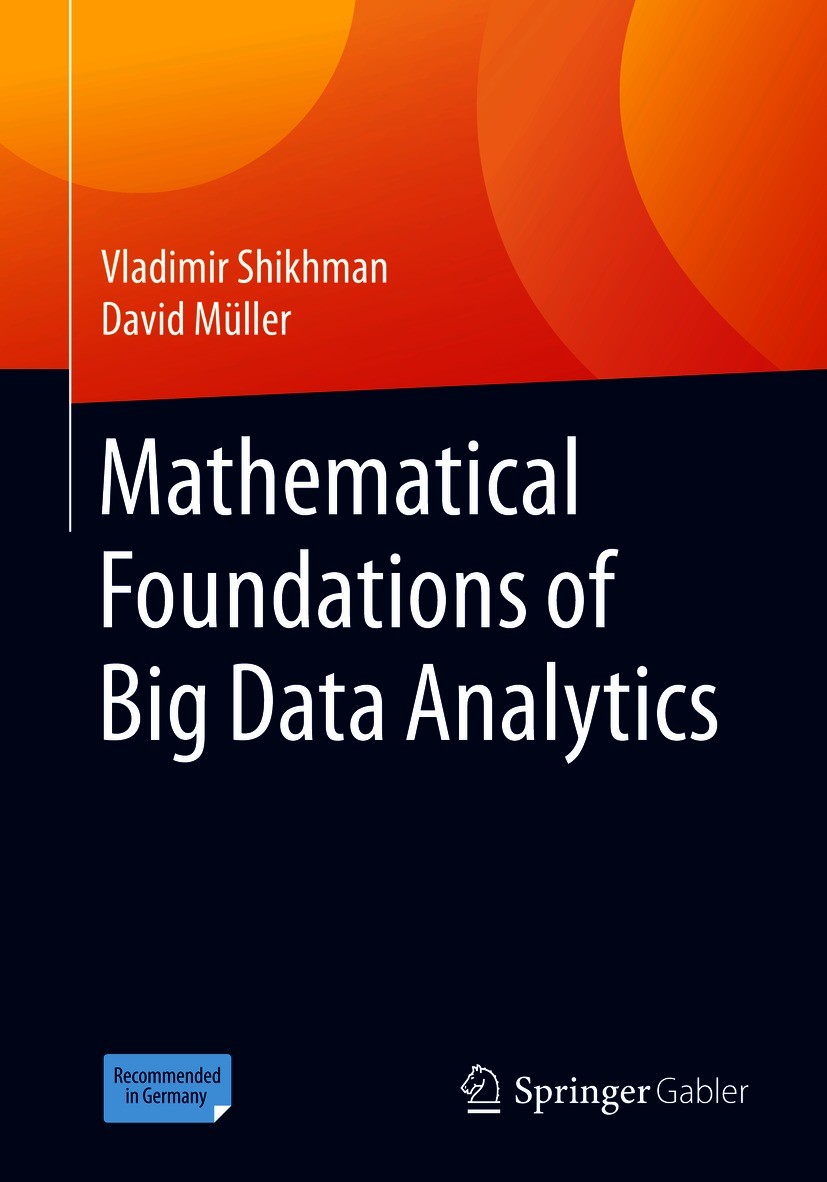 | 描述 | In this textbook, basic mathematical models used in Big Data Analytics are presented and application-oriented references to relevant practical issues are made. Necessary mathematical tools are examined and applied to current problems of data analysis, such as brand loyalty, portfolio selection, credit investigation, quality control, product clustering, asset pricing etc. – mainly in an economic context. In addition, we discuss interdisciplinary applications to biology, linguistics, sociology, electrical engineering, computer science and artificial intelligence. For the models, we make use of a wide range of mathematics – from basic disciplines of numerical linear algebra, statistics and optimization to more specialized game, graph and even complexity theories. By doing so, we cover all relevant techniques commonly used in Big Data Analytics..Each chapter starts with a concrete practical problem whose primary aim is to motivate the study of a particular Big Data Analytics technique. Next, mathematical results follow – including important definitions, auxiliary statements and conclusions arising. Case-studies help to deepen the acquired knowledge by applying it in an interdisciplinar | 出版日期 | Textbook 2021 | 关键词 | Mathematical Models for Big Data Analytics; Analysis of Big Data; Economic Applications of Big Data An | 版次 | 1 | doi | https://doi.org/10.1007/978-3-662-62521-7 | isbn_softcover | 978-3-662-62520-0 | isbn_ebook | 978-3-662-62521-7 | copyright | Springer-Verlag GmbH Germany, part of Springer Nature 2021 |
The information of publication is updating
|
|