书目名称 | Mathematical Foundations for Data Analysis |
编辑 | Jeff M. Phillips |
视频video | |
概述 | Provides accessible, simplified introduction to core mathematical language and concepts.Integrates examples of key concepts through geometric illustrations and Python coding.Addresses topics in locali |
丛书名称 | Springer Series in the Data Sciences |
图书封面 | 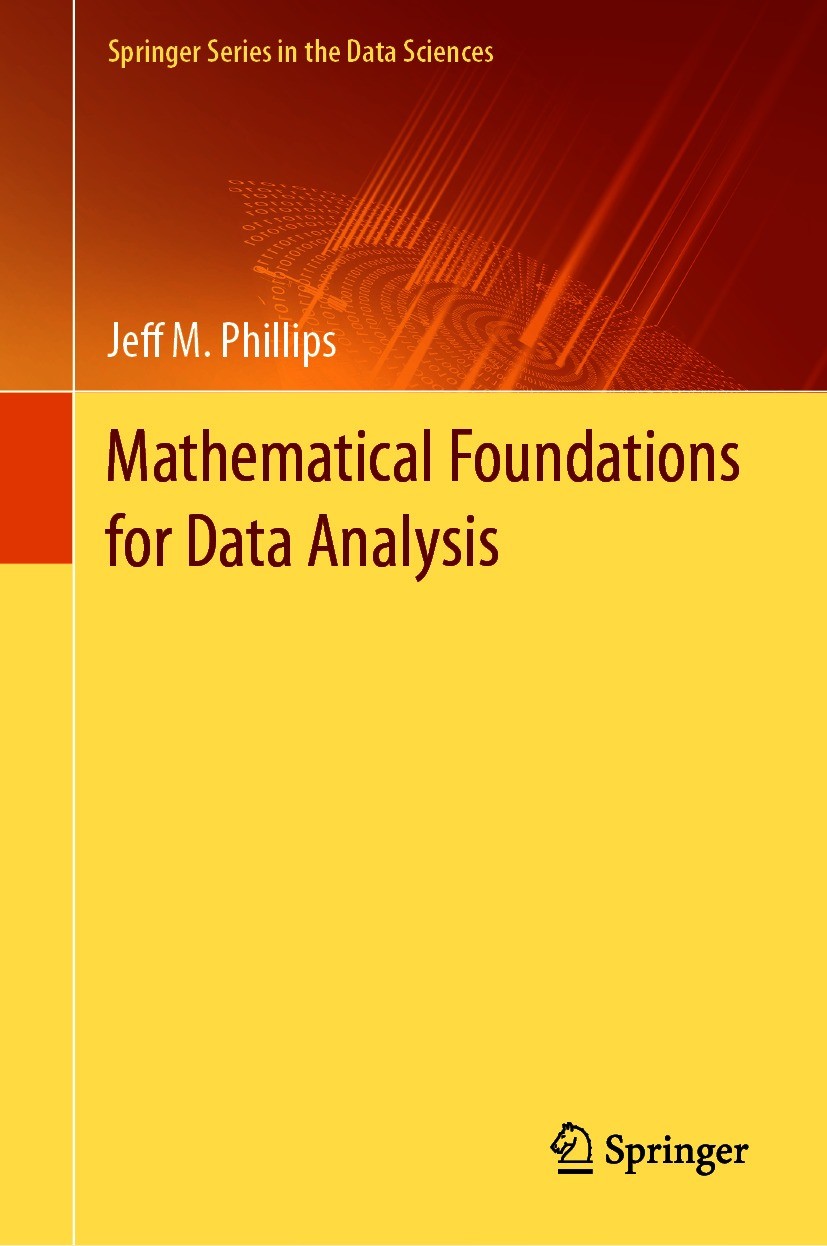 |
描述 | .This textbook, suitable for an early undergraduate up to a graduate course, provides an overview of many basic principles and techniques needed for modern data analysis. In particular, this book was designed and written as preparation for students planning to take rigorous Machine Learning and Data Mining courses. It introduces key conceptual tools necessary for data analysis, including concentration of measure and PAC bounds, cross validation, gradient descent, and principal component analysis. It also surveys basic techniques in supervised (regression and classification) and unsupervised learning (dimensionality reduction and clustering) through an accessible, simplified presentation. Students are recommended to have some background in calculus, probability, and linear algebra. Some familiarity with programming and algorithms is useful to understand advanced topics on computational techniques.. |
出版日期 | Textbook 2021 |
关键词 | Data Analysis; Data Sciences; Data Mining; Machine Learning; Probability; Neural Networks; Geometry of Dat |
版次 | 1 |
doi | https://doi.org/10.1007/978-3-030-62341-8 |
isbn_softcover | 978-3-030-62343-2 |
isbn_ebook | 978-3-030-62341-8Series ISSN 2365-5674 Series E-ISSN 2365-5682 |
issn_series | 2365-5674 |
copyright | Springer Nature Switzerland AG 2021 |