书目名称 | Machine Learning, Optimization, and Data Science | 副标题 | 4th International Co | 编辑 | Giuseppe Nicosia,Panos Pardalos,Vincenzo Sciacca | 视频video | | 丛书名称 | Lecture Notes in Computer Science | 图书封面 | 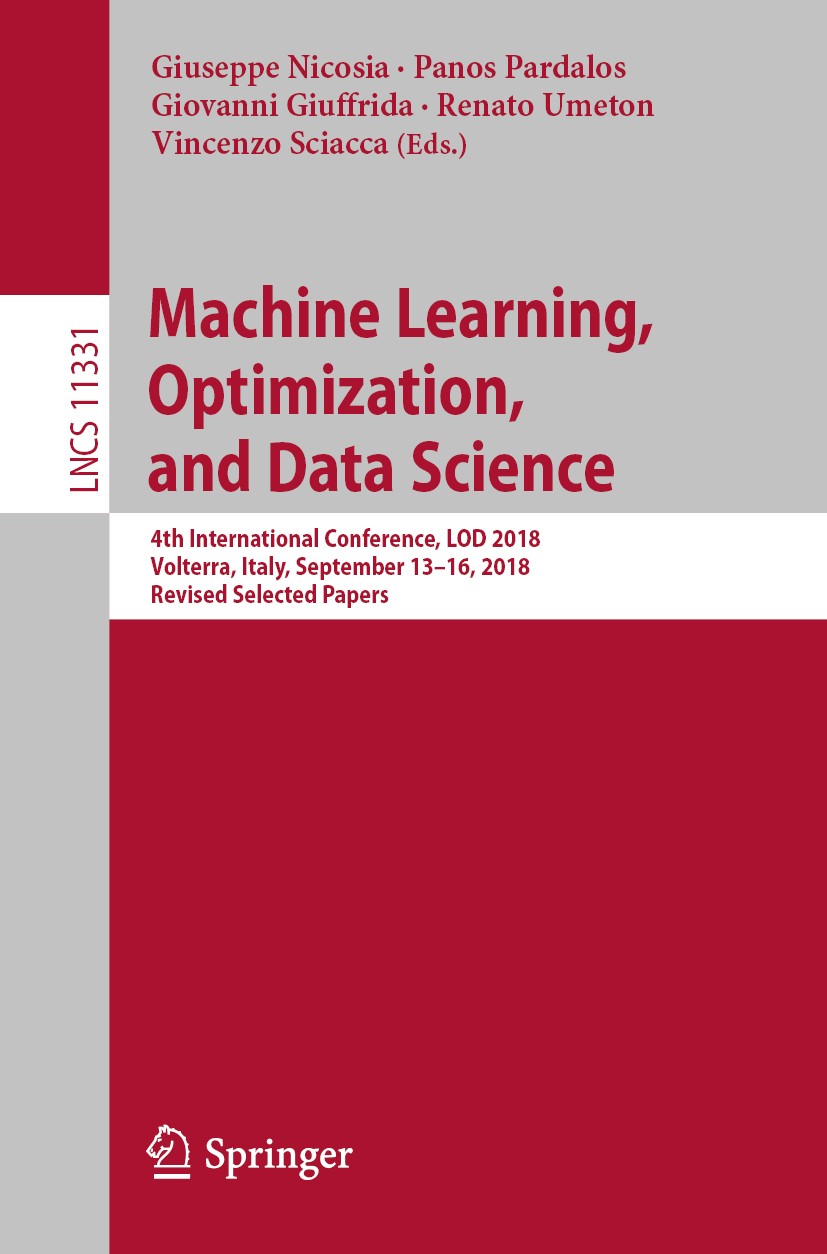 | 描述 | This book constitutes the post-conference proceedings of the 4th International Conference on Machine Learning, Optimization, and Data Science, LOD 2018, held in Volterra, Italy, in September 2018..The 46 full papers presented were carefully reviewed and selected from 126 submissions. The papers cover topics in the field of machine learning, artificial intelligence, reinforcement learning, computational optimization and data science presenting a substantial array of ideas, technologies, algorithms, methods and applications.. | 出版日期 | Conference proceedings 2019 | 关键词 | deep learning; machine learning; reinforcement learning; neural networks; deep reinforcement learning; op | 版次 | 1 | doi | https://doi.org/10.1007/978-3-030-13709-0 | isbn_softcover | 978-3-030-13708-3 | isbn_ebook | 978-3-030-13709-0Series ISSN 0302-9743 Series E-ISSN 1611-3349 | issn_series | 0302-9743 | copyright | Springer Nature Switzerland AG 2019 |
The information of publication is updating
书目名称Machine Learning, Optimization, and Data Science影响因子(影响力) 
书目名称Machine Learning, Optimization, and Data Science影响因子(影响力)学科排名 
书目名称Machine Learning, Optimization, and Data Science网络公开度 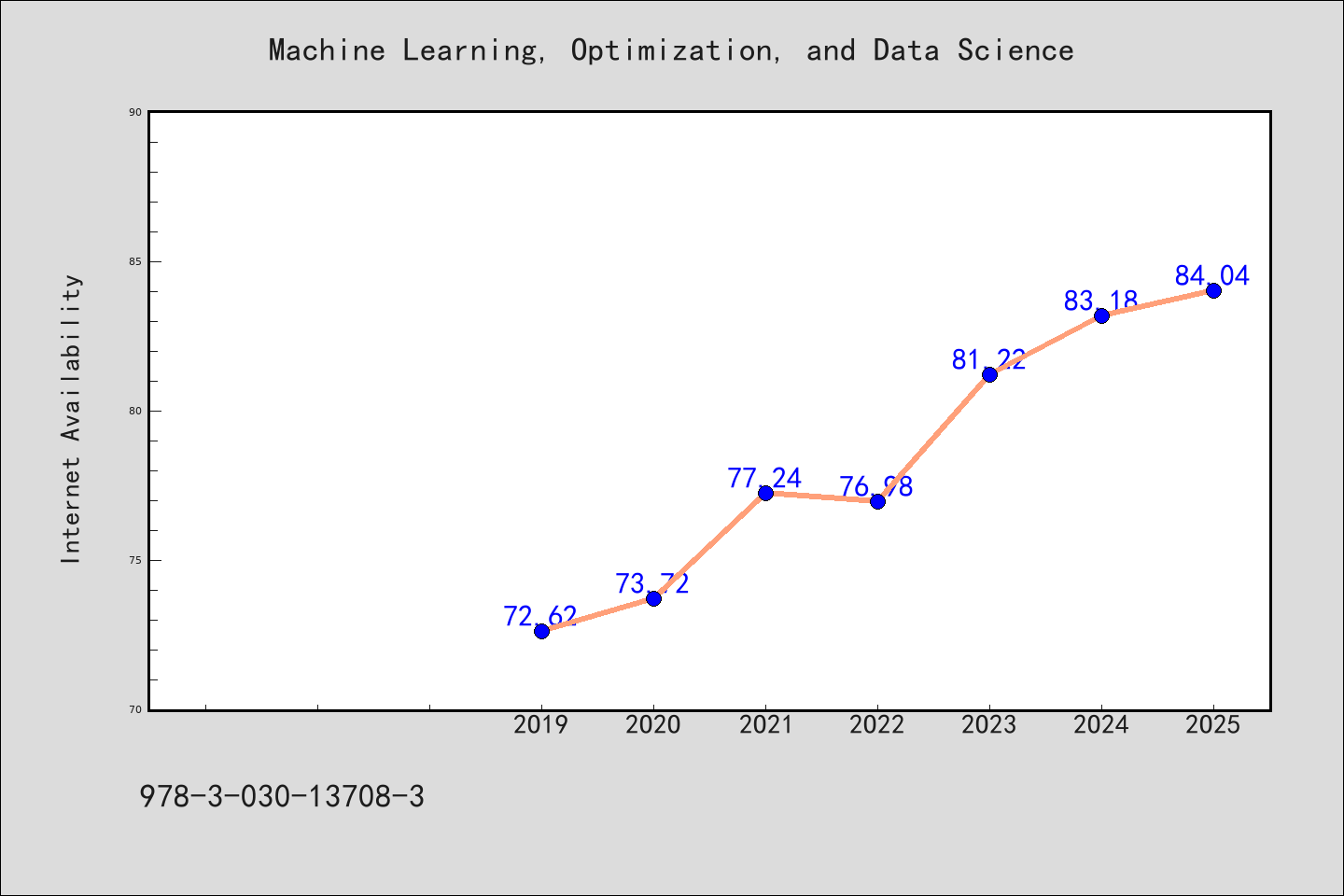
书目名称Machine Learning, Optimization, and Data Science网络公开度学科排名 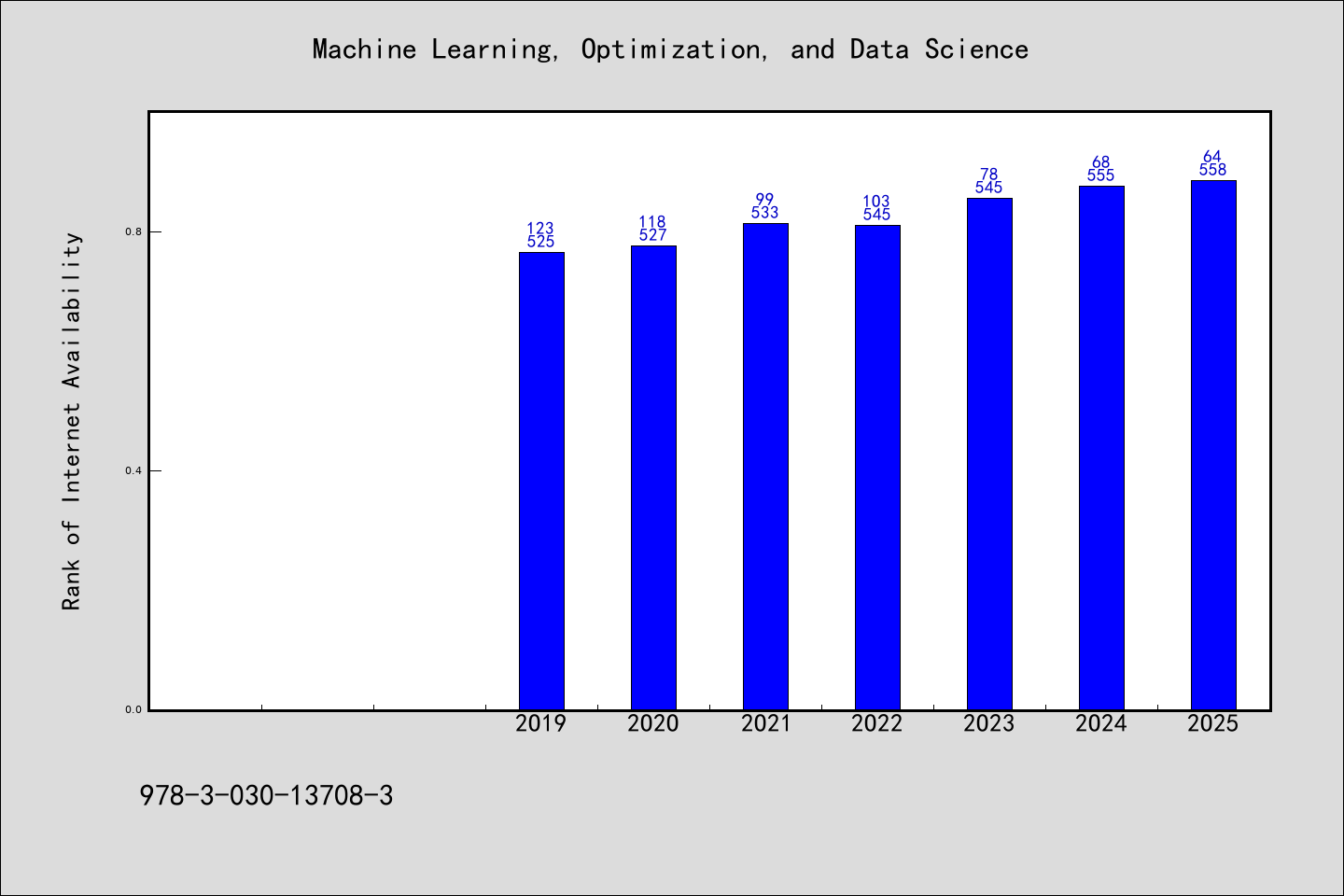
书目名称Machine Learning, Optimization, and Data Science被引频次 
书目名称Machine Learning, Optimization, and Data Science被引频次学科排名 
书目名称Machine Learning, Optimization, and Data Science年度引用 
书目名称Machine Learning, Optimization, and Data Science年度引用学科排名 
书目名称Machine Learning, Optimization, and Data Science读者反馈 
书目名称Machine Learning, Optimization, and Data Science读者反馈学科排名 
|
|
|